Identifying concepts from medical images via transfer learning and image retrieval.
MATHEMATICAL BIOSCIENCES AND ENGINEERING(2019)
摘要
Automatically identifying semantic concepts from medical images provides multimodal insights for clinical research. To study the effectiveness of concept detection on large scale medical images, we reconstructed over 230,000 medical image-concepts pairs collected from the ImageCLEFcaption 2018 evaluation task. A transfer learning-based multi-label classification model was used to predict multiple high-frequency concepts for medical images. Semantically relevant concepts of visually similar medical images were identified by the image retrieval-based topic model. The results showed that the transfer learning method achieved F1 score of 0.1298, which was comparable with the state of art methods in the ImageCLEFcaption tasks. The image retrieval-based method contributed to the recall performance but reduced the overall F1 score, since the retrieval results of the search engine introduced irrelevant concepts. Although our proposed method achieved second-best performance in the concept detection subtask of ImageCLEFcaption 2018, there will be plenty of further work to improve the concept detection with better understanding the medical images.
更多查看译文
关键词
concept detection,transfer learning,multi-label classification,medical image retrieval,LDA
AI 理解论文
溯源树
样例
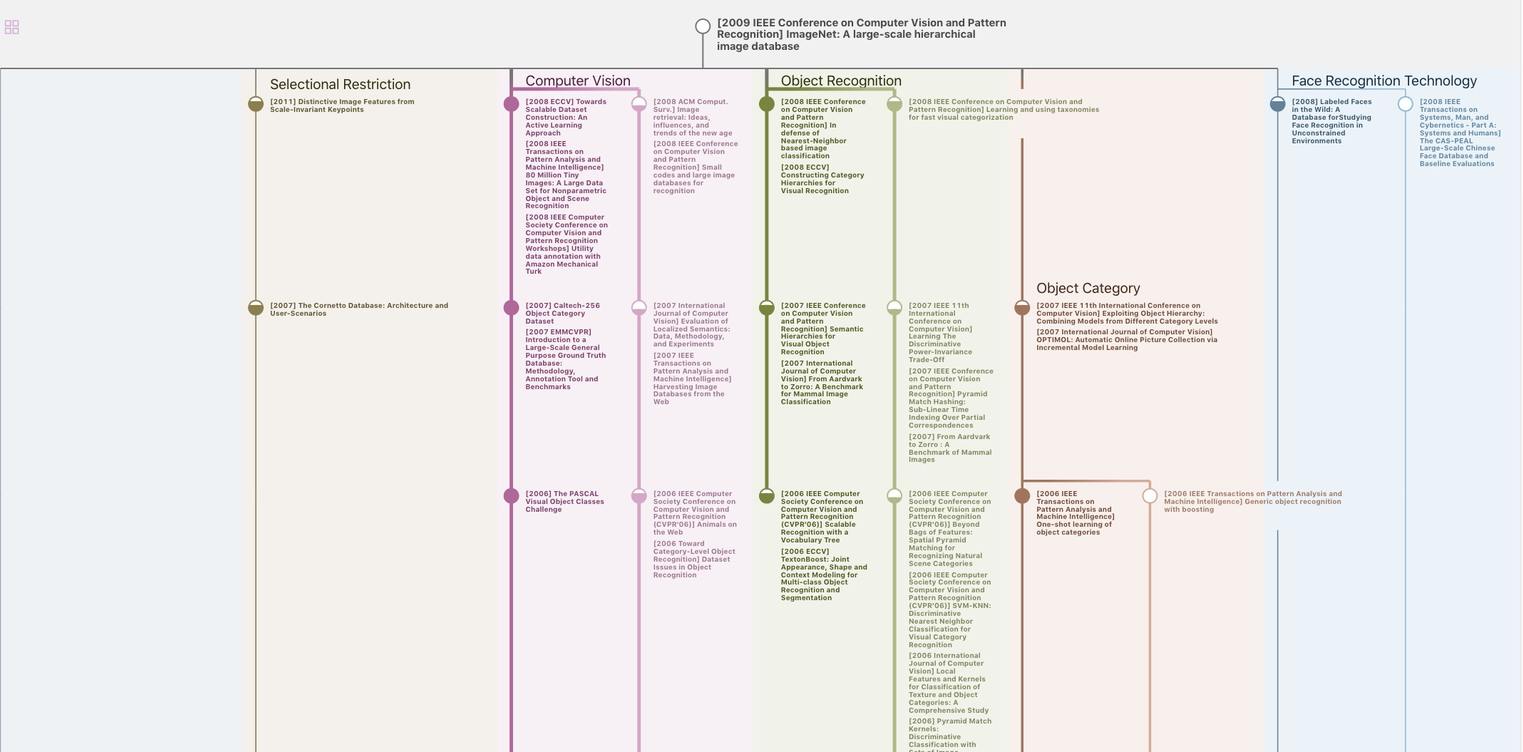
生成溯源树,研究论文发展脉络
Chat Paper
正在生成论文摘要