Encoding Syntactic Dependency and Topical Information for Social Emotion Classification
Proceedings of the 42nd International ACM SIGIR Conference on Research and Development in Information Retrieval(2019)
摘要
Social emotion classification is to estimate the distribution of readers' emotion evoked by an article. In this paper, we design a new neural network model by encoding sentence syntactic dependency and document topical information into the document representation. We first use a dependency embedded recursive neural network to learn syntactic features for each sentence, and then use a gated recurrent unit to transform the sentences' vectors into a document vector. We also use a multi-layer perceptron to encode the topical information of a document into a topic vector. Finally, a gate layer is used to compose the document representation from the gated summation of the document vector and the topic vector. Experiment results on two public datasets indicate that our proposed model outperforms the state-of-the-art methods in terms of better average Pearson correlation coefficient and MicroF1 performance.
更多查看译文
关键词
dependency embedding, recursive neural network, social emotion classification, topic model
AI 理解论文
溯源树
样例
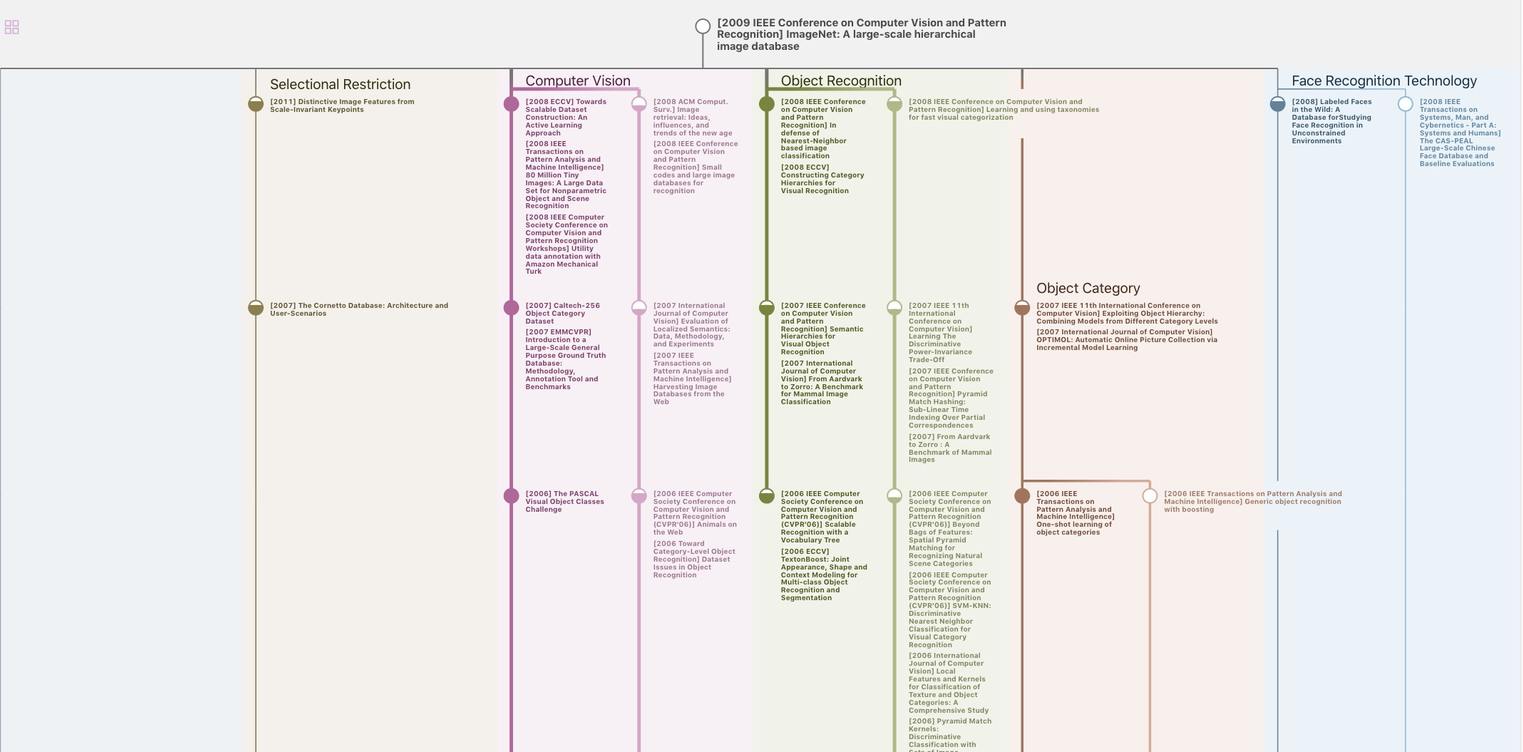
生成溯源树,研究论文发展脉络
Chat Paper
正在生成论文摘要