Simulating Data Access Profiles of Computational Jobs in Data Grids
2019 15th International Conference on eScience (eScience)(2019)
摘要
The data access patterns of applications running in computing grids are changing due to the recent proliferation of high-speed local and wide area networks. The data-intensive jobs are no longer strictly required to run at the computing sites, where the respective input data are located. Instead, jobs may access the data employing arbitrary combinations of data-placement, stage-in and remote data access. These data access profiles exhibit partially non-overlapping throughput bottlenecks. This fact can be exploited in order to minimize the time jobs spend waiting for input data. In this work we present a novel grid computing simulator, which puts a heavy emphasis on the various data access profiles. Its purpose is to enable reproducible performance studies on data access patterns. The fundamental assumptions underlying our simulator are justified by empirical experiments performed in the Worldwide LHC Computing Grid (WLCG) at CERN. We demonstrate how to calibrate the simulator parameters in accordance with the true system using posterior inference with likelihood-free Markov Chain Monte Carlo. Thereafter, we validate the simulator's output with respect to authentic production workloads from WLCG, demonstrating its remarkable accuracy.
更多查看译文
关键词
Grid Computing,Data Access Patterns,Network Modeling,Discrete Event Simulation,Bayesian Deep Learning,Likelihood-free Inference
AI 理解论文
溯源树
样例
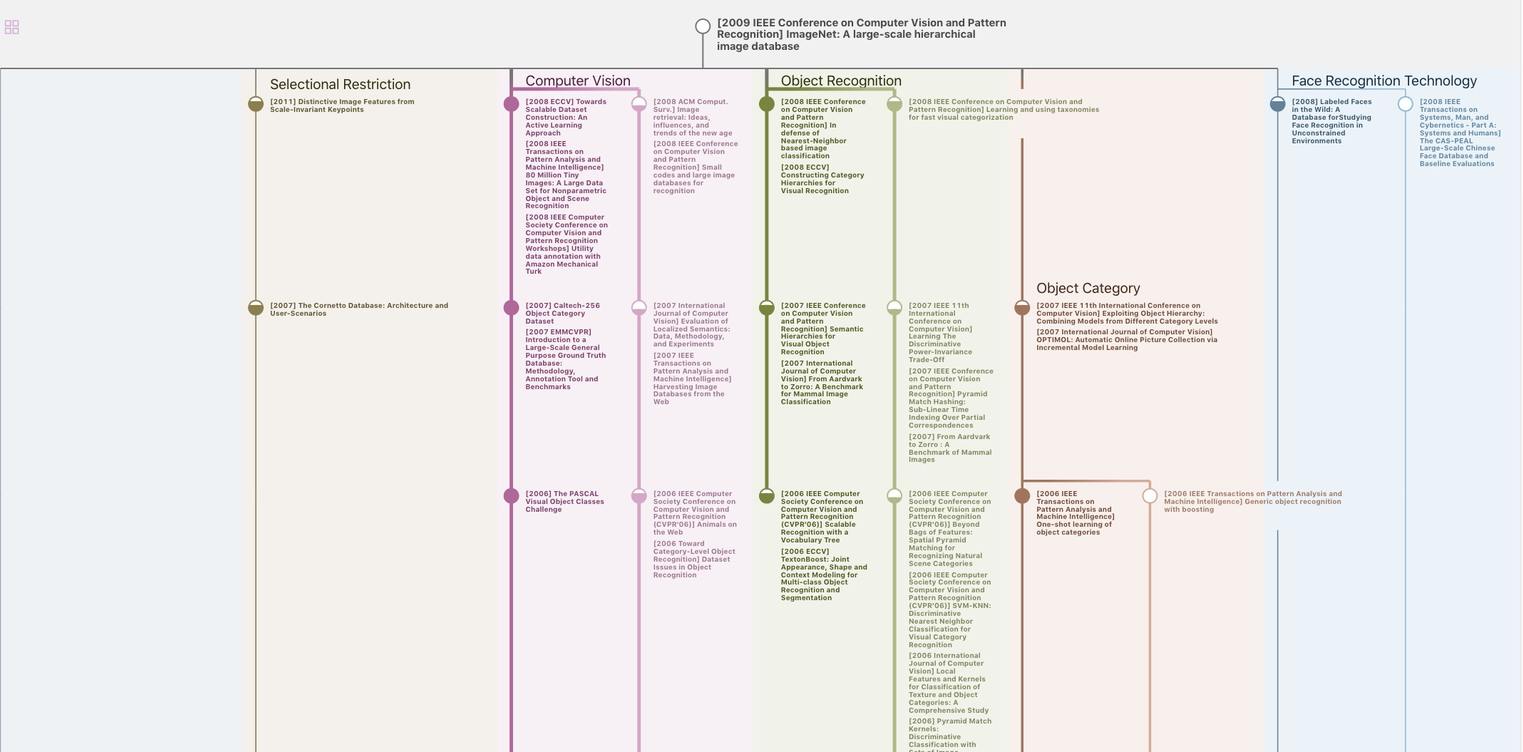
生成溯源树,研究论文发展脉络
Chat Paper
正在生成论文摘要