Deep face recognition with weighted center loss
Proceedings of SPIE(2019)
摘要
ID face photos are widely used for identity verification in many real-world applications. In these cases, the face from ID photo is expected to compare with the face obtained from the daily life image. To improve the ID/life face verification performance, the datasets containing various ID face photos and daily life face images are always needed to train an available CNN model for feature extraction. However, it's really hard to get uniform distributed data for both the ID photos and daily life images. In most face datasets, each subject only contains one ID photo (for example, obtained from the identity card) while contains varies of life images. This imbalanced distribution causes the difficulty of learning a general deep feature representation for both the ID and life faces, since the daily life images tend to have a higher impact on the feature learning than the ID photos. To address this challenge, we propose a weighted center loss which aims to learn a center of deep features for each class and penalizes the distance between the image features and their corresponding class centers with different weights. By emphasizing the ID photo features in the loss computation, our weighted center loss can train a general CNN which obviously narrows the distance between the ID and life faces. Final experiments demonstrate that with the joint supervision of softmax and weighted center loss, our proposed framework can minimize the intra-class variations caused by the imbalanced ID/life data distribution and significantly improve the ID face based verification accuracy.
更多查看译文
关键词
face recognition,convolutional neural network,weighted center loss
AI 理解论文
溯源树
样例
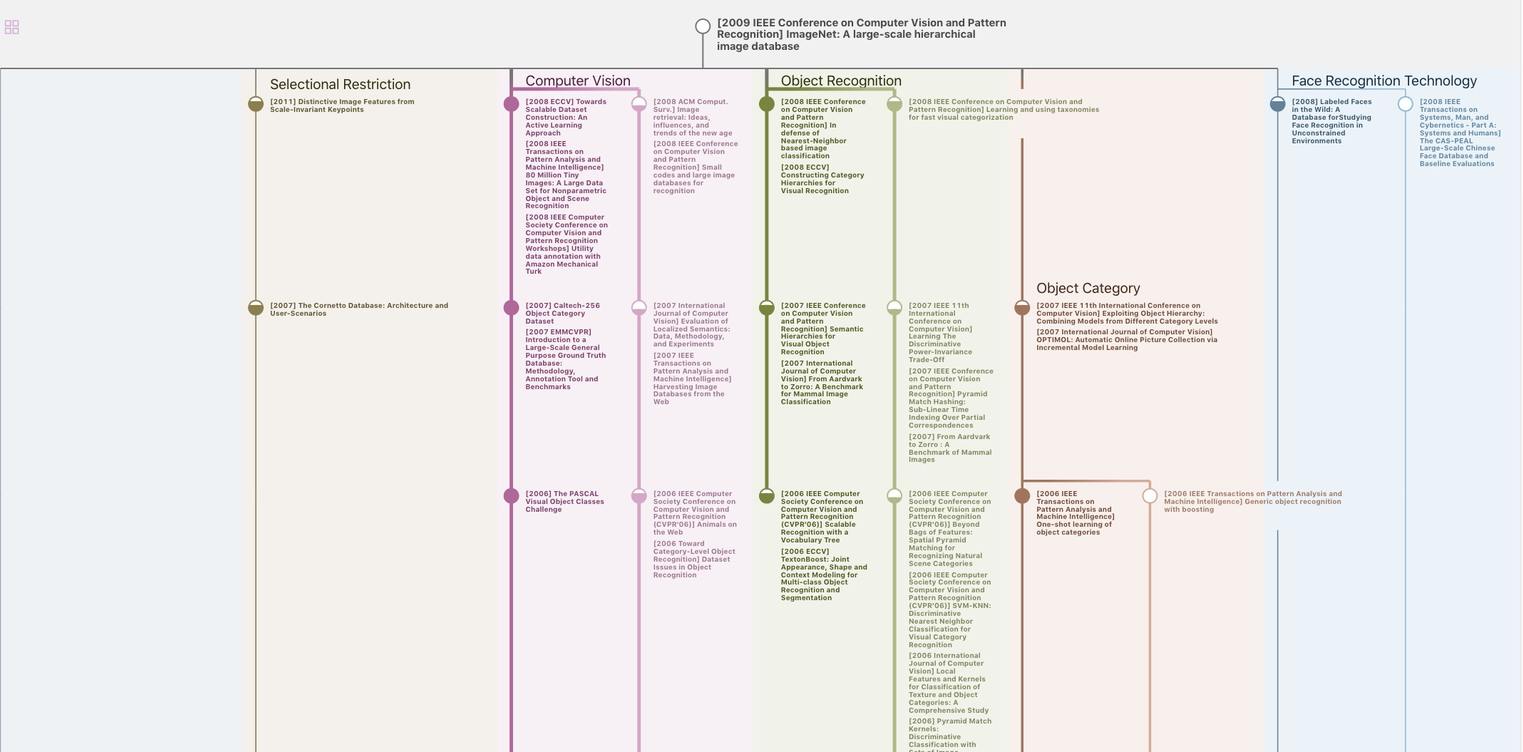
生成溯源树,研究论文发展脉络
Chat Paper
正在生成论文摘要