Deep Spatio-Temporal Neural Networks for Click-Through Rate Prediction
KDD'19: PROCEEDINGS OF THE 25TH ACM SIGKDD INTERNATIONAL CONFERENCCE ON KNOWLEDGE DISCOVERY AND DATA MINING(2019)
摘要
Click-through rate (CTR) prediction is a critical task in online advertising systems. A large body of research considers each ad independently, but ignores its relationship to other ads that may impact the CTR. In this paper, we investigate various types of auxiliary ads for improving the CTR prediction of the target ad. In particular, we explore auxiliary ads from two viewpoints: one is from the spatial domain, where we consider the contextual ads shown above the target ad on the same page; the other is from the temporal domain, where we consider historically clicked and unclicked ads of the user. The intuitions are that ads shown together may influence each other, clicked ads reflect a user's preferences, and unclicked ads may indicate what a user dislikes to certain extent. In order to effectively utilize these auxiliary data, we propose the Deep Spatio-Temporal neural Networks (DSTNs) for CTR prediction. Our model is able to learn the interactions between each type of auxiliary data and the target ad, to emphasize more important hidden information, and to fuse heterogeneous data in a unified framework. Offline experiments on one public dataset and two industrial datasets show that DSTNs outperform several state-of-the-art methods for CTR prediction. We have deployed the best-performing DSTN in Shenma Search, which is the second largest search engine in China. The A/B test results show that the online CTR is also significantly improved compared to our last serving model.
更多查看译文
关键词
Click-through rate prediction,Online advertising,Deep learning
AI 理解论文
溯源树
样例
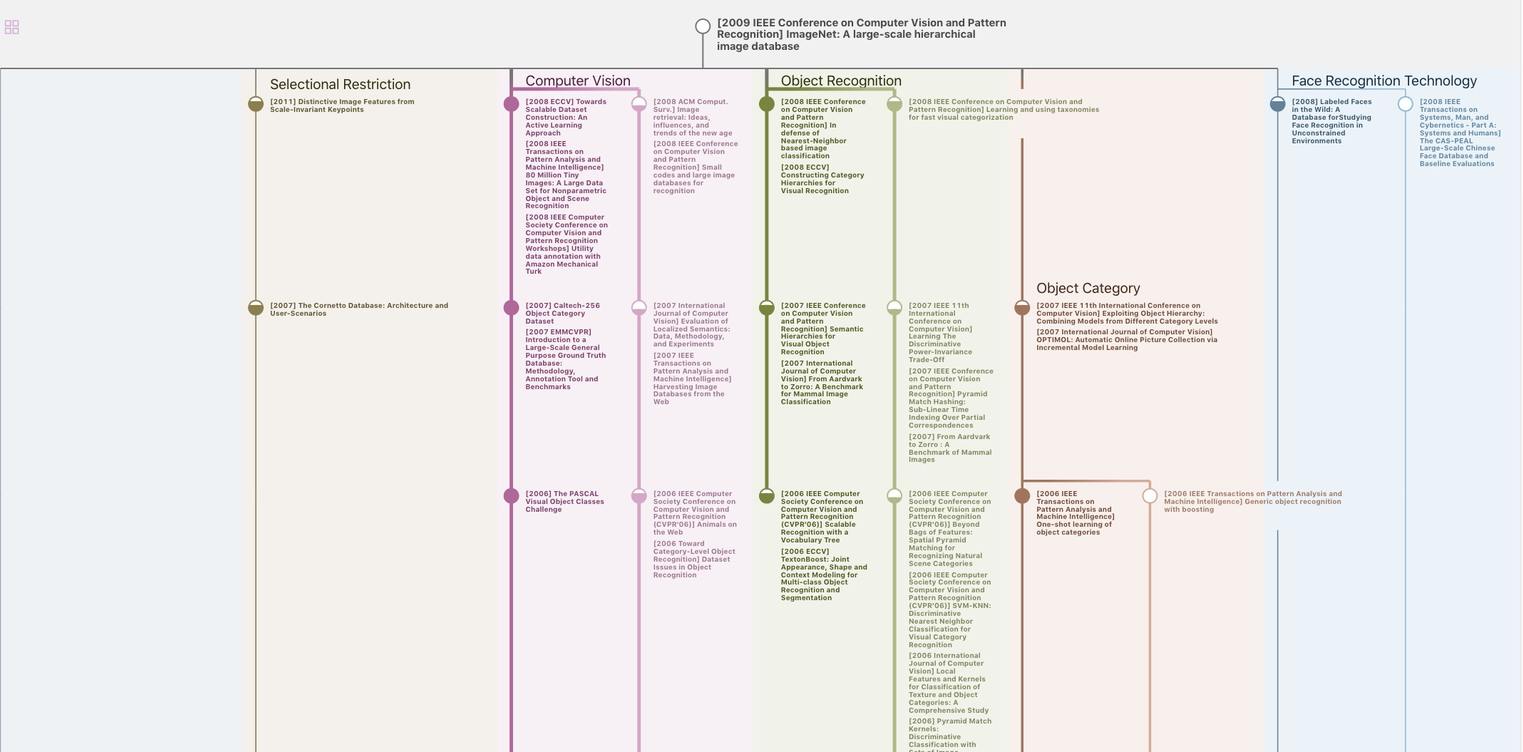
生成溯源树,研究论文发展脉络
Chat Paper
正在生成论文摘要