Marathon Environments: Multi-Agent Continuous Control Benchmarks in a Modern Video Game Engine.
arXiv: Artificial Intelligence(2019)
摘要
Recent advances in deep reinforcement learning in the paradigm of locomotion using continuous control have raised the interest of game makers for the potential of digital actors using active ragdoll. Currently, the available options to develop these ideas are either researchersu0027 limited codebase or proprietary closed systems. We present Marathon Environments, a suite of open source, continuous control benchmarks implemented on the Unity game engine, using the Unity ML- Agents Toolkit. We demonstrate through these benchmarks that continuous control research is transferable to a commercial game engine. Furthermore, we exhibit the robustness of these environments by reproducing advanced continuous control research, such as learning to walk, run and backflip from motion capture data; learning to navigate complex terrains; and by implementing a video game input control system. We show further robustness by training with alternative algorithms found in OpenAI.Baselines. Finally, we share strategies for significantly reducing the training time.
更多查看译文
关键词
modern video game engine,control,environments,multi-agent
AI 理解论文
溯源树
样例
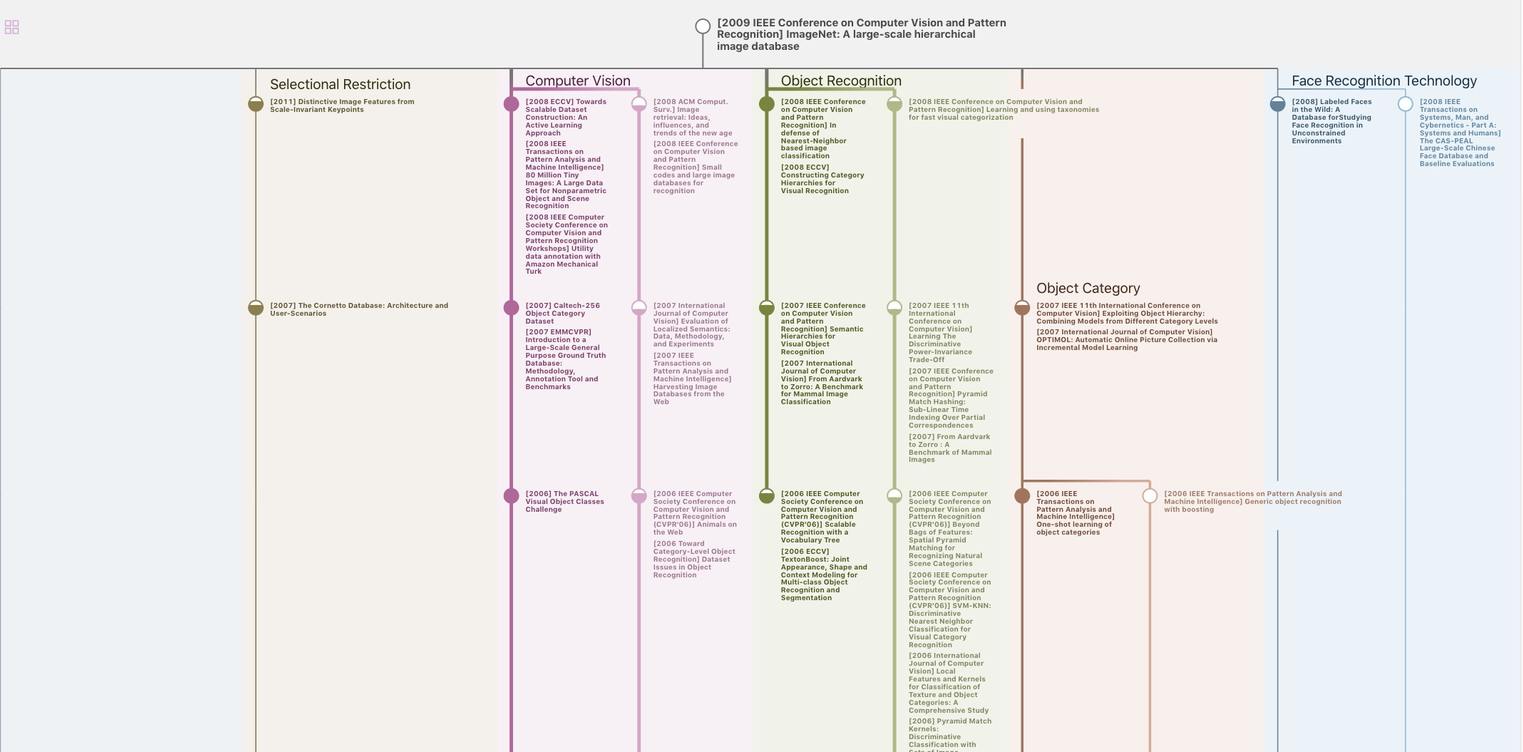
生成溯源树,研究论文发展脉络
Chat Paper
正在生成论文摘要