Epithelial Segmentation From In Situ Hybridisation Histological Samples Using A Deep Central Attention Learning Approach
2019 IEEE 16TH INTERNATIONAL SYMPOSIUM ON BIOMEDICAL IMAGING (ISBI 2019)(2019)
摘要
The assessment of pathological samples by molecular techniques, such as in situ hybridization (ISH) and immunohistochemistry (IHC), has revolutionised modern Histopathology. Most often it is important to detect ISH/IHC reaction products in certain cells or tissue types. For instance, detection of human papilloma virus (HPV) in oropharyngeal cancer samples by ISH products is difficult and remains a tedious and time consuming task for experts. Here we introduce a proposed framework to segment epithelial regions in oropharyngeal tissue images with ISH staining. First, we use colour deconvolution to obtain a counterstain channel and generate input patches based on superpixels and their neighbouring areas. Then, a novel deep attention residual network is applied to identify the epithelial regions to produce an epithelium segmentation mask. In the experimental results, comparing the proposed network with other state-of-the-art deep learning approaches, our network provides a better performance than region-based and pixel-based segmentations.
更多查看译文
关键词
Oropharyngeal Cancer, Tumor Segmentation, Deep Learning, In Situ Hybridisation, Histology
AI 理解论文
溯源树
样例
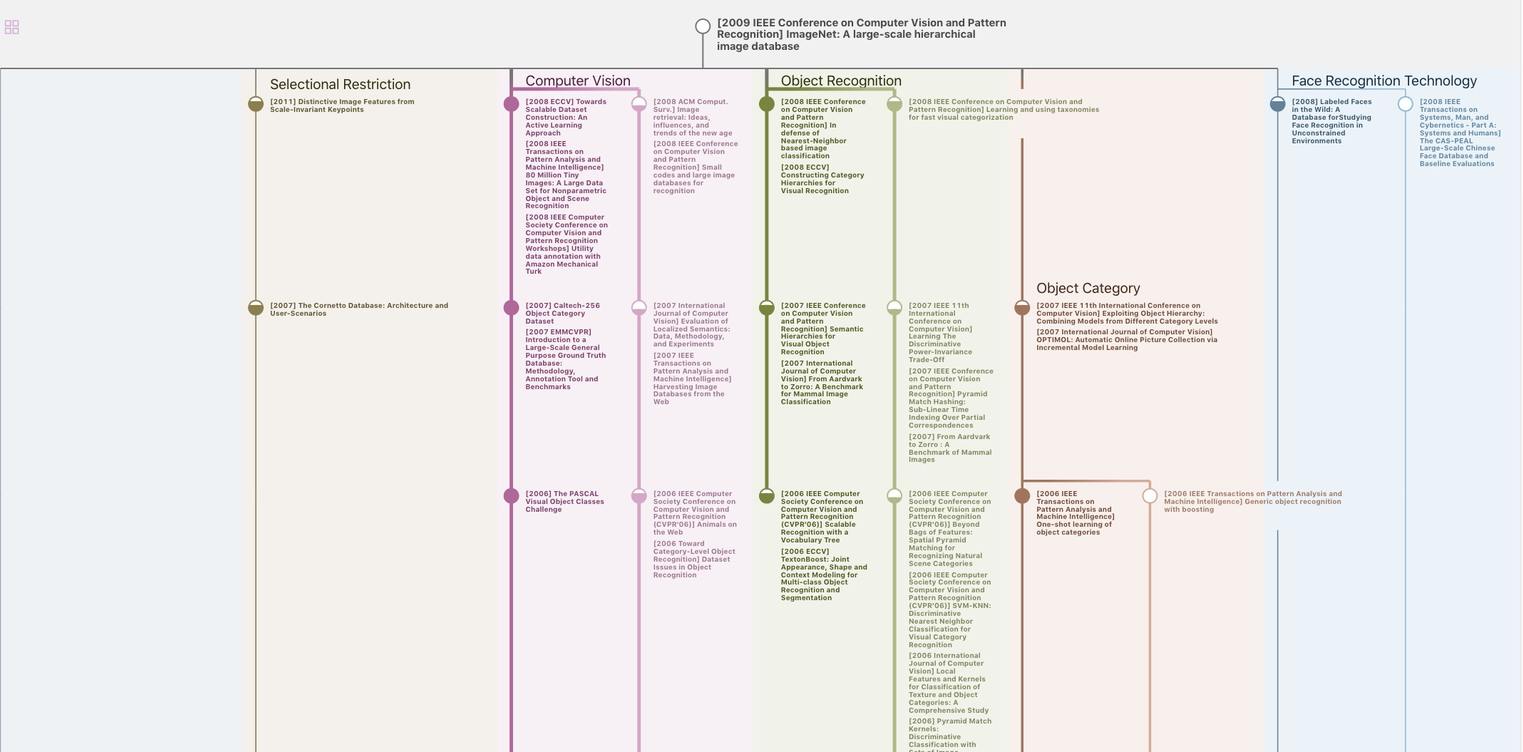
生成溯源树,研究论文发展脉络
Chat Paper
正在生成论文摘要