On Local Optimizers of Acquisition Functions in Bayesian Optimization
MACHINE LEARNING AND KNOWLEDGE DISCOVERY IN DATABASES, ECML PKDD 2020, PT II(2021)
摘要
Bayesian optimization is a sample-efficient method for finding a global optimum of an expensive-to-evaluate black-box function. A global solution is found by accumulating a pair of query point and its function value, repeating these two procedures: (i) modeling a surrogate function; (ii) maximizing an acquisition function to determine where next to query. Convergence guarantees are only valid when the global optimizer of the acquisition function is found at each round and selected as the next query point. In practice, however, local optimizers of an acquisition function are also used, since searching for the global optimizer is often a non-trivial or time-consuming task. In this paper we consider three popular acquisition functions, PI, EI, and GP-UCB induced by Gaussian process regression. Then we present a performance analysis on the behavior of local optimizers of those acquisition functions, in terms of instantaneous regrets over global optimizers. We also introduce an analysis, allowing a local optimization method to start from multiple different initial conditions. Numerical experiments confirm the validity of our theoretical analysis.
更多查看译文
关键词
Global optimization, Bayesian optimization, Acquisition function optimization, Instantaneous regret analysis
AI 理解论文
溯源树
样例
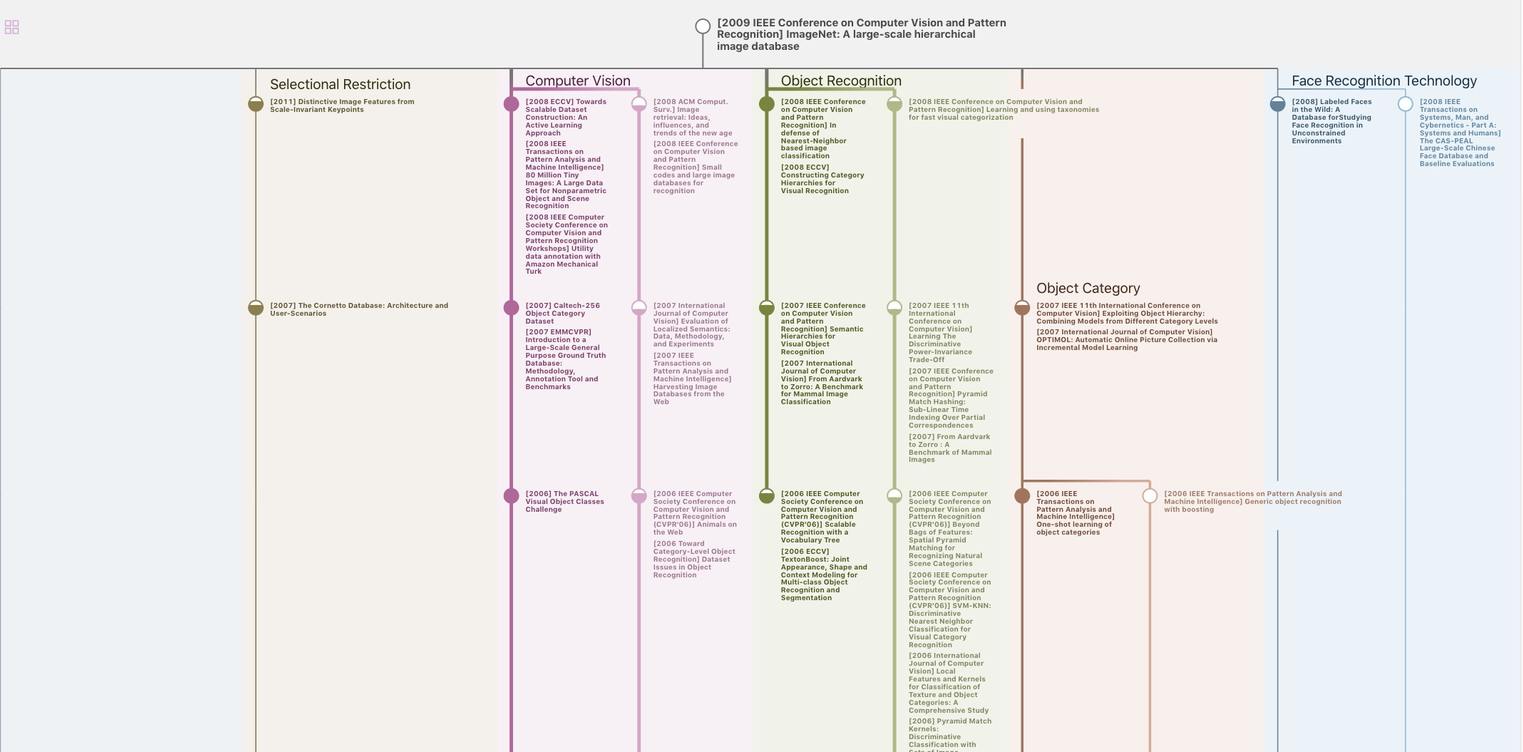
生成溯源树,研究论文发展脉络
Chat Paper
正在生成论文摘要