Segmentation And Quantification Of Cellular Load In Pulmonary Endomicroscopic Images Using Convolutional Neural Networks
2019 IEEE 16TH INTERNATIONAL SYMPOSIUM ON BIOMEDICAL IMAGING (ISBI 2019)(2019)
摘要
Fibre Bundle Endomicroscopy (FBE mu) is an emerging tool that facilitates the real-time structural and functional (via fluorescent dyes) imaging of the distal lung, providing valuable in vivo, in situ indicators across a range pathological or physiological processes. This paper proposes a novel approach for localising and quantifying abnormalities in distal lung, such as increased cellular load, through semantic image segmentation. Two Convolutional Neural Network (CNN) architectures have been tested, (i) U-Net, a purpose specific network for biomedical image applications, and (ii) ENct, a network optimised for fast inference. The results indicate that semantic segmentation of cells as well as quantification of cellular load is viable, with U-Net consistently outperforming ENet, obtaining a pixel accuracy of 0.842 and a correlation (r) with the corresponding manual cellular load estimation of 0.866.*
更多查看译文
关键词
Endomicroscopy, convolutional neural networks, semantic segmentation, cellular load, lung
AI 理解论文
溯源树
样例
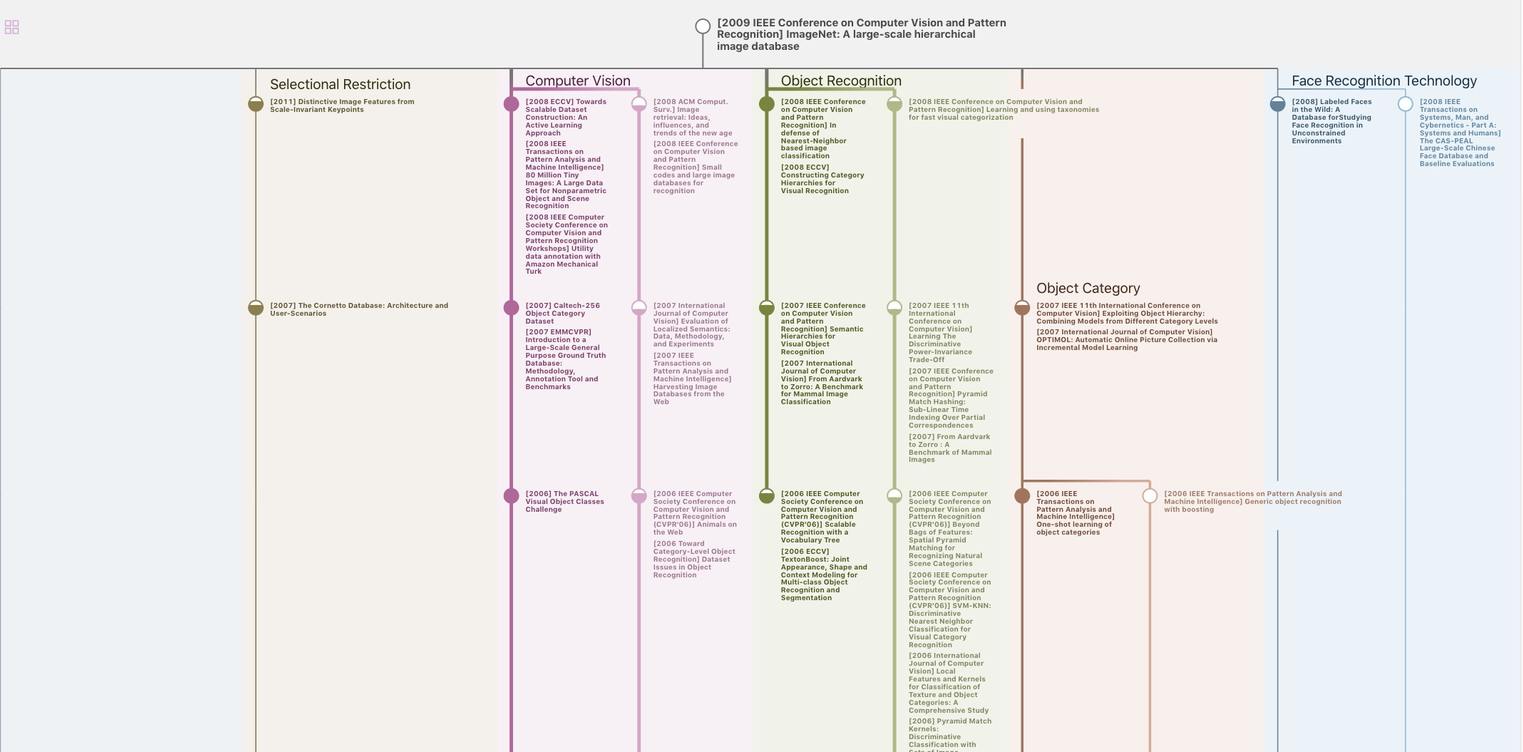
生成溯源树,研究论文发展脉络
Chat Paper
正在生成论文摘要