The Effect Of Evidence Transfer On Latent Feature Relevance For Clustering
INFORMATICS-BASEL(2019)
摘要
Evidence transfer for clustering is a deep learning method that manipulates the latent representations of an autoencoder according to external categorical evidence with the effect of improving a clustering outcome. Evidence transfer's application on clustering is designed to be robust when introduced with a low quality of evidence, while increasing the effectiveness of the clustering accuracy during relevant corresponding evidence. We interpret the effects of evidence transfer on the latent representation of an autoencoder by comparing our method to the information bottleneck method. Information bottleneck is an optimisation problem of finding the best tradeoff between maximising the mutual information of data representations and a task outcome while at the same time being effective in compressing the original data source. We posit that the evidence transfer method has essentially the same objective regarding the latent representations produced by an autoencoder. We verify our hypothesis using information theoretic metrics from feature selection in order to perform an empirical analysis over the information that is carried through the bottleneck of the latent space. We use the relevance metric to compare the overall mutual information between the latent representations and the ground truth labels before and after their incremental manipulation, as well as, to study the effects of evidence transfer regarding the significance of each latent feature.
更多查看译文
关键词
deep neural networks, evidence transfer, relevance, feature selection, information bottleneck, latent features, deep learning
AI 理解论文
溯源树
样例
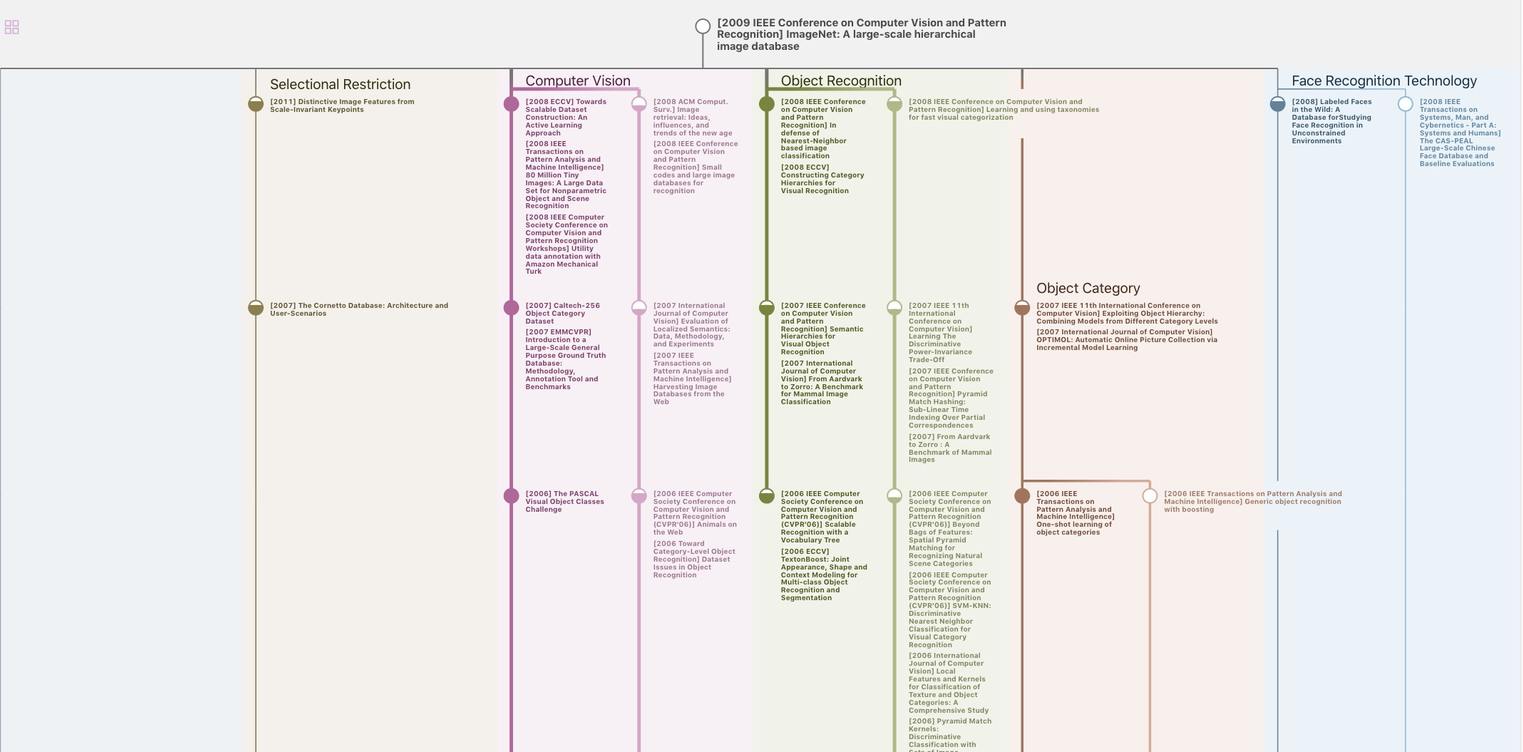
生成溯源树,研究论文发展脉络
Chat Paper
正在生成论文摘要