Radar: Rare Category Detection Via Computation Of Boundary Degree
PAKDD'11: Proceedings of the 15th Pacific-Asia conference on Advances in knowledge discovery and data mining - Volume Part II(2011)
摘要
Rare category detection is an open challenge for active learning. It can help select anomalies and then query their class labels with human experts. Compared with traditional anomaly detection, this task does not focus on finding individual and isolated instances. Instead, it selects interesting and useful anomalies from small compact clusters. Furthermore, the goal of rare category detection is to request as few queries as possible to find at least one representative data point from each rare class. Previous research works can be divided into three major groups, model-based, density-based and clustering-based methods. Performance of these approaches is affected by the local densities of the rare classes. In this paper, we develop a density insensitive method for rare category detection called RADAR. It makes use of reverse k-nearest neighbors to measure the boundary degree of each data point, and then selects examples with high boundary degree for the class-label querying. Experimental results on both synthetic and real-world data sets demonstrate the effectiveness of our algorithm.
更多查看译文
关键词
active learning,anomaly detection,rare category detection
AI 理解论文
溯源树
样例
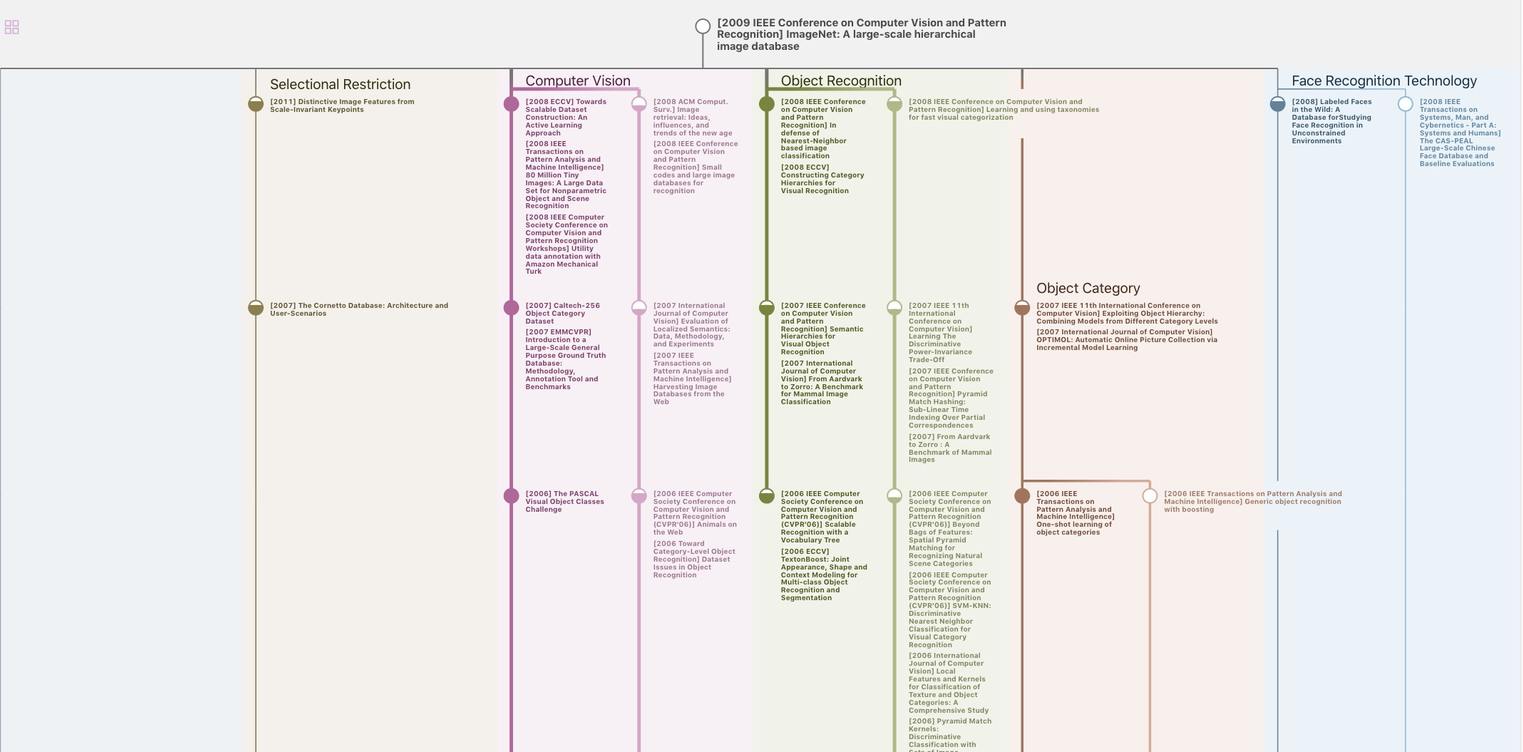
生成溯源树,研究论文发展脉络
Chat Paper
正在生成论文摘要