Structured Bayesian Compression For Deep Models In Mobile-Enabled Devices For Connected Healthcare
arXiv: Computer Vision and Pattern Recognition(2020)
摘要
Deep models, typically deep neural networks, have millions of parameters, analyze medical data accurately, yet in a time-consuming method. However, energy cost effectiveness and computational efficiency are important for prerequisites developing and deploying mobile-enabled devices, the mainstream trend in connected healthcare. Therefore, deep models' compression has become a problem of great significance for real-time health services. In this article, we first emphasize the use of Bayesian learning for model sparsity, effectively reducing the number of parameters while maintaining model performance. Specifically, with sparsity inducing priors, large parts of the network can be pruned with a simple retraining of arbitrary datasets. Then, we propose a novel structured Bayesian compression architecture by adaptively learning both group sparse and block sparse while also designing sparse-oriented mixture priors to improve the expandability of the compression model. Experimental results from both simulated datasets (MNIST) as well as practical medical datasets (Histopathologic Cancer) demonstrate the effectiveness and good performance of our framework on deep model compression.
更多查看译文
关键词
Bayes methods,Computational modeling,Medical services,Adaptation models,Computer architecture,Mixture models,Task analysis
AI 理解论文
溯源树
样例
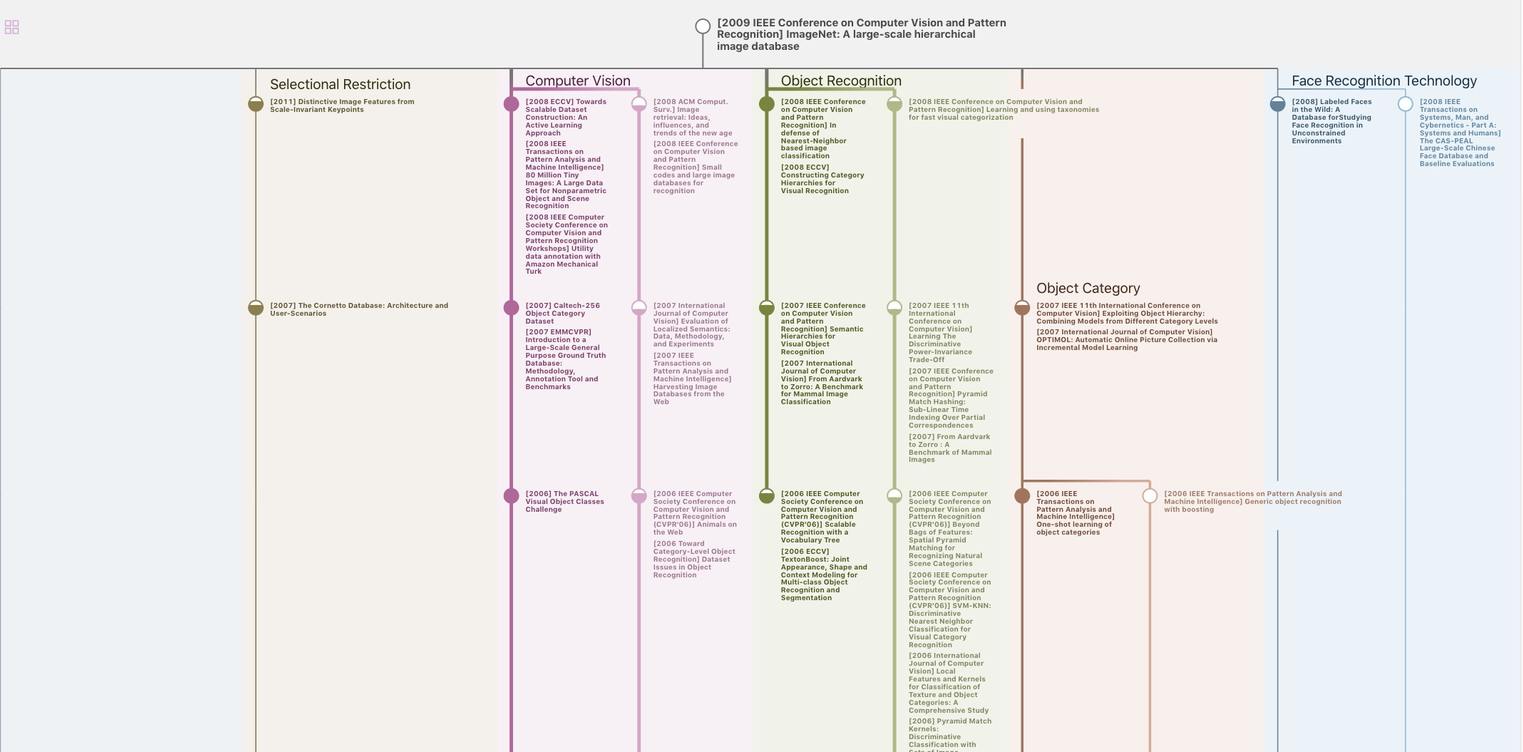
生成溯源树,研究论文发展脉络
Chat Paper
正在生成论文摘要