Motion Planning Networks: Bridging the Gap Between Learning-Based and Classical Motion Planners
IEEE Transactions on Robotics(2021)
摘要
This article describes motion planning networks (MPNet), a computationally efficient, learning-based neural planner for solving motion planning problems.MPNet uses neural networks to learn general near-optimal heuristics for path planning in seen and unseen environments. It takes environment information such as raw point cloud from depth sensors, as well as a robot's initial and desired goal configurations and recursively calls itself to bidirectionally generate connectable paths. In addition to finding directly connectable and near-optimal paths in a single pass, we show that worst-case theoretical guarantees can be proven if we merge this neural network strategy with classical sample-based planners in a hybrid approach while still retaining significant computational and optimality improvements. To train the MPNet models, we present an active continual learning approach that enables MPNet to learn from streaming data and actively ask for expert demonstrations when needed, drastically reducing data for training. We validate MPNet against gold-standard and state-of-the-art planning methods in a variety of problems from two-dimensional to seven-dimensional robot configuration spaces in challenging and cluttered environments, with results showing significant and consistently stronger performance metrics, and motivating neural planning in general as a modern strategy for solving motion planning problems efficiently.
更多查看译文
关键词
Deep learning in robotics and automation,learning and adaptive systems,learning from demonstration,motion and path planning
AI 理解论文
溯源树
样例
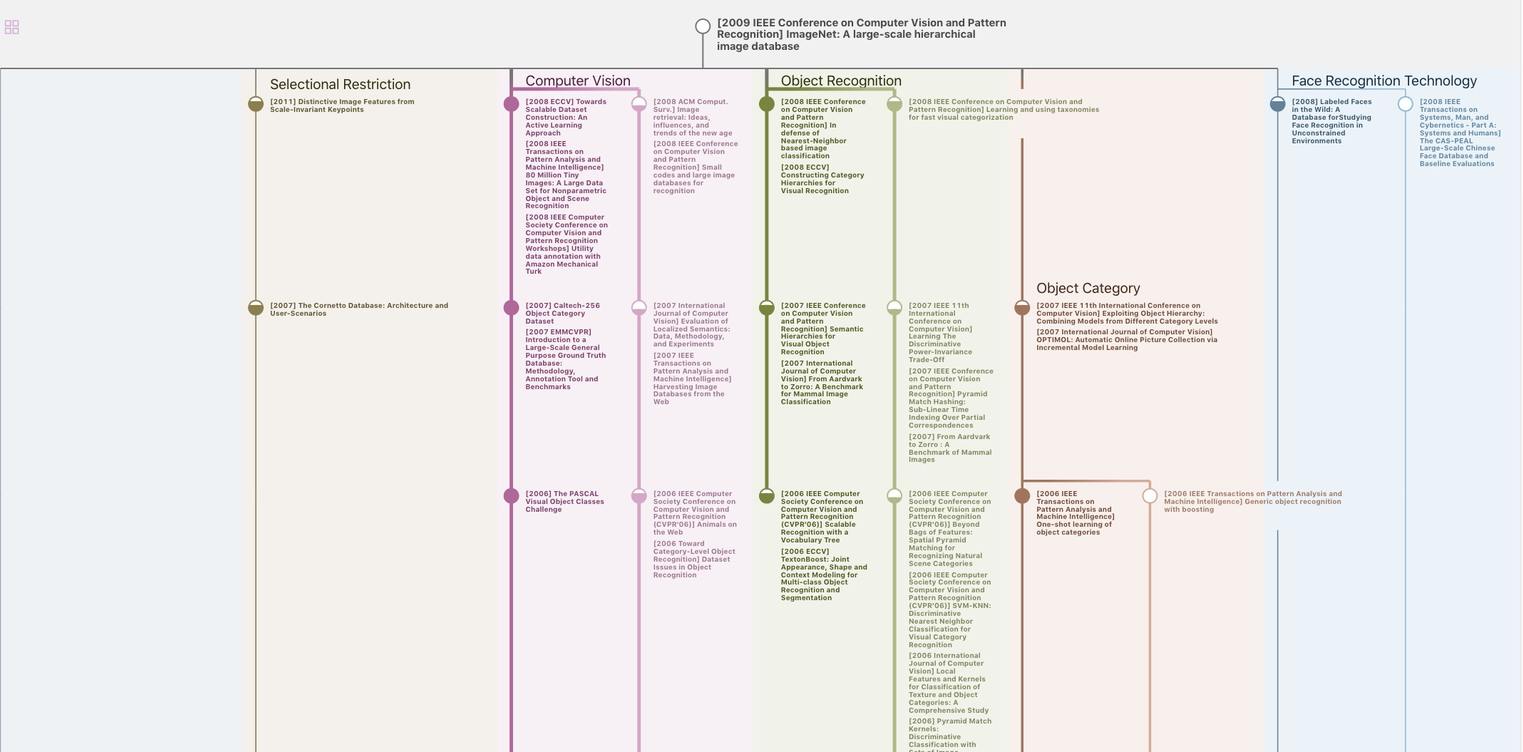
生成溯源树,研究论文发展脉络
Chat Paper
正在生成论文摘要