Unsupervised Object-Level Image-to-Image Translation Using Positional Attention Bi-Flow Generative Network.
IEEE ACCESS(2019)
摘要
Recent work in unsupervised image-to-image translation by adversarially learning mapping between different domains, which cannot distinguish the foreground and background. The existing methods of image-to-image translation mainly transfer the global image across the source and target domains. However, it is evident that not all regions of images should be transferred because forcefully transferring the unnecessary part leads to some unrealistic translations. In this paper, we present a positional attention bi-flow generative network, focusing our translation model on an interesting region or object in the image. We assume that the image representation can be decomposed into three parts: image-content, image-style, and image-position features. We apply an encoder to extract these features and bi-flow generator with attention module to achieve the translation task in an end-to-end manner. To realize the object-level translation, we adopt the image-position features to label the common interesting region between the source and target domains. We analyze the proposed framework and provide qualitative and quantitative comparisons. The extensive experiments validate that our proposed model is qualified to accomplish the object-level translation and obtain compelling results with other state-of-the-art approaches.
更多查看译文
关键词
Image-to-image translation,attention mechanism,GANs
AI 理解论文
溯源树
样例
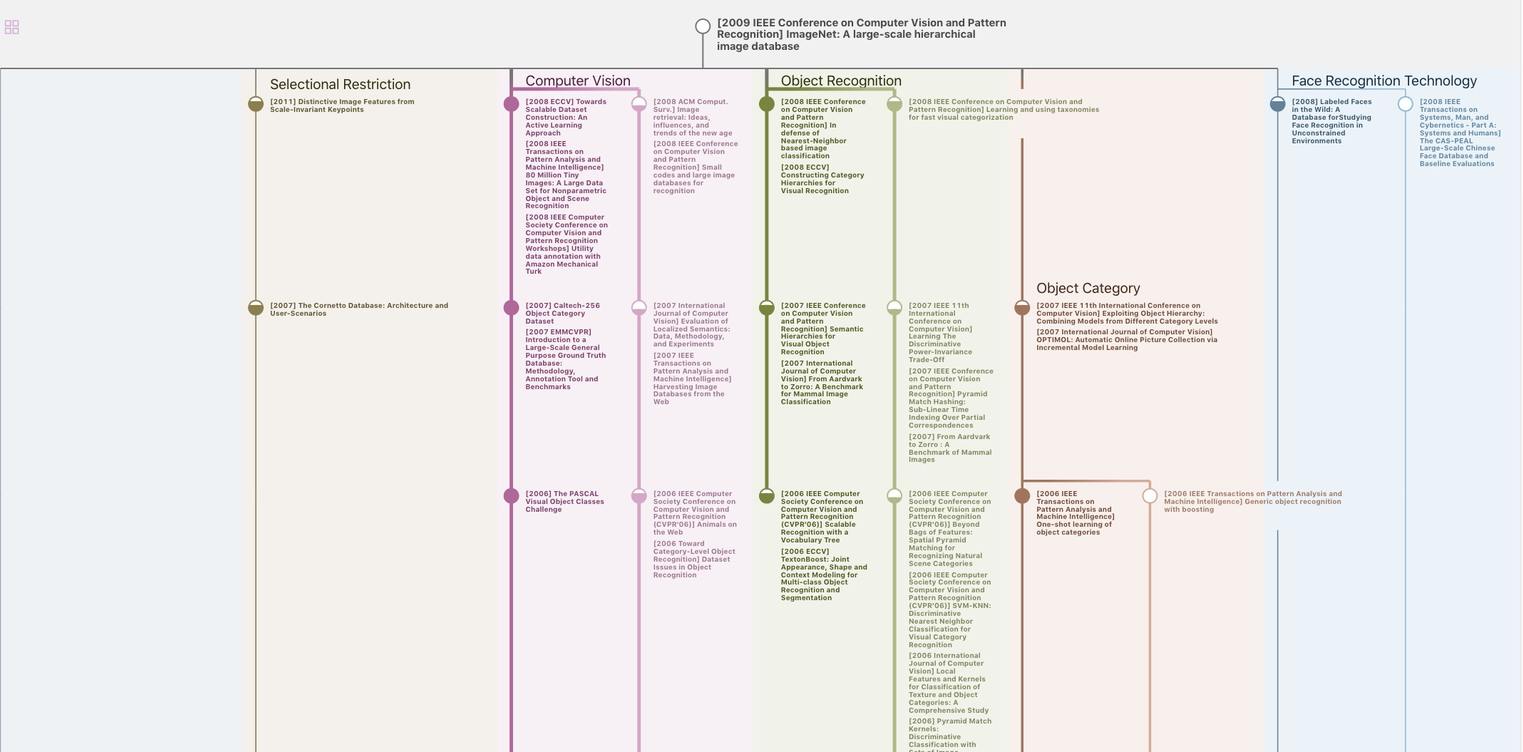
生成溯源树,研究论文发展脉络
Chat Paper
正在生成论文摘要