Sparse ReRAM engine: joint exploration of activation and weight sparsity in compressed neural networks
Proceedings of the 46th International Symposium on Computer Architecture(2019)
摘要
Exploiting model sparsity to reduce ineffectual computation is a commonly used approach to achieve energy efficiency for DNN inference accelerators. However, due to the tightly coupled crossbar structure, exploiting sparsity for ReRAM-based NN accelerator is a less explored area. Existing architectural studies on ReRAM-based NN accelerators assume that an entire crossbar array can be activated in a single cycle. However, due to inference accuracy considerations, matrix-vector computation must be conducted in a smaller granularity in practice, called Operation Unit (OU). An OU-based architecture creates a new opportunity to exploit DNN sparsity. In this paper, we propose the first practical Sparse ReRAM Engine that exploits both weight and activation sparsity. Our evaluation shows that the proposed method is effective in eliminating ineffectual computation, and delivers significant performance improvement and energy savings.
更多查看译文
关键词
ReRAM, accelerator architecture, neural network, sparsity
AI 理解论文
溯源树
样例
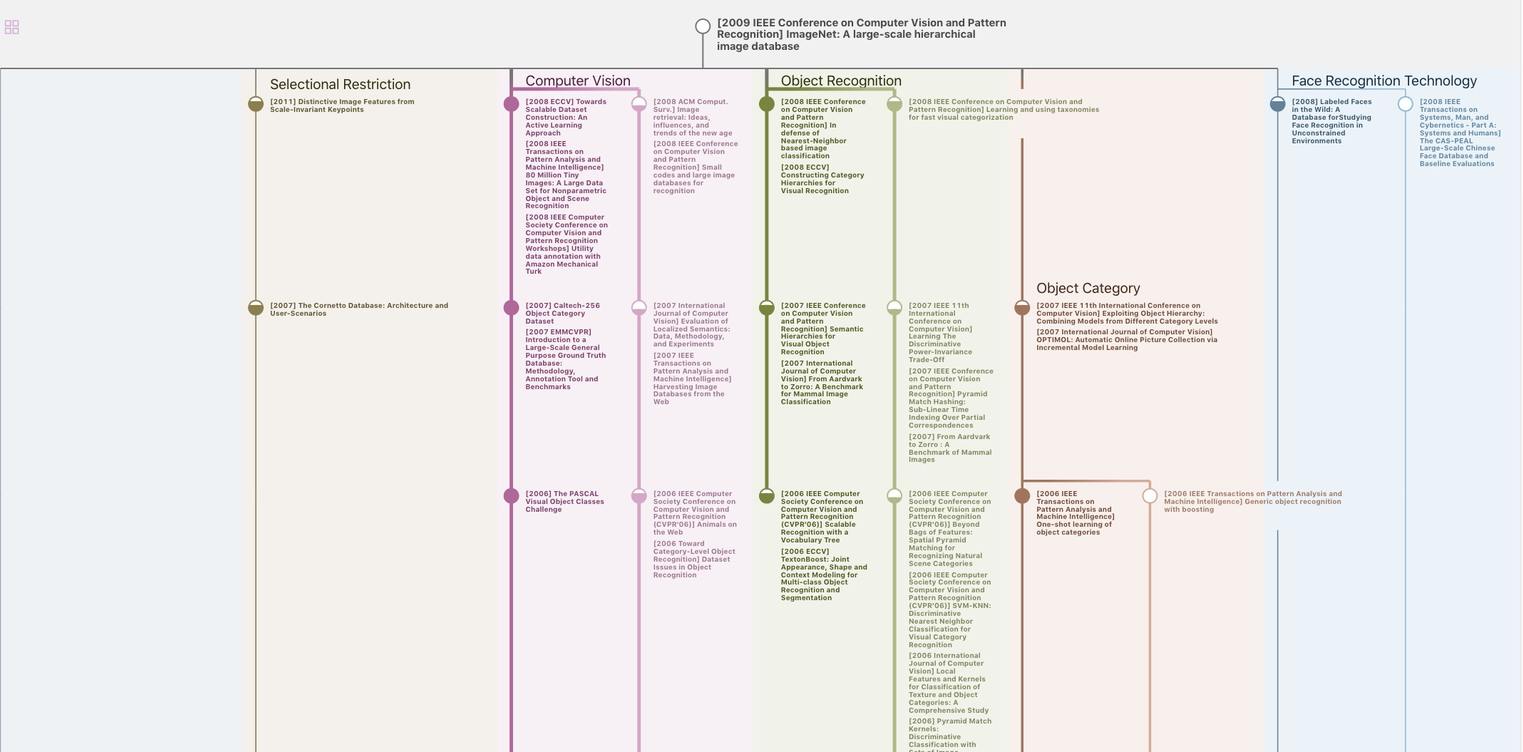
生成溯源树,研究论文发展脉络
Chat Paper
正在生成论文摘要