A Cost-Reducing Partial Labeling Estimator in Text Classification Problem
ADVANCES IN INFORMATION AND COMMUNICATION, VOL 2(2020)
摘要
The paper proposes a new approach to address the text classification problems when learning with partial labels is beneficial. Instead of offering each training sample a set of candidate labels, researchers assign negative-oriented labels to ambiguous training examples if they are unlikely falling into certain classes. Researchers construct two new maximum likelihood estimators with self-correction property, and prove that under some conditions, new estimators converge faster. Also the paper discusses the advantages of applying one of new estimators to a fully supervised learning problem. The proposed method has potential applicability in many areas, such as crowd-sourcing, natural language processing and medical image analysis.
更多查看译文
关键词
Machine learning,NLP,Text classification,Partial label,Naive Bayes,Maximum likelihood estimator,Self correction,Cost reducing
AI 理解论文
溯源树
样例
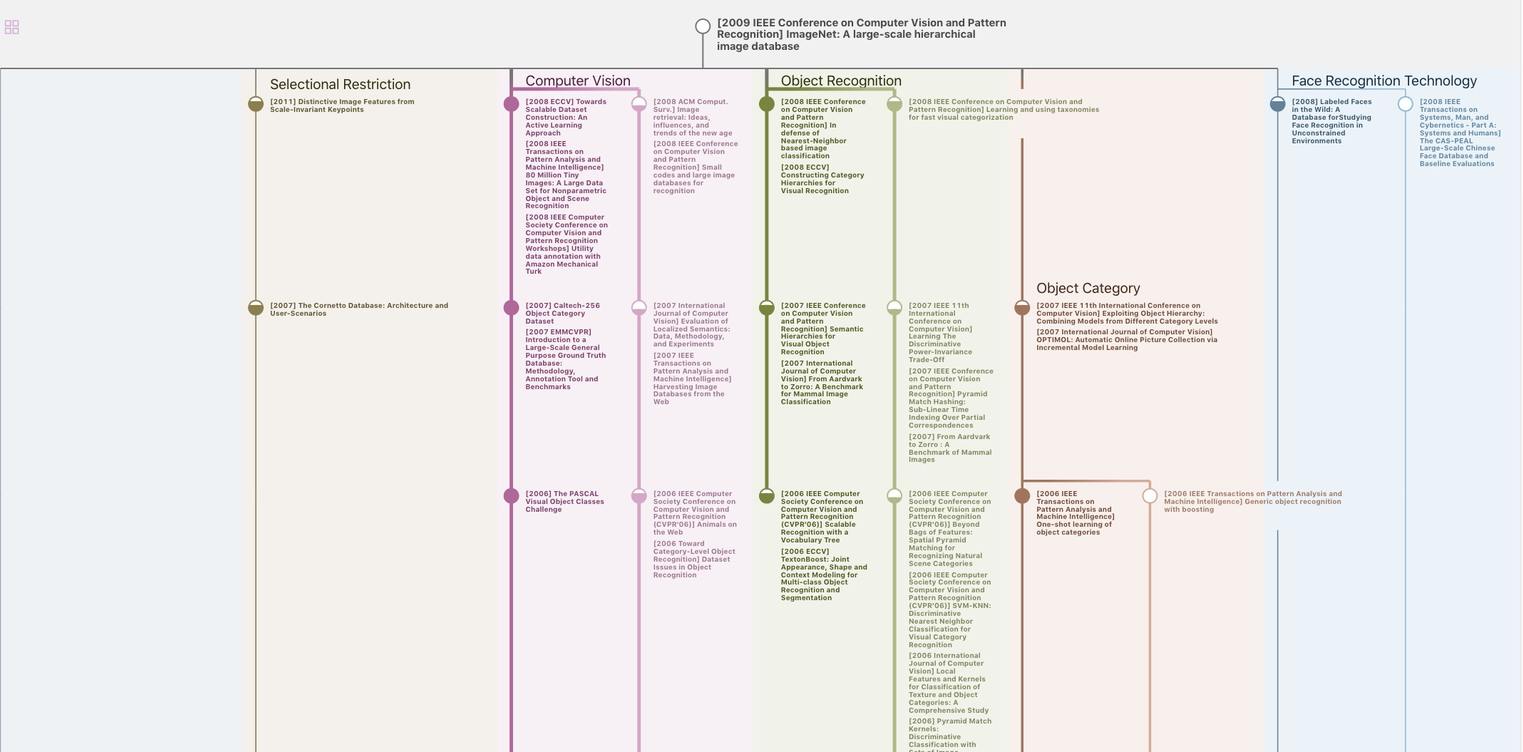
生成溯源树,研究论文发展脉络
Chat Paper
正在生成论文摘要