PLUS: performance learning for uncertainty of software
Proceedings of the 41st International Conference on Software Engineering: New Ideas and Emerging Results(2019)
摘要
Uncertainty is particularly critical in software performance engineering when it relates to the values of important parameters such as workload, operational profile, and resource demand, because such parameters inevitably affect the overall system performance. Prior work focused on monitoring the performance characteristics of software systems while considering influence of configuration options. The problem of incorporating uncertainty as a first-class concept in the software development process to identify performance issues is still challenging. The PLUS (Performance Learning for Uncertainty of Software) approach aims at addressing these limitations by investigating the specification of a new class of performance models capturing how the different uncertainties underlying a software system affect its performance characteristics. The main goal of PLUS is to answer a fundamental question in the software performance engineering domain: How to model the variable configuration options (i.e., software and hardware resources) and their intrinsic uncertainties (e.g., resource demand, processor speed) to represent the performance characteristics of software systems? This way, software engineers are exposed to a quantitative evaluation of their systems that supports them in the task of identifying performance critical configurations along with their uncertainties.
更多查看译文
关键词
machine learning, uncertainty
AI 理解论文
溯源树
样例
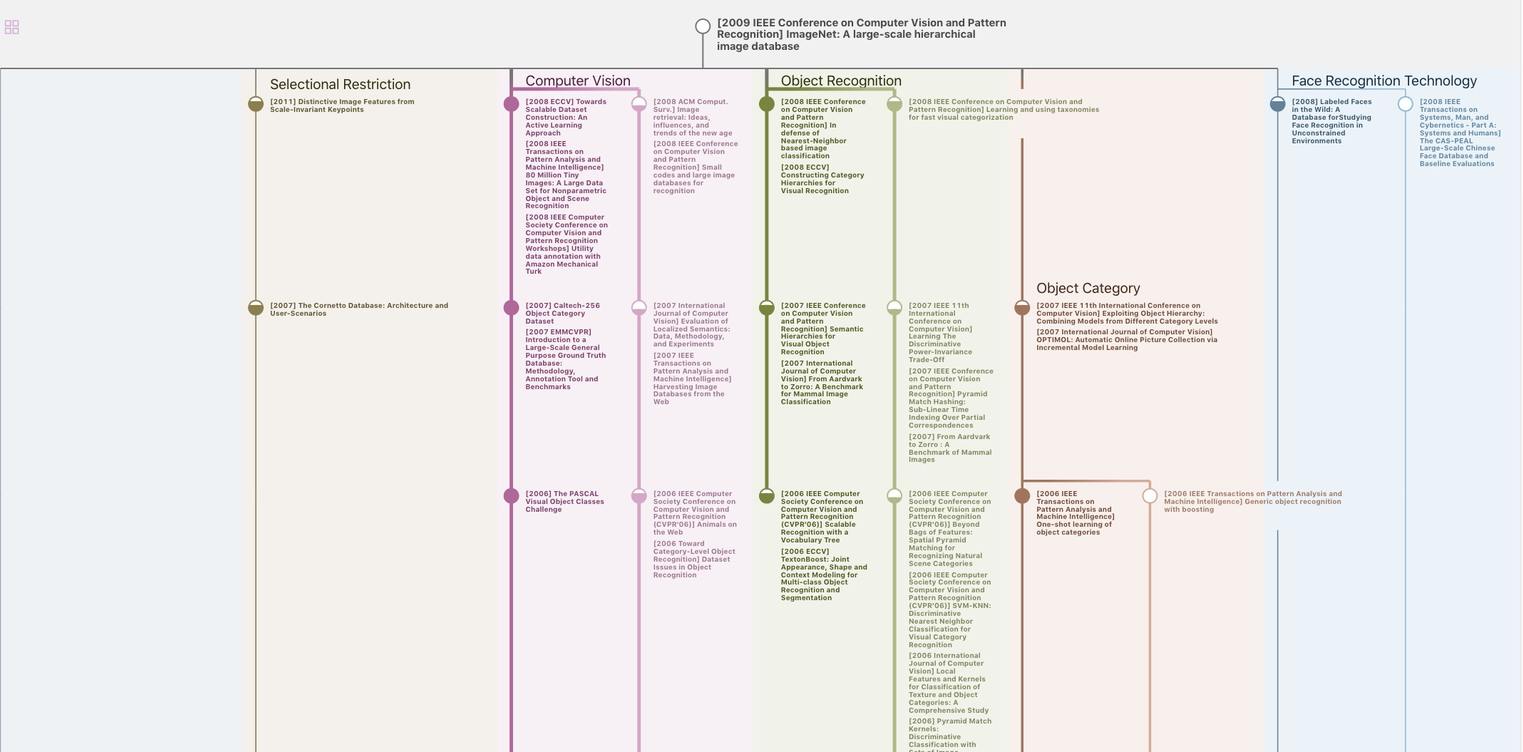
生成溯源树,研究论文发展脉络
Chat Paper
正在生成论文摘要