Machine learning approach of automatic identification and counting of blood cells.
HEALTHCARE TECHNOLOGY LETTERS(2019)
摘要
A complete blood cell count is an important test in medical diagnosis to evaluate overall health condition. Traditionally blood cells are counted manually using haemocytometer along with other laboratory equipment's and chemical compounds, which is a time-consuming and tedious task. In this work, the authors present a machine learning approach for automatic identification and counting of three types of blood cells using 'you only look once' (YOLO) object detection and classification algorithm. YOLO framework has been trained with a modified configuration BCCD Dataset of blood smear images to automatically identify and count red blood cells, white blood cells, and platelets. Moreover, this study with other convolutional neural network architectures considering architecture complexity, reported accuracy, and running time with this framework and compare the accuracy of the models for blood cells detection. They also tested the trained model on smear images from a different dataset and found that the learned models are generalised. Overall the computer-aided system of detection and counting enables us to count blood cells from smear images in less than a second, which is useful for practical applications.
更多查看译文
关键词
automatic identification,blood,blood cells detection,blood smear images,cellular biophysics,complete blood cell count,counting,image classification,learning (artificial intelligence),machine learning approach,medical image processing,neural nets,object detection,patient diagnosis,red blood cells,white blood cells
AI 理解论文
溯源树
样例
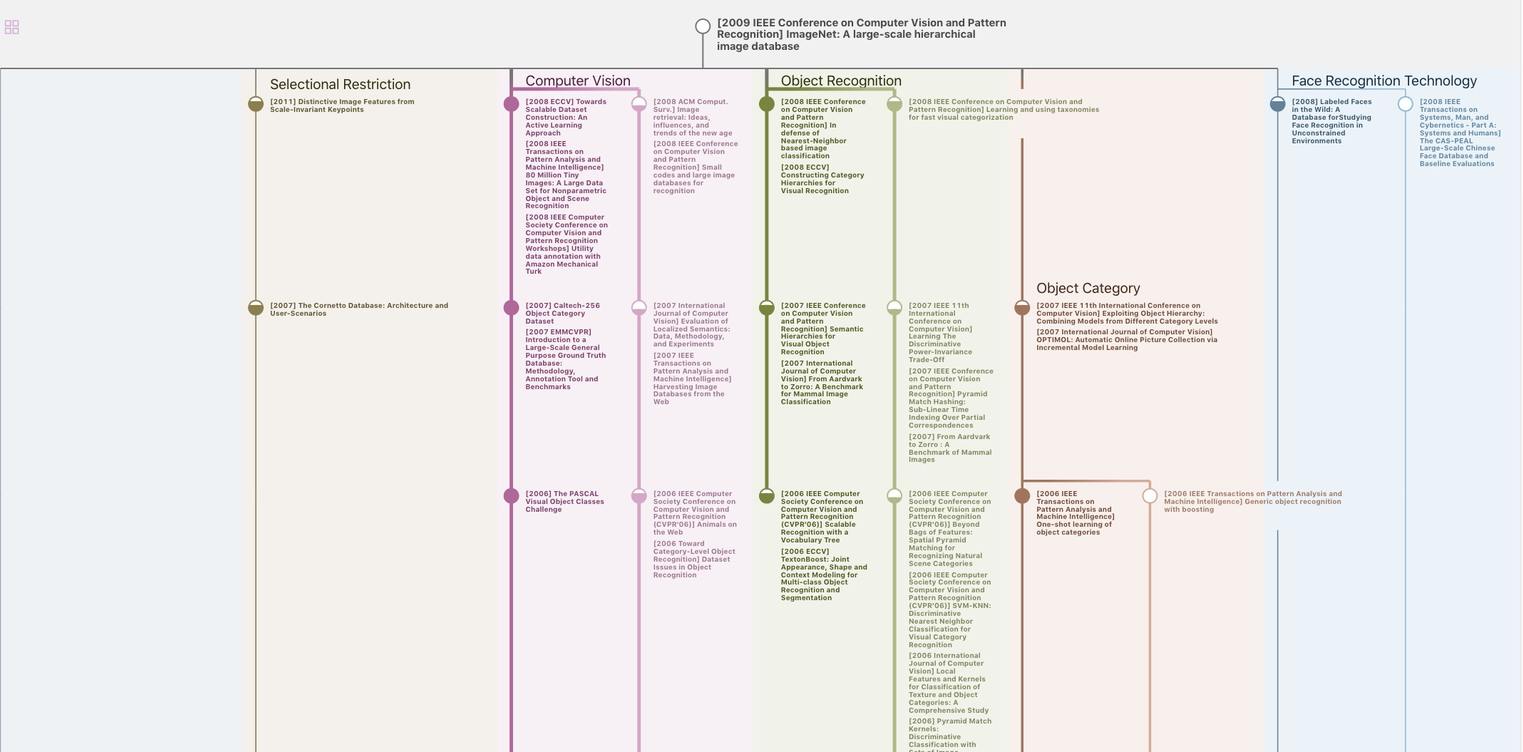
生成溯源树,研究论文发展脉络
Chat Paper
正在生成论文摘要