A lighten CNN-LSTM model for speaker verification on embedded devices
Future Generation Computer Systems(2019)
摘要
Augmented by deep learning methods, the performance of speaker recognition pipeline has been drastically boosted. For the scenario of smart home, the algorithms of speaker recognition should be user friendly and has high speed, high precision and low resource demand. However, most of the existing algorithms are designed without considering these four performance requirements simultaneously. To fill this gap, this paper proposes a text-independent speaker verification model. Specifically, the lighten network scheme is constructed using one convolution layer, two bilateral Long Short-term Memory (LSTM) layers and one fully connected layer. Utterance segments are mapped to a hypersphere where cosine similarity is used to measure the degree of difference between speakers. Then we analyze the defects of Additive Angular Margin (AAM) loss and propose a 3-stage training method. Softmax pre-training is used for avoiding divergence. After pre-training, AAM loss is adopted to boost training process. In the end, we use triplet loss to further fine-tune the model. Short-term speech utterances are used in training and testing. The experimental results demonstrate that the proposed model reaches 1.17% Equal Error Rate (EER) on a 200 persons benchmark with real-time inference speed on a generic embedded device.
更多查看译文
AI 理解论文
溯源树
样例
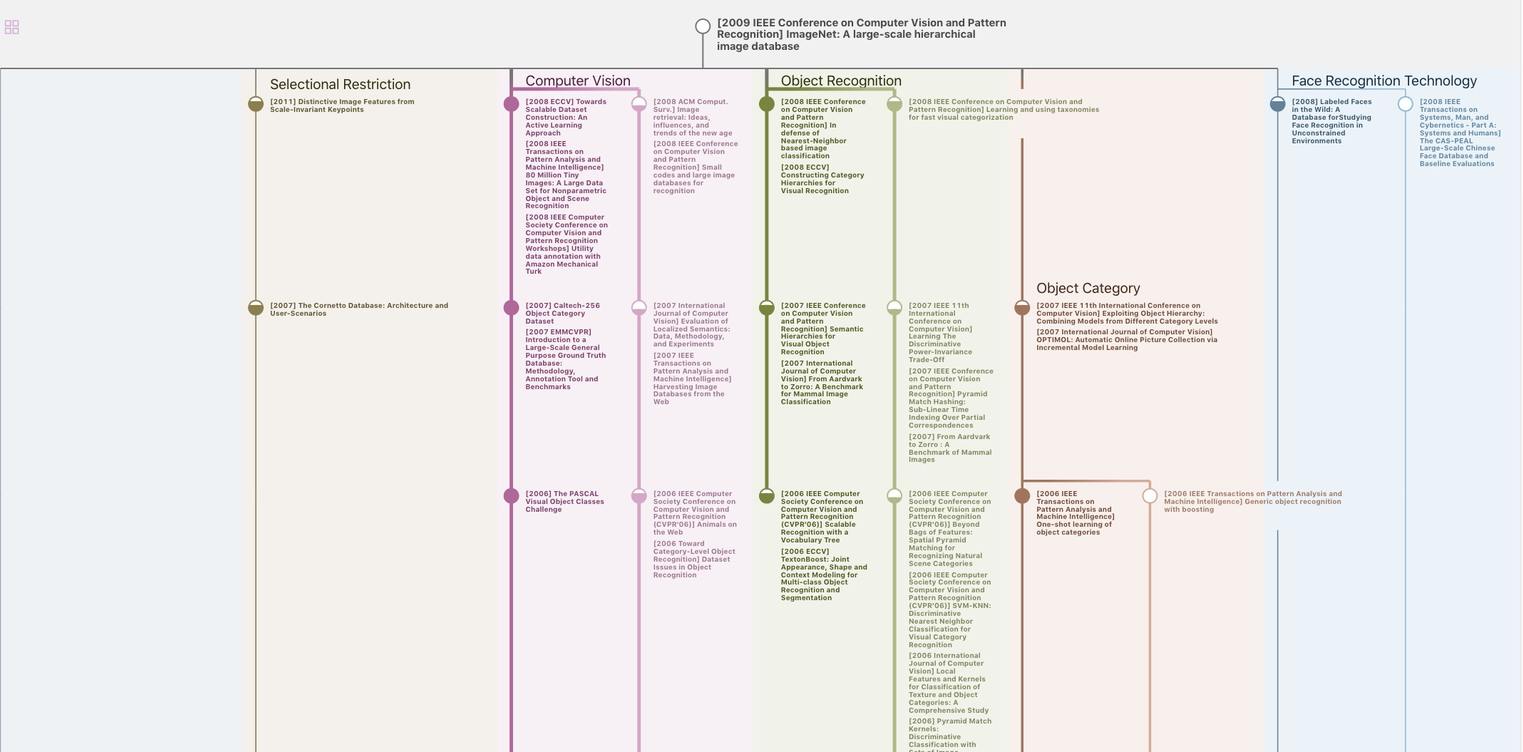
生成溯源树,研究论文发展脉络
Chat Paper
正在生成论文摘要