Hyperspectral-based Estimation of Leaf Nitrogen Content in Corn Using Optimal Selection of Multiple Spectral Variables.
SENSORS(2019)
摘要
Accurate and dynamic monitoring of crop nitrogen status is the basis of scientific decisions regarding fertilization. In this study, we compared and analyzed three types of spectral variables: Sensitive spectral bands, the position of spectral features, and typical hyperspectral vegetation indices. First, the Savitzky-Golay technique was used to smooth the original spectrum, following which three types of spectral parameters describing crop spectral characteristics were extracted. Next, the successive projections algorithm (SPA) was adopted to screen out the sensitive variable set from each type of parameters. Finally, partial least squares (PLS) regression and random forest (RF) algorithms were used to comprehensively compare and analyze the performance of different types of spectral variables for estimating corn leaf nitrogen content (LNC). The results show that the integrated variable set composed of the optimal ones screened by SPA from three types of variables had the best performance for LNC estimation by the validation data set, with the values of R-2, root means square error (RMSE), and normalized root mean square error (NRMSE) of 0.77, 0.31, and 17.1%, and 0.55, 0.43, and 23.9% from PLS and RF, respectively. It indicates that the PLS model with optimally multitype spectral variables can provide better fits and be a more effective tool for evaluating corn LNC.
更多查看译文
关键词
hyperspectral,leaf nitrogen content (LNC),successive projections algorithm (SPA),partial least squares (PLS) model,random forest (RF) model
AI 理解论文
溯源树
样例
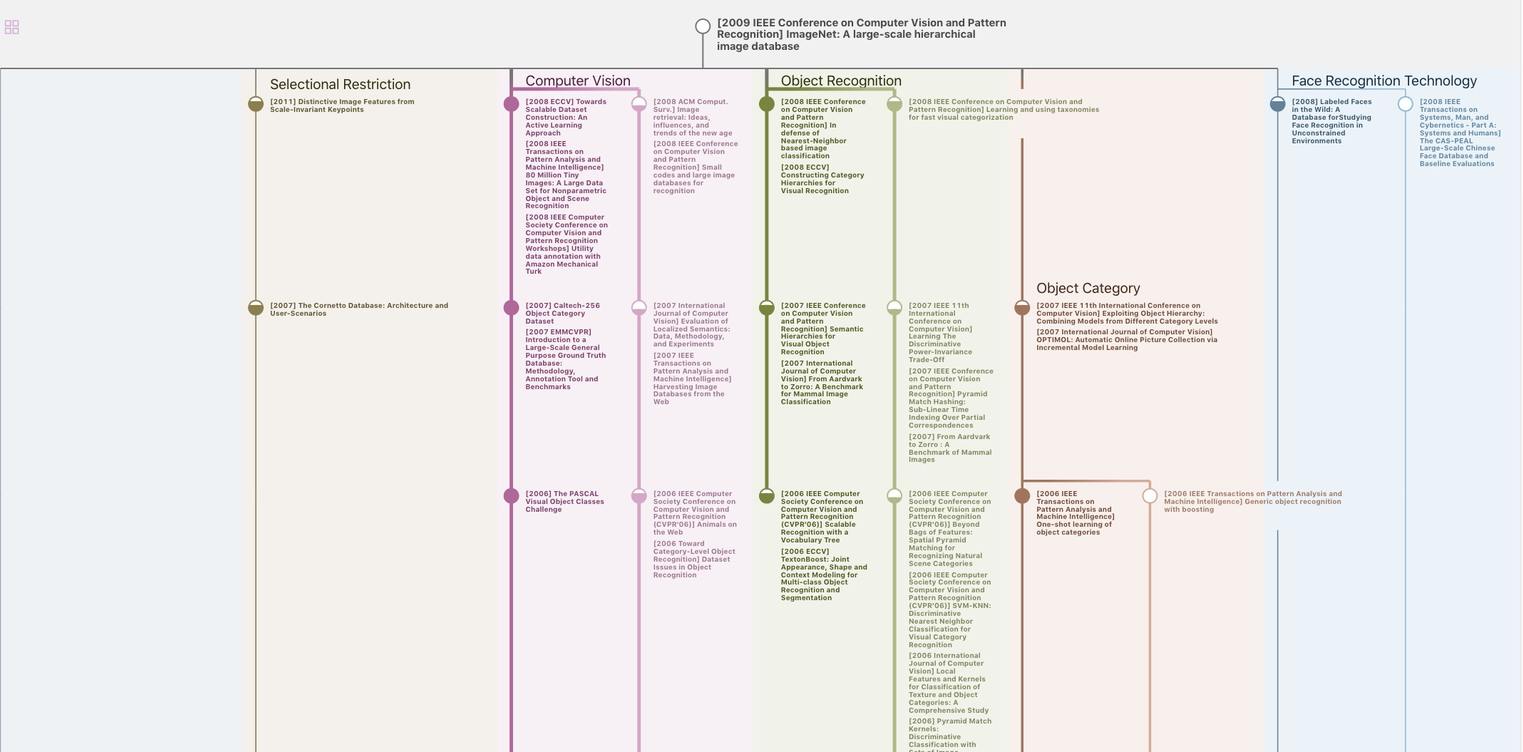
生成溯源树,研究论文发展脉络
Chat Paper
正在生成论文摘要