A Supervised Filter Feature Selection Method For Mixed Data Based On The Spectral Gap Score
PATTERN RECOGNITION, MCPR 2019(2019)
摘要
Feature Selection for supervised classification plays a fundamental role in pattern recognition, data mining, machine learning, among other areas. However, most supervised feature selection methods have been designed for handling exclusively numerical or non-numerical data; so, in practical problems of fields such as medicine, economy, business, and social sciences, where the objects of study are usually described by both numerical and non-numerical features (mixed data), traditional supervised feature selection methods cannot be directly applied. This paper introduces a supervised filter feature selection method for mixed data based on the spectral gap score and a new kernel capable of modeling the data structure in a supervised way. To demonstrate the effectiveness of the proposed method, we conducted some experiments on public real-world mixed datasets.
更多查看译文
关键词
Supervised feature selection, Spectral feature selection, Mixed data, Feature ranking, Feature subset selection
AI 理解论文
溯源树
样例
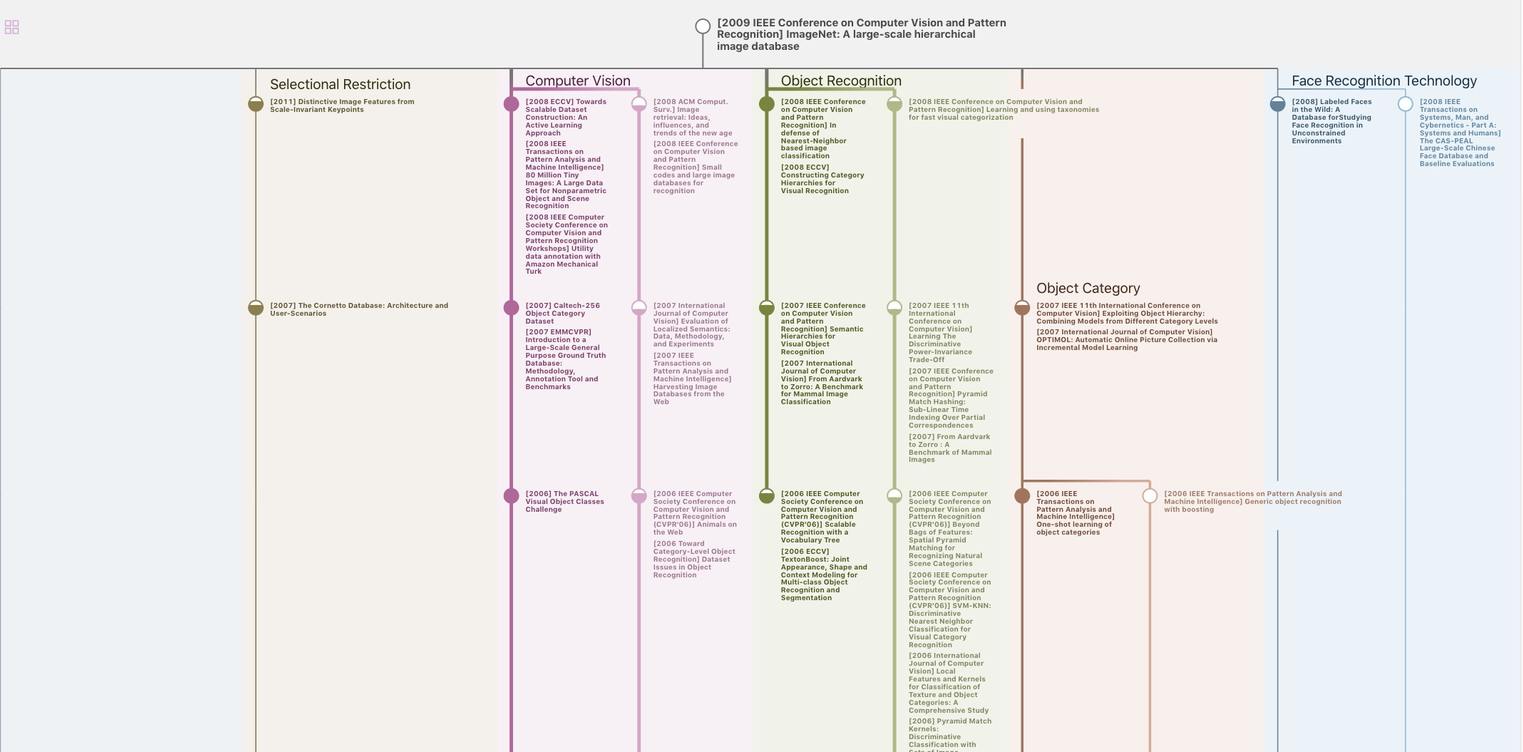
生成溯源树,研究论文发展脉络
Chat Paper
正在生成论文摘要