Modeling Decisions in Collective Risk Social Dilemma Games for Climate Change Using Reinforcement Learning
2019 IEEE Conference on Cognitive and Computational Aspects of Situation Management (CogSIMA)(2019)
摘要
Prior research has used reinforcement-learning (RL) models like Expectancy-Valence-Learning (EVL) and Prospect-Valence-Learning (PVL) to investigate human decisions in choice games. However, currently little is known on how RL models would account for human decisions in games where people face a collective risk social dilemma (CRSD) against societal problems like climate change. In CRSD game, a group of players invested some part of their private incomes to a public fund over several rounds with the goal of collectively reaching a climate target, failing which climate change would occur with a certain probability and players would lose their remaining incomes. Next EVL and PVL models were calibrated to human decisions across two between-subject conditions in CRSD (Info-all: N=120; No-Info: N=120), where half of the players in each condition possessed lesser wealth (poor) compared to the other half (rich). A symmetric Nash model was also run in both conditions as a benchmark. In Info-all condition, players possessed complete information on investments of other players after every round; whereas, in the No-info condition, players did not possess this information. Our results showed that for both rich and poor players, the EVL model performed better than the PVL model in No-info condition; however, the PVL model performed better than the EVL model in the Info condition. Both the EVL and PVL models outperformed the symmetric Nash model. Model parameters showed reliance on recency, reward-seeking, and exploitative behaviours. We highlight the implications of our model results for situations involving a collective risk social dilemma.
更多查看译文
关键词
Investment,Games,Computational modeling,Data models,Mathematical model
AI 理解论文
溯源树
样例
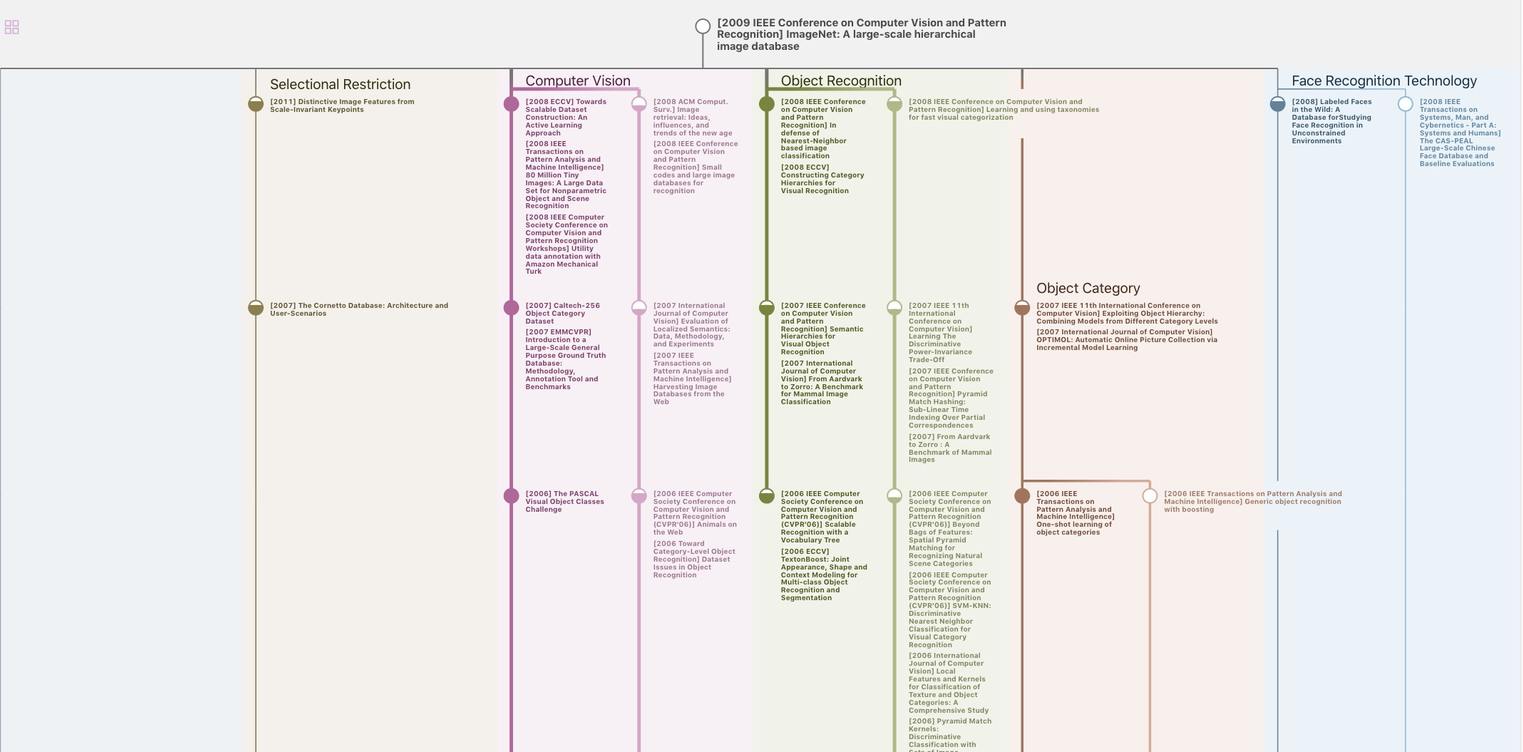
生成溯源树,研究论文发展脉络
Chat Paper
正在生成论文摘要