Reusing the Past Difference Vectors in Differential Evolution—A Simple But Significant Improvement
IEEE Transactions on Cybernetics(2020)
摘要
Differential evolution (DE) has established itself as a simple but efficient population-based, nonconvex optimization algorithm for continuous search spaces. Unlike the conventional real-coded genetic algorithms (GAs) and evolution strategies (ESs), DE uses a mandatory self-referential mutation for its population members, each of which are perturbed with the scaled difference(s) of the individuals from the current generation (iteration). These difference vectors determine the direction of the search moves for the individuals. However, unlike the better individuals, they are not retained in the elitist evolution cycle of DE. In this paper, we show that by archiving the most promising difference vectors from past generations and then by reusing them for generating offspring in the subsequent generations, we can strikingly improve the performance of DE. This strategy can be integrated with any classical or advanced DE variant with no serious overhead in time or space complexity. We demonstrate that when combined with the DE-based winners of the IEEE Congress on Evolutionary Computation (CEC) 2013, 2014, and 2017 competitions on real parameter optimization, the simple reuse strategy leads to a statistically significant performance improvement in the majority of test cases. We further showcase the efficacy of our proposal on a practical optimization problem concerning the design of circular antenna arrays with a prespecified radiation pattern.
更多查看译文
关键词
Benchmarking,circular antenna array design,continuous optimization,difference vectors,differential evolution (DE),mutation
AI 理解论文
溯源树
样例
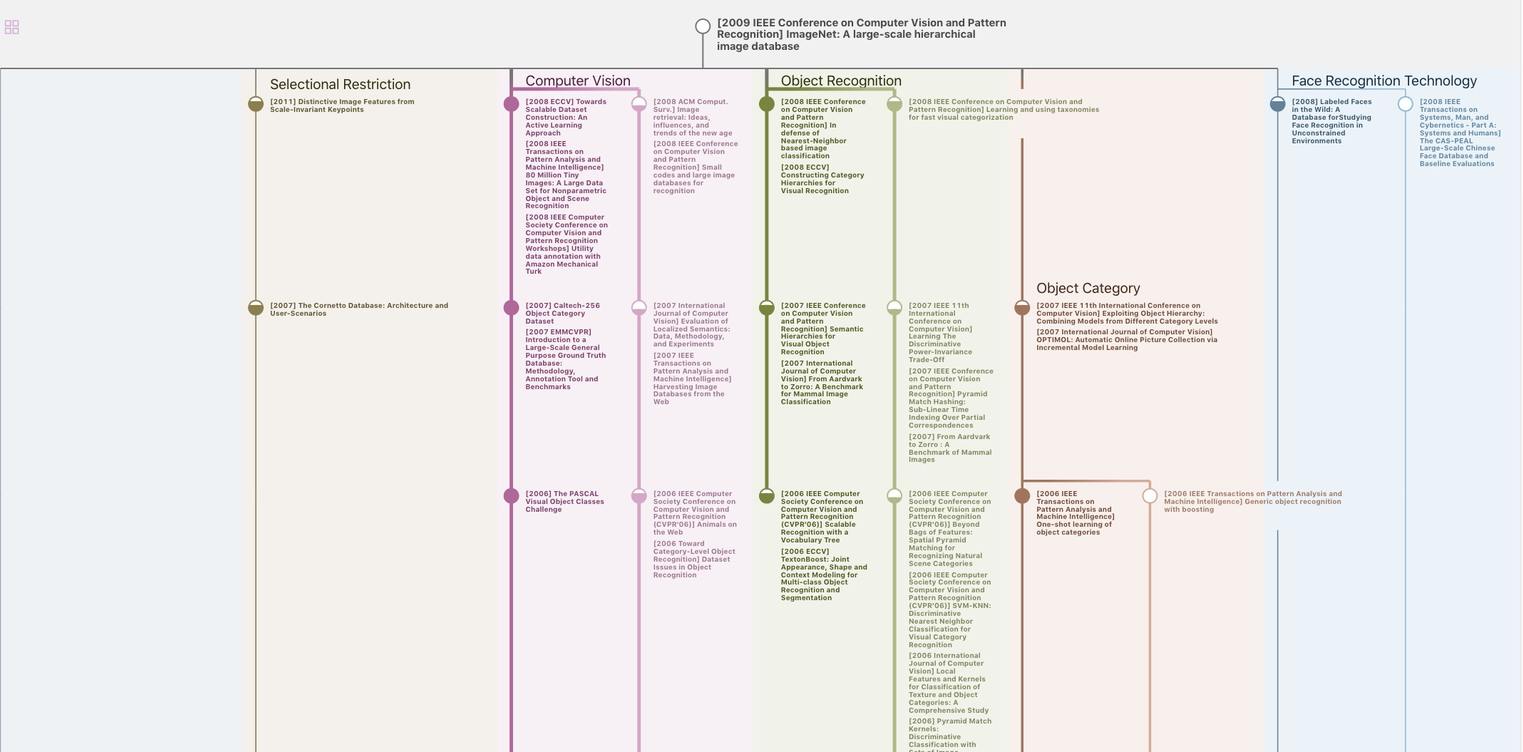
生成溯源树,研究论文发展脉络
Chat Paper
正在生成论文摘要