Weakly Supervised Semantic Segmentation Based on Superpixel Sampling Clustering Networks
Proceedings of the 2nd International Conference on Computer Science and Software Engineering(2019)
摘要
Semantic segmentation models based on deep neural networks depend highly on a large number of pixel-level annotations, while pixel-level annotations require a lot of manpower and resources. Therefore, we propose a weakly supervised image semantic segmentation algorithm based on deep convolutional neural network. This method only the quantity image-level annotations to perform image semantic segmentation. In this paper, the non-conductible superpixel sampling algorithm SLIC is transformed into derivable by relaxing restrictions, added to the deep convolutional neural network to achieve end-to-end training, and the center of the superpixel is dynamically obtained step by step. The saliency map with semantic information is used as the seed, and the seed is used as the cluster center. Then the superpixel block is used as the basic unit when seed grows, and the dynamically generated superpixel module is clustered using the K-Means algorithm. Set the number of categories for image segmentation to the number of clusters of the K-Means algorithm. Until a steady state is reached. Finally, the segmentation results optimized by CRF algorithm to generates fined graind contour. The algorithm is tested in the open challenge data set PASCAL VOC 2012, which is superior to the existing weakly supervised semantic segmentation algorithm, the convergence speed is significantly improved, and the segmentation result is more refined.
更多查看译文
关键词
K-Means, Semantic segmentation, Superpixel, Weakely supervision
AI 理解论文
溯源树
样例
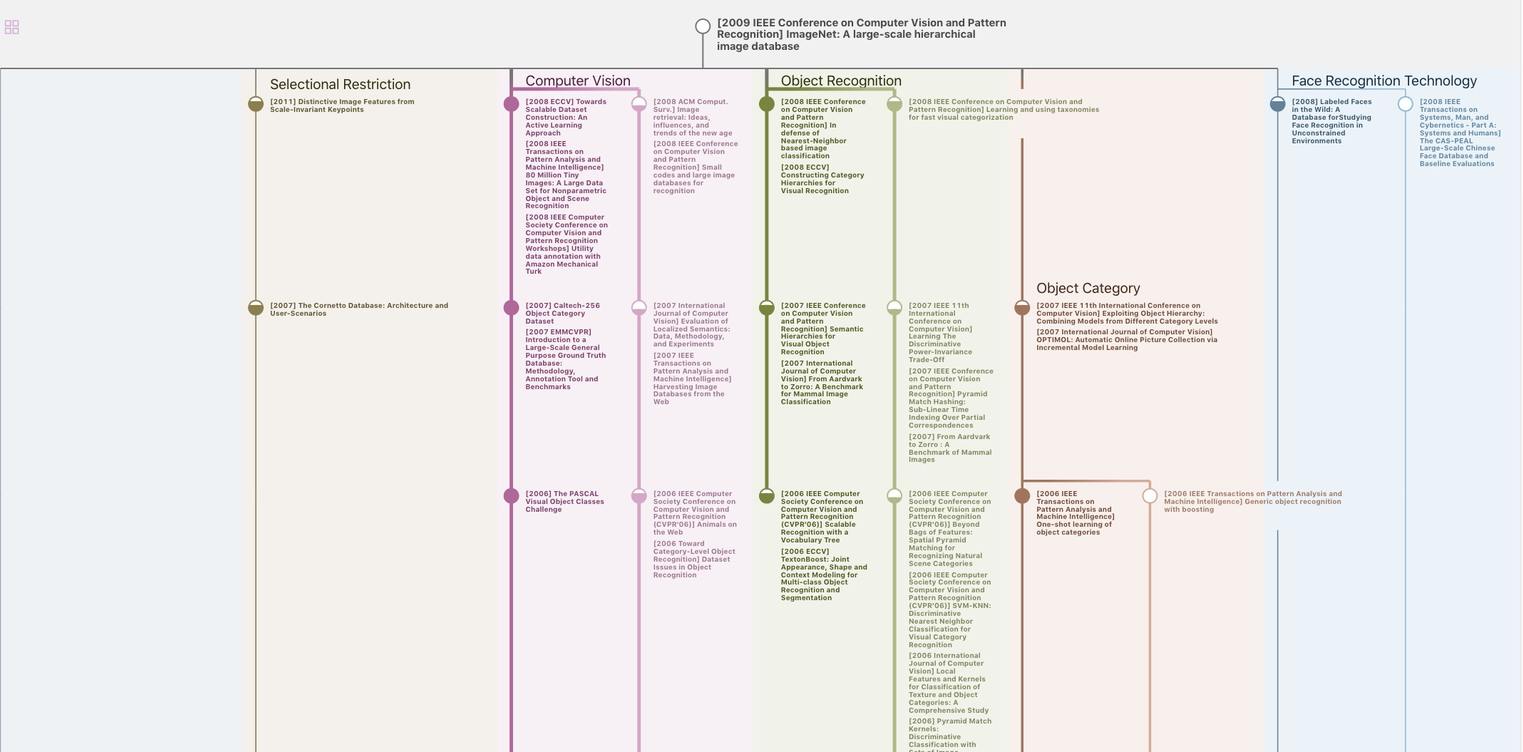
生成溯源树,研究论文发展脉络
Chat Paper
正在生成论文摘要