Cost-Efficient Task Scheduling for Geo-distributed Data Analytics.
SpaCCS Workshops(2019)
摘要
Geo-distributed data processing is affected by many factors, some countries or regions prohibit the transmission of original user data abroad. Therefore, it is necessary to adopt a non-centralized processing method for these data, but at the same time, many problems will arise. Firstly, it is unavoidable to transfer job’s intermediate data across regions, which will result in data transmission cost. Secondly, the WAN bandwidth is often much smaller than the bandwidth within clusters, which makes it easier to become the bottleneck of geo-distributed job. In addition, because the idle computing resources in the cluster may change with time, it will also cause some difficulties in task scheduling. Therefore, this paper considers the problem of task scheduling for big data jobs on geo-distributed data, considering the budget constraints on intermediate data trans-regional transmission, and without moving the original data. we design a budget-constrained task scheduling strategy CETS. Through the experimental analysis of different scenarios, the effectiveness of the proposed algorithm strategy is verified.
更多查看译文
关键词
Big data, Cloud computing, Cost efficient, Geo-distributed data processing, Task scheduling
AI 理解论文
溯源树
样例
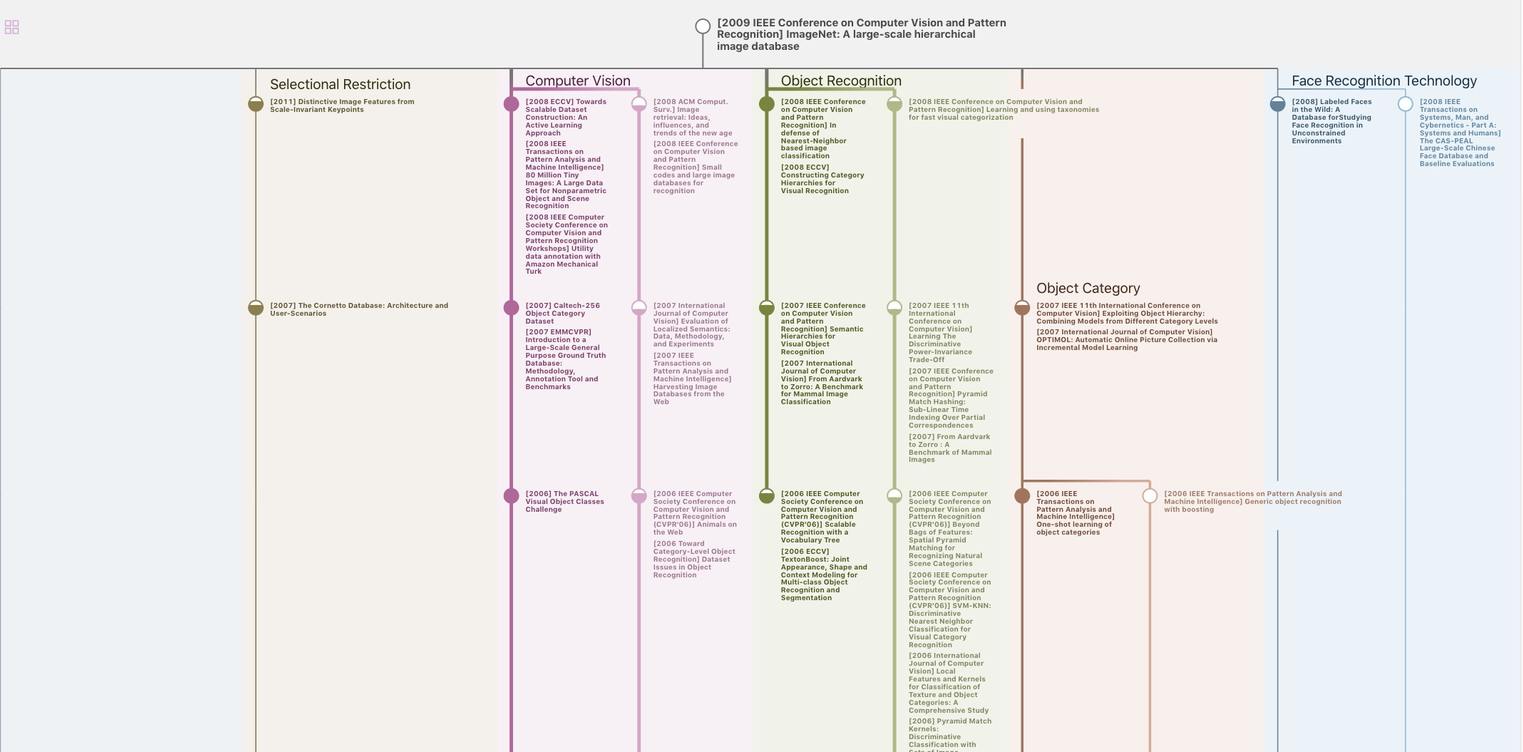
生成溯源树,研究论文发展脉络
Chat Paper
正在生成论文摘要