Sparse Signal Recovery with Minimization of 1-Norm Minus 2-Norm
IEEE Transactions on Vehicular Technology(2019)
摘要
The key aim of compressed sensing is to stably recover a
$K$
-sparse signals
${\boldsymbol{x}}$
from a linear model
${\boldsymbol{y}}=\boldsymbol{A}{\boldsymbol{x}}+\boldsymbol{v}$
, where
$\boldsymbol{v}$
is a noise vector. Minimization of
$\Vert {\boldsymbol{x}}\Vert _1-\Vert {\boldsymbol{x}}\Vert _2$
is a recently proposed effective recovery method. In this paper, we show that if the mutual coherence
$\mu$
of
$\boldsymbol{A}$
satisfies
$\mu < \frac{1}{3K}$
, then this method can stably recover any
$K$
-sparse signal
${\boldsymbol{x}}$
based on
${\boldsymbol{y}}$
and
$\boldsymbol{A}$
. As far as we know, this is the first sufficient condition based on mutual coherence for such method.
更多查看译文
关键词
Coherence,Matching pursuit algorithms,Sensors,Sparse matrices,Gaussian noise,Wireless sensor networks,Minimization
AI 理解论文
溯源树
样例
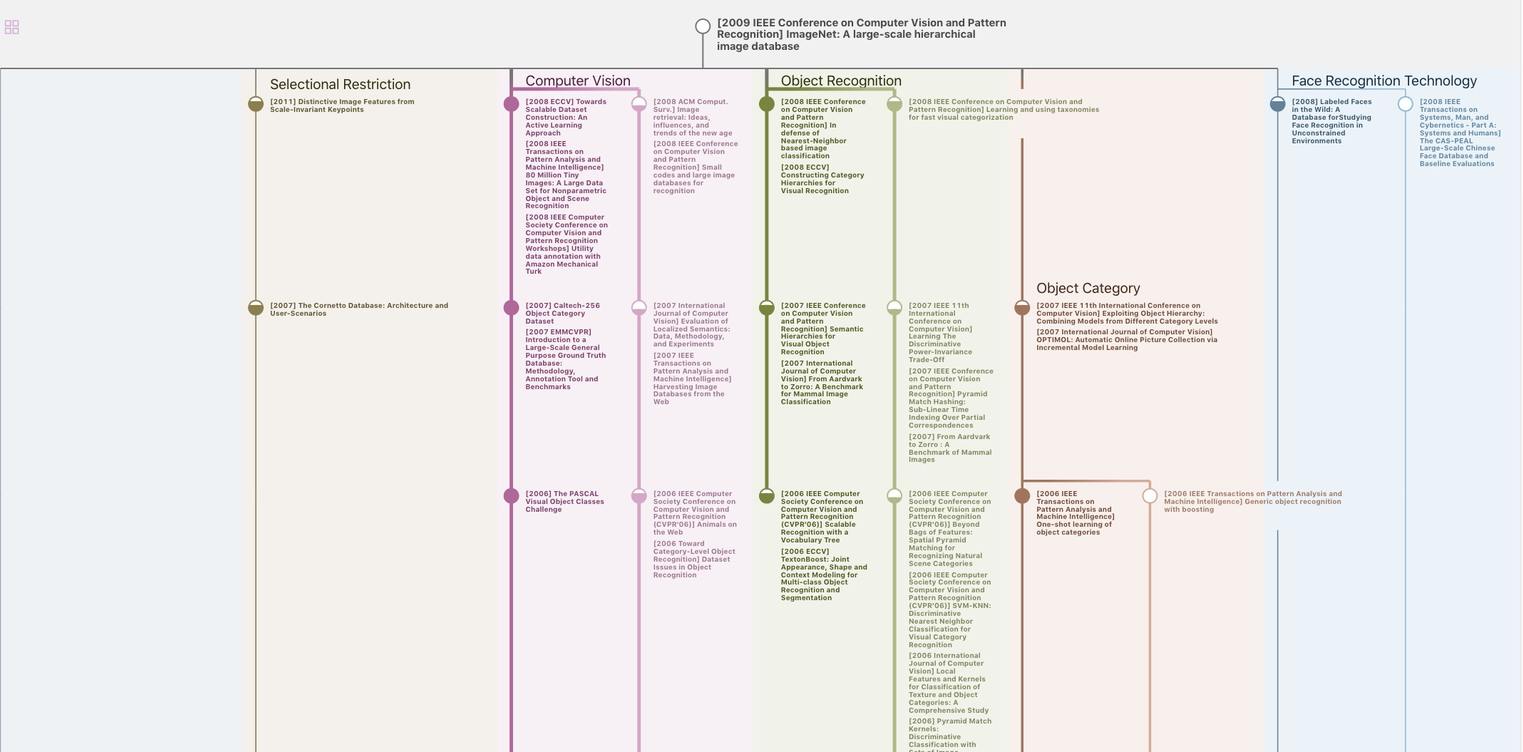
生成溯源树,研究论文发展脉络
Chat Paper
正在生成论文摘要