Looking South: Learning Urban Perception in Developing Cities
ACM Transactions on Social Computing(2018)
摘要
Mobile and social technologies are providing new opportunities to document, characterize, and gather impressions of urban environments. In this article, we present a study that examines urban perceptions of three cities in central Mexico; the study integrates a mobile crowdsourcing framework to collect geo-localized images of urban environments by a local youth community, an online crowdsourcing platform to gather impressions of urban environments along 12 physical and psychological dimensions, and a deep learning framework to automatically infer human impressions of outdoor urban scenes. Our study resulted in a collection of 7,000 geo-localized images containing outdoor scenes and views of each city’s built environment, including touristic, historical, and residential neighborhoods, and 144,000 individual judgments from Amazon Mechanical Turk. Statistical analyses show that outdoor environments can be assessed in terms of interrater agreement for most of the urban dimensions by the observers of crowdsourced images. Furthermore, we proposed a methodology to automatically infer human perceptions of outdoor scenes using a variety of low-level image features and generic deep learning (CNN) features. We found that CNN features consistently outperformed all the individual low-level image features for all the studied urban dimensions. We obtained a maximum R2 of 0.49 using CNN features; for 9 out of 12 labels, the obtained R2 values exceeded 0.44.
更多查看译文
关键词
ICTD, Mexico, Mobile crowdsourcing, collective action, deep learning, outdoor places, urban perception
AI 理解论文
溯源树
样例
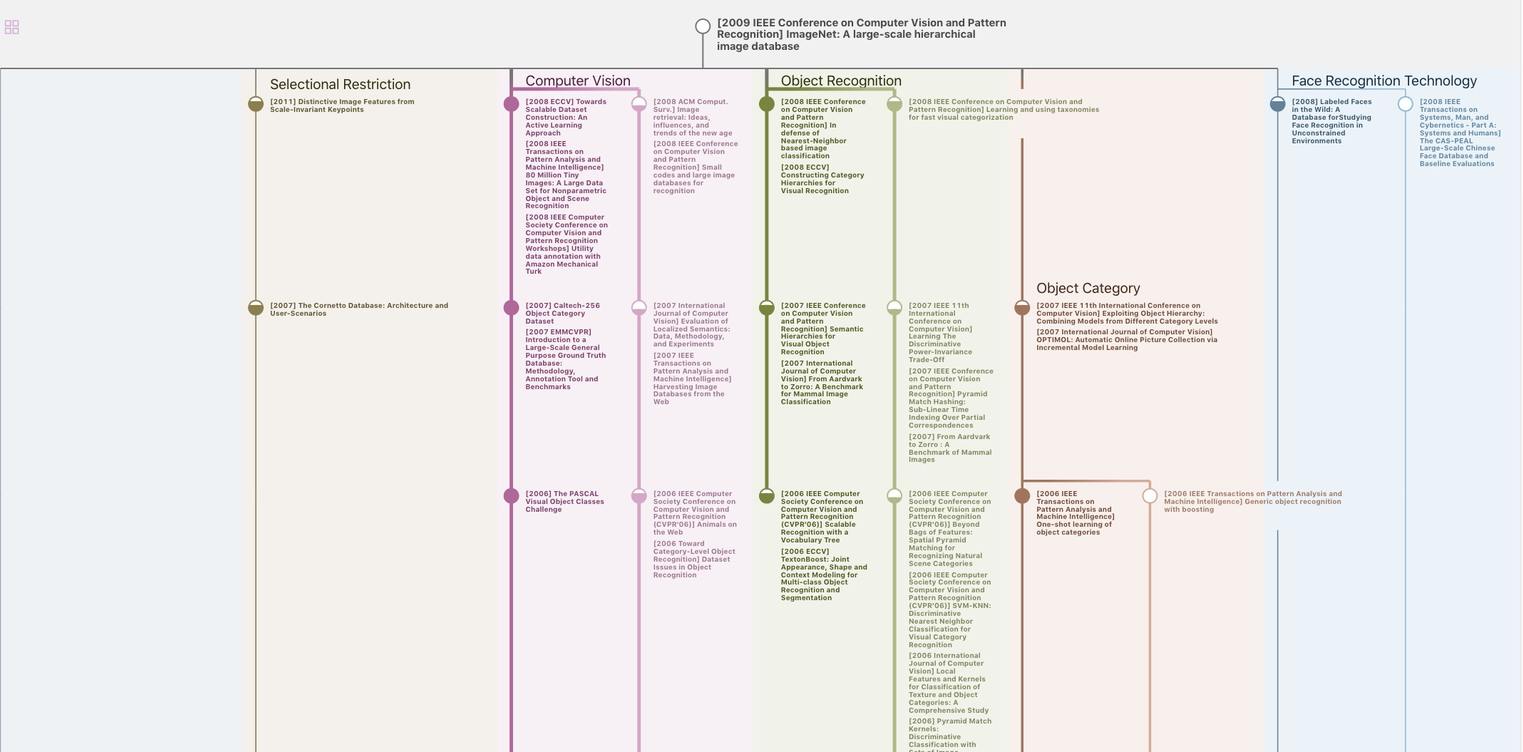
生成溯源树,研究论文发展脉络
Chat Paper
正在生成论文摘要