Overlearning Reveals Sensitive Attributes.
CoRR(2020)
摘要
``"Overlearning'' means that a model trained for a seemingly simple
objective implicitly learns to recognize attributes and concepts that are
(1) not part of the learning objective, and (2) sensitive from a privacy
or bias perspective. For example, a binary gender classifier of facial
images also learns to recognize races, even races that are
not represented in the training data, and identities.
We demonstrate overlearning in several vision and NLP models and analyze
its harmful consequences. First, inference-time representations of an
overlearned model reveal sensitive attributes of the input, breaking
privacy protections such as model partitioning. Second, an overlearned
model can be "`re-purposed'' for a different, privacy-violating task
even in the absence of the original training data.
We show that overlearning is intrinsic for some tasks and cannot be
prevented by censoring unwanted attributes. Finally, we investigate
where, when, and why overlearning happens during model training.
更多查看译文
关键词
privacy, censoring representation, transfer learning
AI 理解论文
溯源树
样例
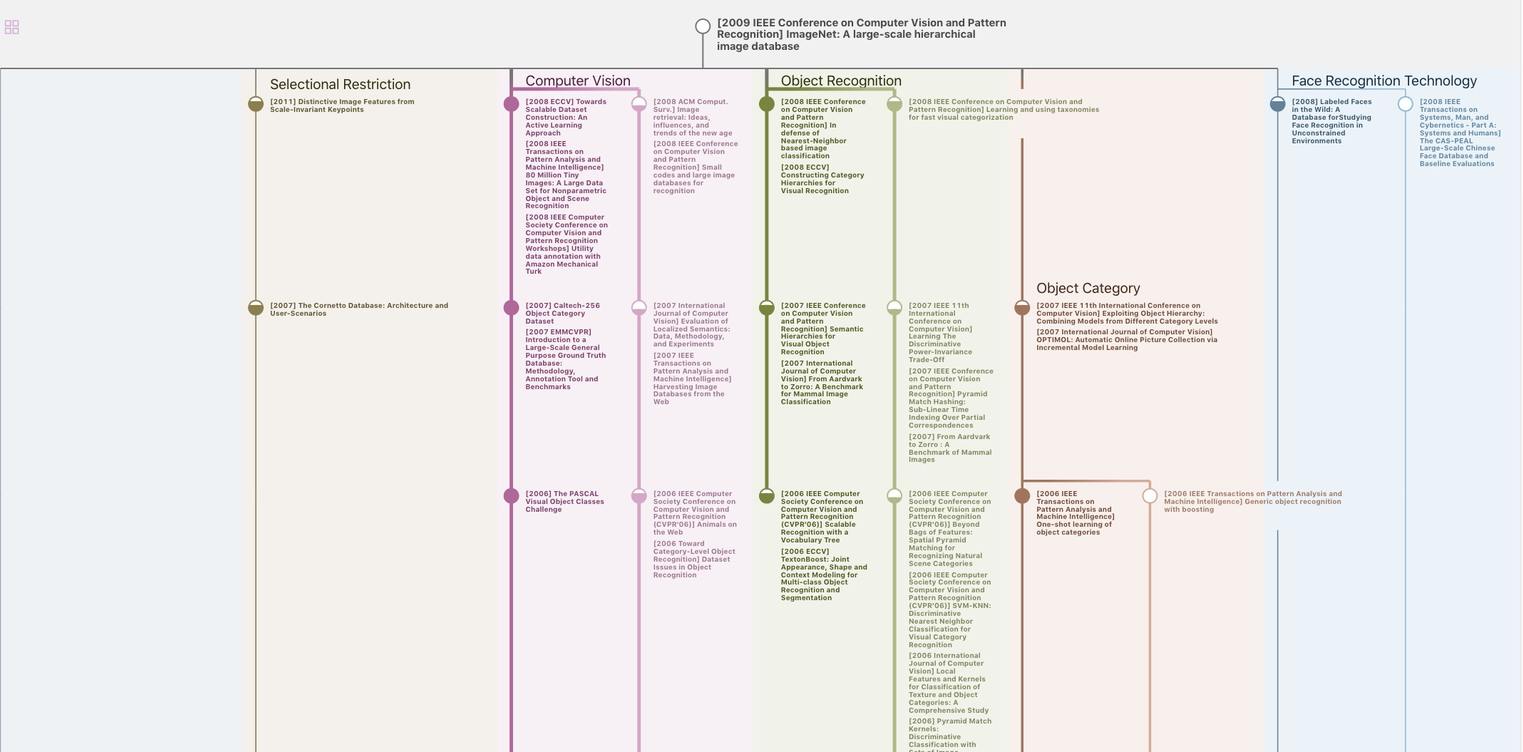
生成溯源树,研究论文发展脉络
Chat Paper
正在生成论文摘要