Predicting Motion Of Vulnerable Road Users Using High-Definition Maps And Efficient Convnets
2020 IEEE INTELLIGENT VEHICLES SYMPOSIUM (IV)(2020)
摘要
Following detection and tracking of traffic actors, prediction of their future motion is the next critical component of a self-driving vehicle (SDV) technology, allowing the SDV to operate safely and efficiently in its environment. This is particularly important when it comes to vulnerable road users (VRUs), such as pedestrians and bicyclists. These actors need to be handled with special care due to an increased risk of injury, as well as the fact that their behavior is less predictable than that of motorized actors. To address this issue, in the current study we present a deep learning-based method for predicting VRU movement, where we rasterize high-definition maps and actor's surroundings into a bird's-eye view image used as an input to deep convolutional networks. In addition, we propose a fast architecture suitable for real-time inference, and perform an ablation study of various rasterization approaches to find the optimal choice for accurate prediction. The results strongly indicate benefits of using the proposed approach for motion prediction of VRUs, both in terms of accuracy and latency.
更多查看译文
关键词
vulnerable road users,high-definition maps,ConvNets,tracking,traffic actors,critical component,self-driving vehicle technology,SDV,VRUs,pedestrians,bicyclists,special care,motorized actors,deep learning-based method,actor,bird,deep convolutional networks,motion prediction
AI 理解论文
溯源树
样例
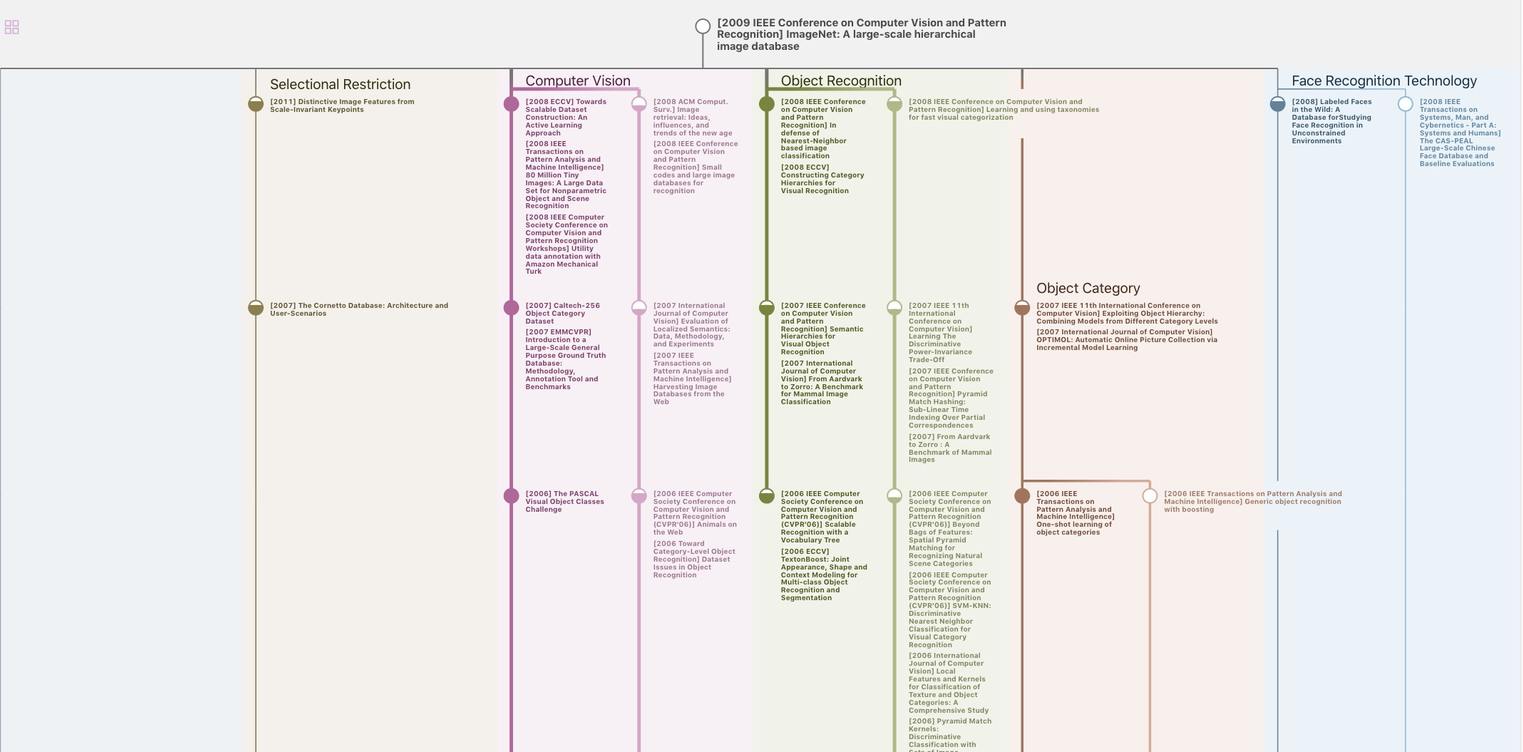
生成溯源树,研究论文发展脉络
Chat Paper
正在生成论文摘要