Accurate Low Rank Approximation at a Low Computational Cost
arxiv(2019)
摘要
Given an algorithm A for computing a crude Low Rank Approximation (LRA) of a
matrix having fast decaying spectra of singular values, we greatly decreased
the spectral norm of its output error matrix at sublinear additional
computational cost. Furthermore, for a variety of synthetic and real world
inputs we tested numerically variants where our entire algorithms run at
sublinear cost, and in most cases we still obtained accurate or even
near-optimal LRAs. Towards enhancing our progress to a larger class of
matrices, we nontrivially extended to LRA the popular technique of iterative
refinement.
更多查看译文
AI 理解论文
溯源树
样例
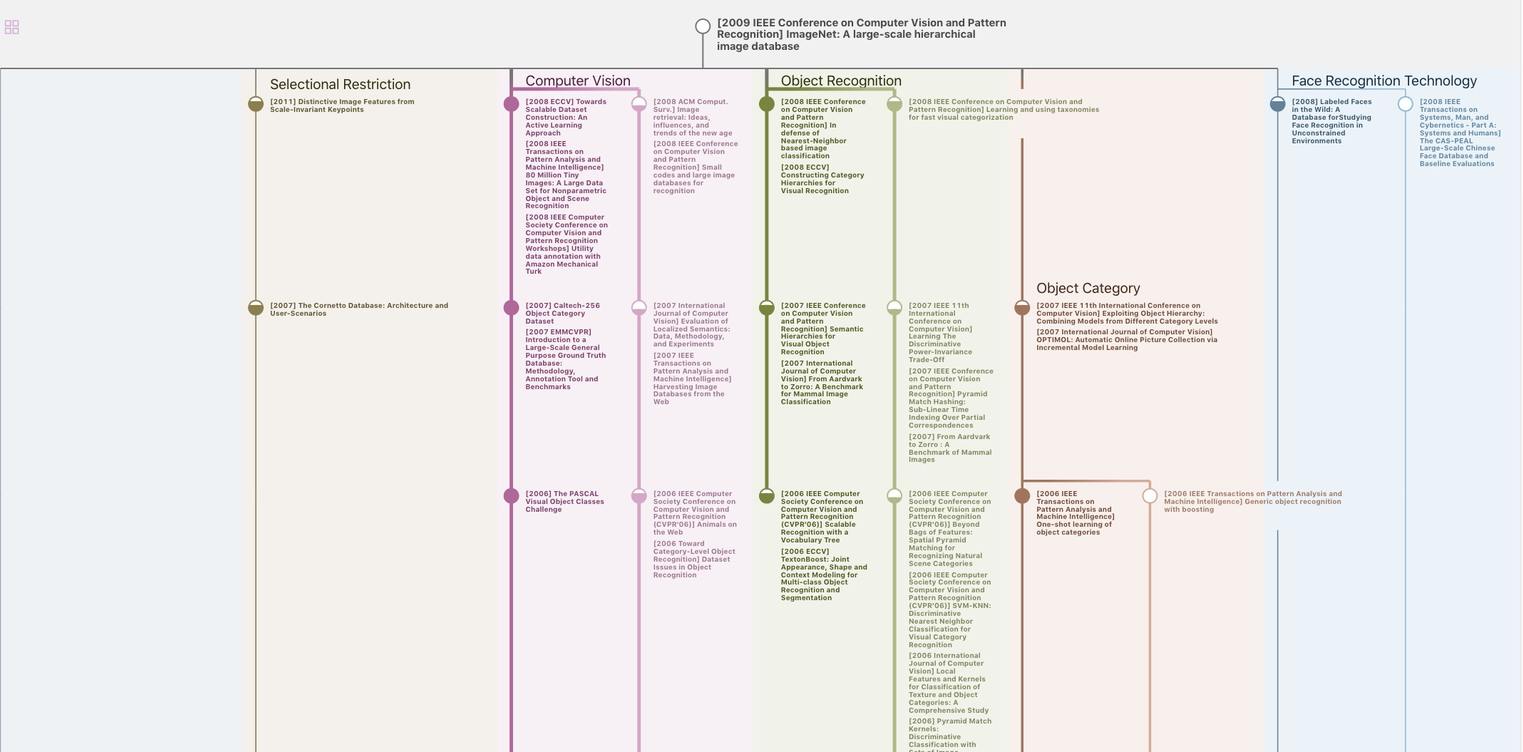
生成溯源树,研究论文发展脉络
Chat Paper
正在生成论文摘要