Perceptual image quality using dual generative adversarial network
NEURAL COMPUTING & APPLICATIONS(2019)
摘要
Generative adversarial networks have received a remarkable success in many computer vision applications for their ability to learn from complex data distribution. In particular, they are capable to generate realistic images from latent space with a simple and intuitive structure. The main focus of existing models has been improving the performance; however, there is a little attention to make a robust model. In this paper, we investigate solutions to the super-resolution problems—in particular perceptual quality—by proposing a robust GAN. The proposed model unlike the standard GAN employs two generators and two discriminators in which, a discriminator determines that the samples are from real data or generated one, while another discriminator acts as classifier to return the wrong samples to its corresponding generators. Generators learn a mixture of many distributions from prior to the complex distribution. This new methodology is trained with the feature matching loss and allows us to return the wrong samples to the corresponding generators, in order to regenerate the real-look samples. Experimental results in various datasets show the superiority of the proposed model compared to the state of the art methods.
更多查看译文
关键词
Image processing,Perceptual quality,Data distribution,Generative adversarial network,Classification
AI 理解论文
溯源树
样例
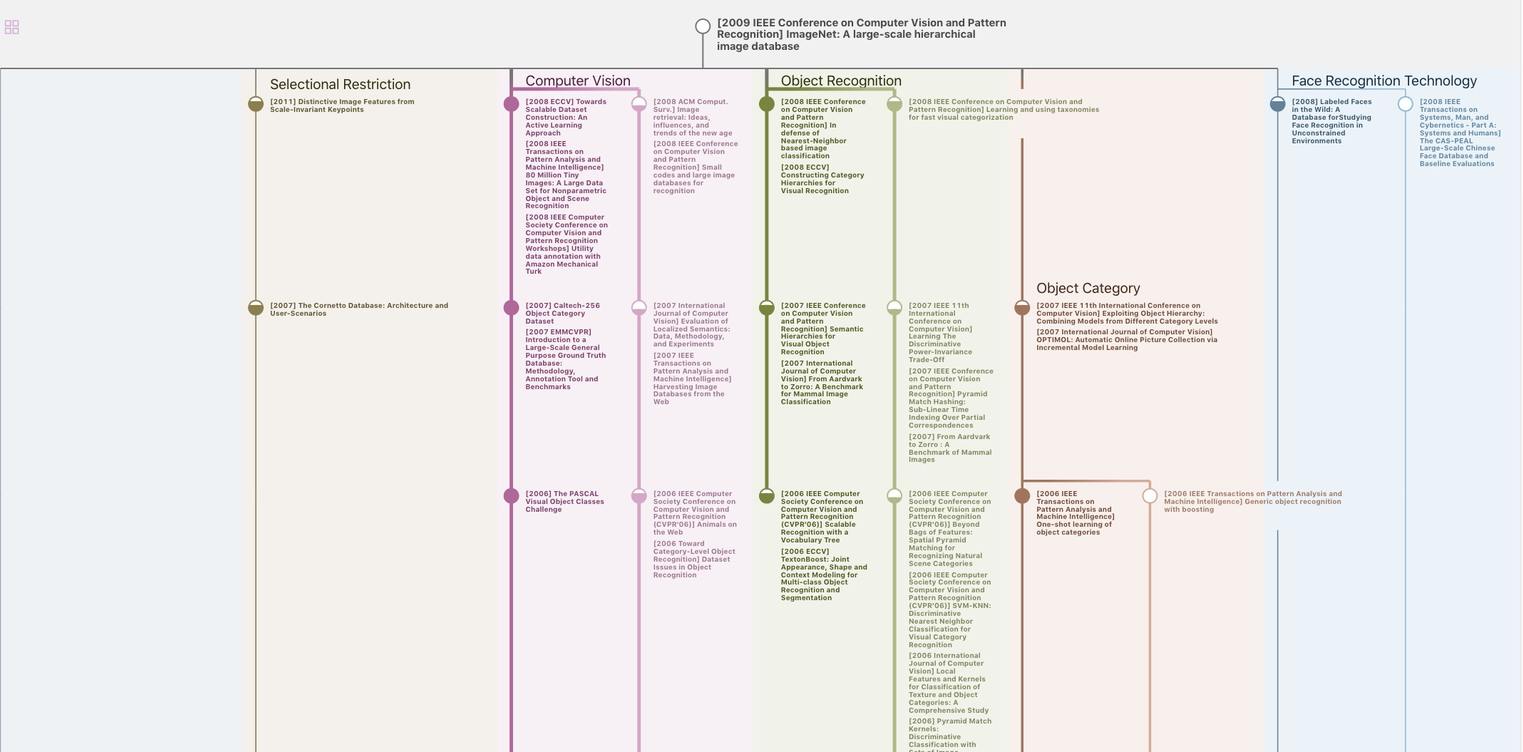
生成溯源树,研究论文发展脉络
Chat Paper
正在生成论文摘要