Iterated Deep Reinforcement Learning in Games: History-Aware Training for Improved Stability
Proceedings of the 2019 ACM Conference on Economics and Computation(2019)
摘要
Deep reinforcement learning (RL) is a powerful method for generating policies in complex environments, and recent breakthroughs in game-playing have leveraged deep RL as part of an iterative multiagent search process. We build on such developments and present an approach that learns progressively better mixed strategies in complex dynamic games of imperfect information, through iterated use of empirical game-theoretic analysis (EGTA) with deep RL policies. We apply the approach to a challenging cybersecurity game defined over attack graphs. Iterating deep RL with EGTA to convergence over dozens of rounds, we generate mixed strategies far stronger than earlier published heuristic strategies for this game. We further refine the strategy-exploration process, by fine-tuning in a training environment that includes out-of-equilibrium but recently seen opponents. Experiments suggest this history-aware approach yields strategies with lower regret at each stage of training.
更多查看译文
关键词
attack graphs, deep reinforcement learning, double oracle, multi-agent reinforcement learning, security games
AI 理解论文
溯源树
样例
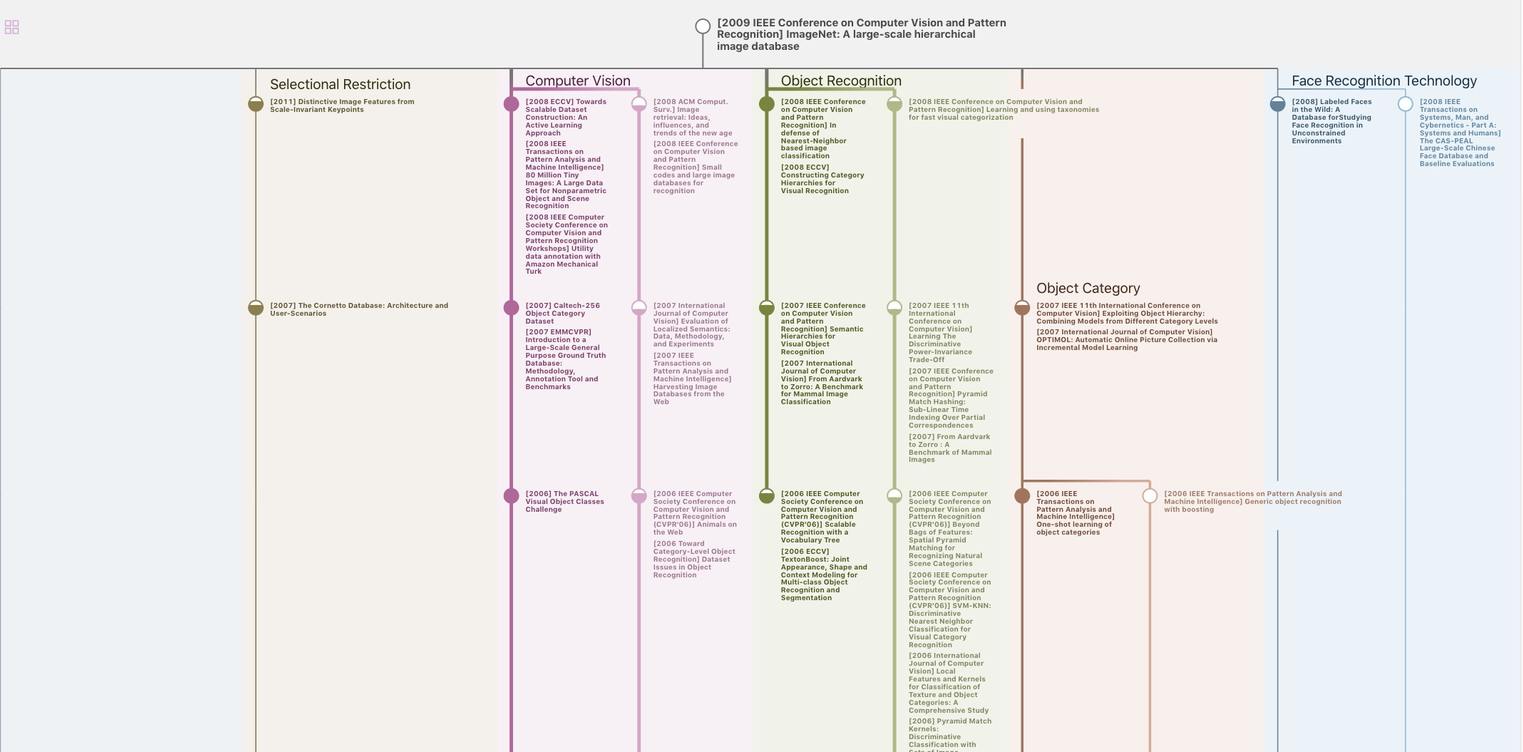
生成溯源树,研究论文发展脉络
Chat Paper
正在生成论文摘要