Privacy-Preserving Classification with Secret Vector Machines
CIKM '20: The 29th ACM International Conference on Information and Knowledge Management Virtual Event Ireland October, 2020(2020)
摘要
Today, large amounts of valuable data are distributed among millions of user-held devices, such as personal computers, phones, or Internet-of-things devices. Many companies collect such data with the goal of using it for training machine learning models allowing them to improve their services. User-held data is, however, often sensitive, and collecting it is problematic in terms of privacy. We address this issue by proposing a novel way of training a supervised classifier in a distributed setting akin to the recently proposed federated learning paradigm, but under the stricter privacy requirement that the server that trains the model is assumed to be untrusted and potentially malicious. We thus preserve user privacy by design, rather than by trust. In particular, our framework, called secret vector machine (SecVM), provides an algorithm for training linear support vector machines (SVM) in a setting in which data-holding clients communicate with an untrusted server by exchanging messages designed to not reveal any personally identifiable information. We evaluate our model in two ways. First, in an offline evaluation, we train SecVM to predict user gender from tweets, showing that we can preserve user privacy without sacrificing classification performance. Second, we implement SecVM's distributed framework for the Cliqz web browser and deploy it for predicting user gender in a large-scale online evaluation with thousands of clients, outperforming baselines by a large margin and thus showcasing that SecVM is suitable for production environments.
更多查看译文
AI 理解论文
溯源树
样例
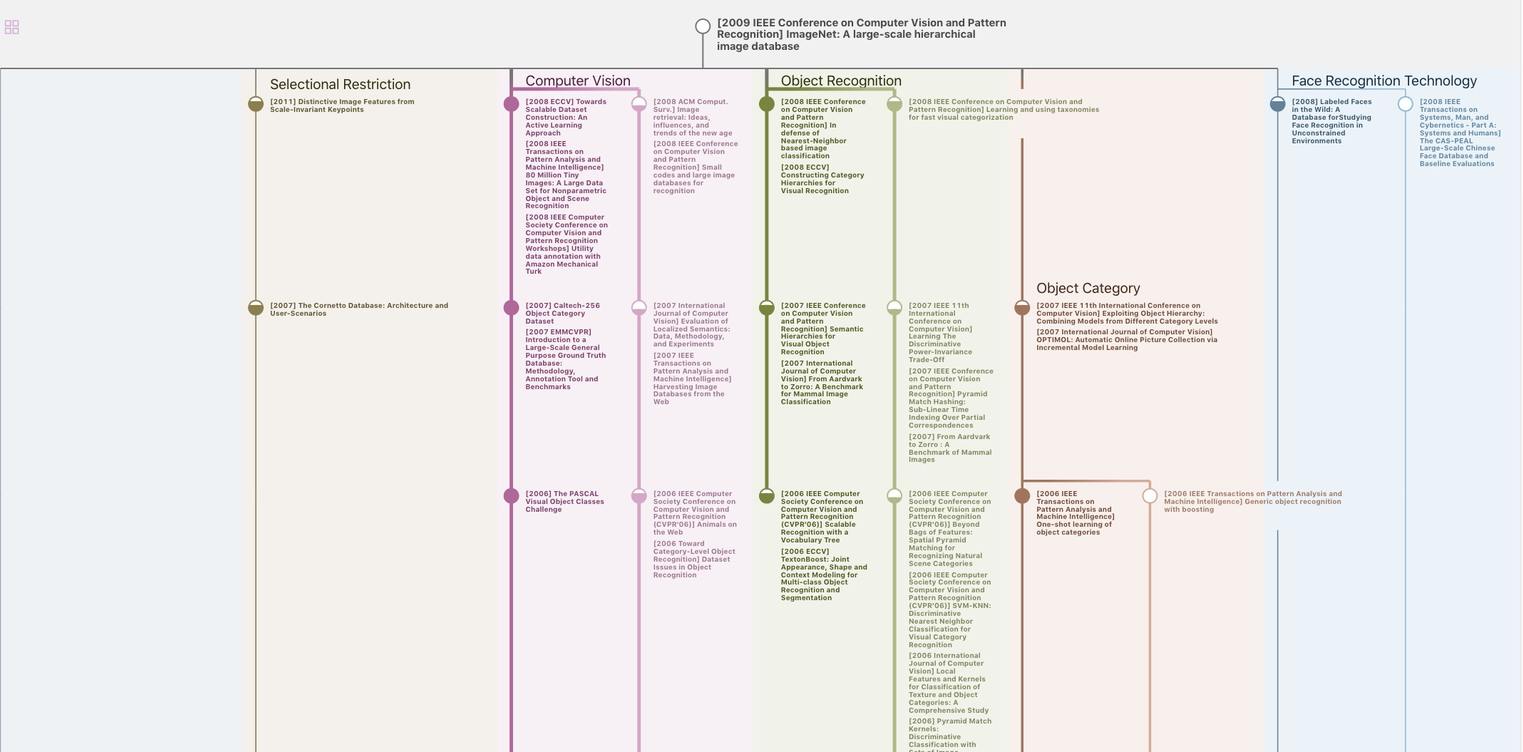
生成溯源树,研究论文发展脉络
Chat Paper
正在生成论文摘要