Heart sounds classification using a novel 1-D convolutional neural network with extremely low parameter consumption
Neurocomputing(2020)
摘要
Automatic heart sound auscultation is one of the common used techniques for cardiovascular diseases detection. In this paper, a novel heart sound classification method based on deep learning technologies for cardiovascular disease prediction is introduced, which is mainly comprised three parts: pre-processing, 1-D waveform heart sound patches classification using a deep convolutional neural network (CNN) with attention mechanism, and majority voting for final prediction of heart sound recordings. In order to enhance the information flow of the CNNs, a block-stacked style architecture with clique blocks is employed, and in each clique block a bidirectional connection structure is introduced in the proposed CNN. By using the stacked clique and transition blocks, the proposed CNN achieves both spatial and channel attention leading a promising classification performance. Moreover, a novel separable convolution with inverted bottleneck is utilized to decouple the spatial and channel-wise relevancy of features efficiently. Experiments on PhysioNet/CinC 2016 show that the proposed method obtains a superior classification results and excels in consumption of parameter comparing to state-of-the-art methods.
更多查看译文
关键词
Cardiovascular disease detection,Heart sounds classification,Convolutional neural network,Attention mechanism,Separable convolution
AI 理解论文
溯源树
样例
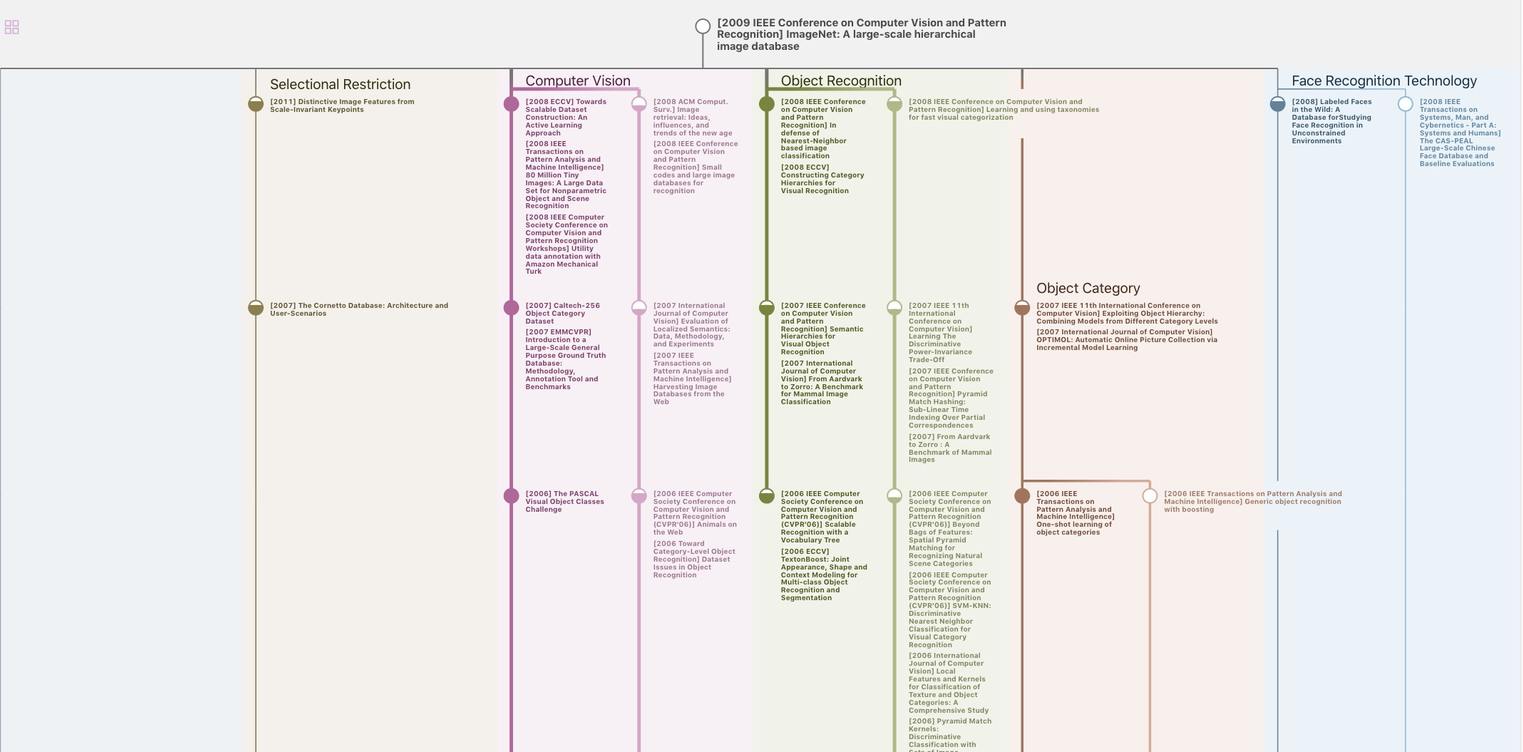
生成溯源树,研究论文发展脉络
Chat Paper
正在生成论文摘要