Orthogonal Bandit Learning For Portfolio Selection Under Cardinality Constraint
COMPUTATIONAL SCIENCE AND ITS APPLICATIONS - ICCSA 2019, PT III: 19TH INTERNATIONAL CONFERENCE, SAINT PETERSBURG, RUSSIA, JULY 1-4, 2019, PROCEEDINGS, PART III(2019)
摘要
In this paper, we address the portfolio selection problem and solve it by means of a machine learning approach. More precisely, we study portfolio optimization under cardinality constraint, which limits the number of assets in a portfolio. This problem is known to be NP-hard and, consequently, difficult to solve for some settings of the cardinality parameter. In order to solve the problem, we introduce a hybrid approach that combines effectively an existing bandit learning algorithm with a kernel search heuristic. The bandit learning algorithm is used for conducting online portfolio selections and the kernel search manages the cardinality constraint. In order to investigate the performance of our algorithm, we carried out computational experiments on real-world market data sets. According to the numerical results, we observe that, despite the presence of cardinality constraint, our hybrid algorithm shows good performance in solving the test instances in reasonable computation time and, in many cases, the algorithm provides portfolios with higher cumulative wealth than those obtained through the existing bandit learning algorithm, which is applied on classical portfolio selection problem.
更多查看译文
关键词
Portfolio selection problem, Machine learning, Multi-armed bandit, Bandit learning, Cardinality constraint, Kernel search
AI 理解论文
溯源树
样例
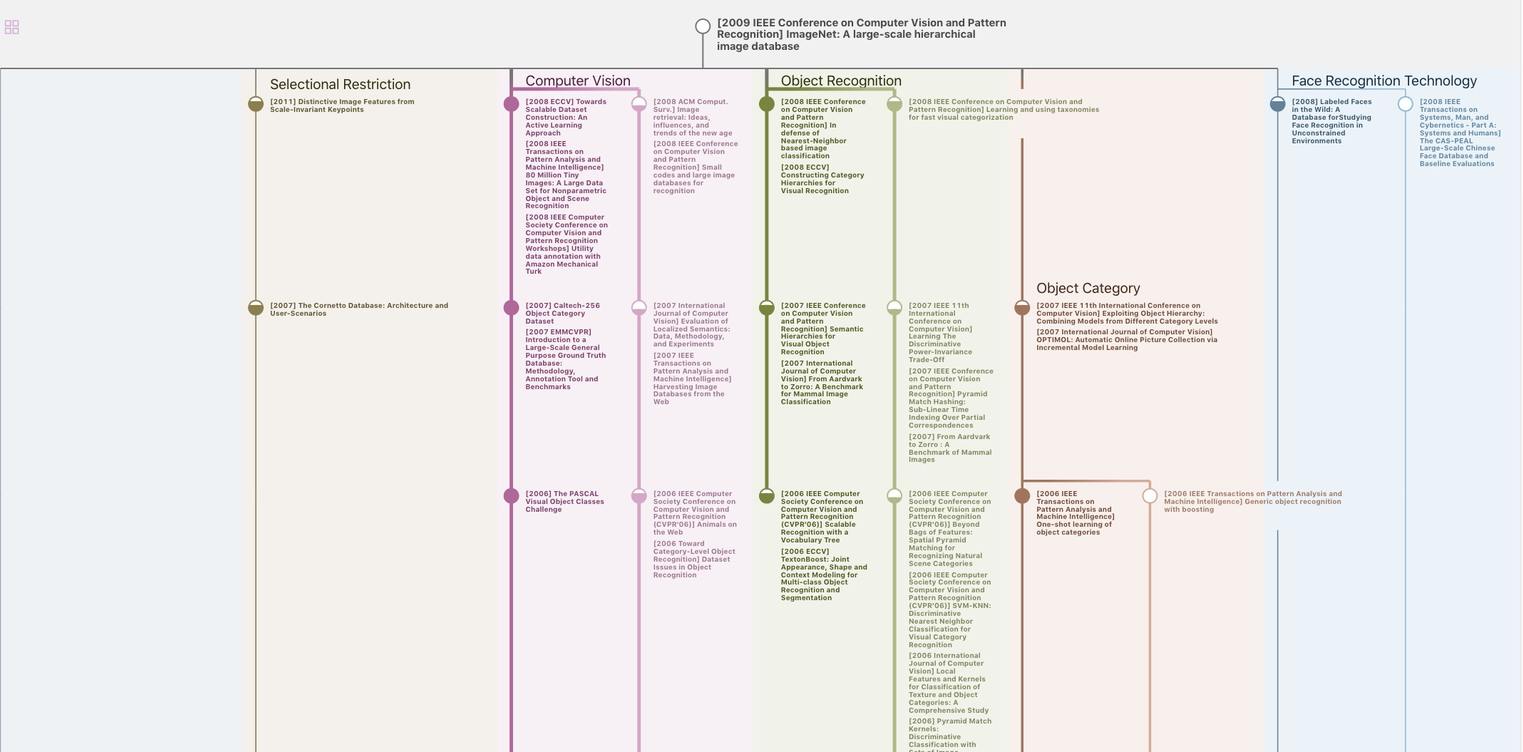
生成溯源树,研究论文发展脉络
Chat Paper
正在生成论文摘要