Approximation Hardness for A Class of Sparse Optimization Problems.
JOURNAL OF MACHINE LEARNING RESEARCH(2019)
摘要
In this paper, we consider three typical optimization problems with a convex loss function and a nonconvex sparse penalty or constraint. For the sparse penalized problem, we prove that fi nding an O (n(c1)d(c2))-optimal solution to an n x d problem is strongly NP-hard for any c(1), c(2) epsilon [0; 1) such that c(1) + c(2) < 1. For two constrained versions of the sparse optimization problem, we show that it is intractable to approximately compute a solution path associated with increasing values of some tuning parameter. The hardness results apply to a broad class of loss functions and sparse penalties. They suggest that one cannot even approximately solve these three problems in polynomial time, unless P = NP.
更多查看译文
关键词
nonconvex optimization,computational complexity,variable selection,NP-hardness,sparsity
AI 理解论文
溯源树
样例
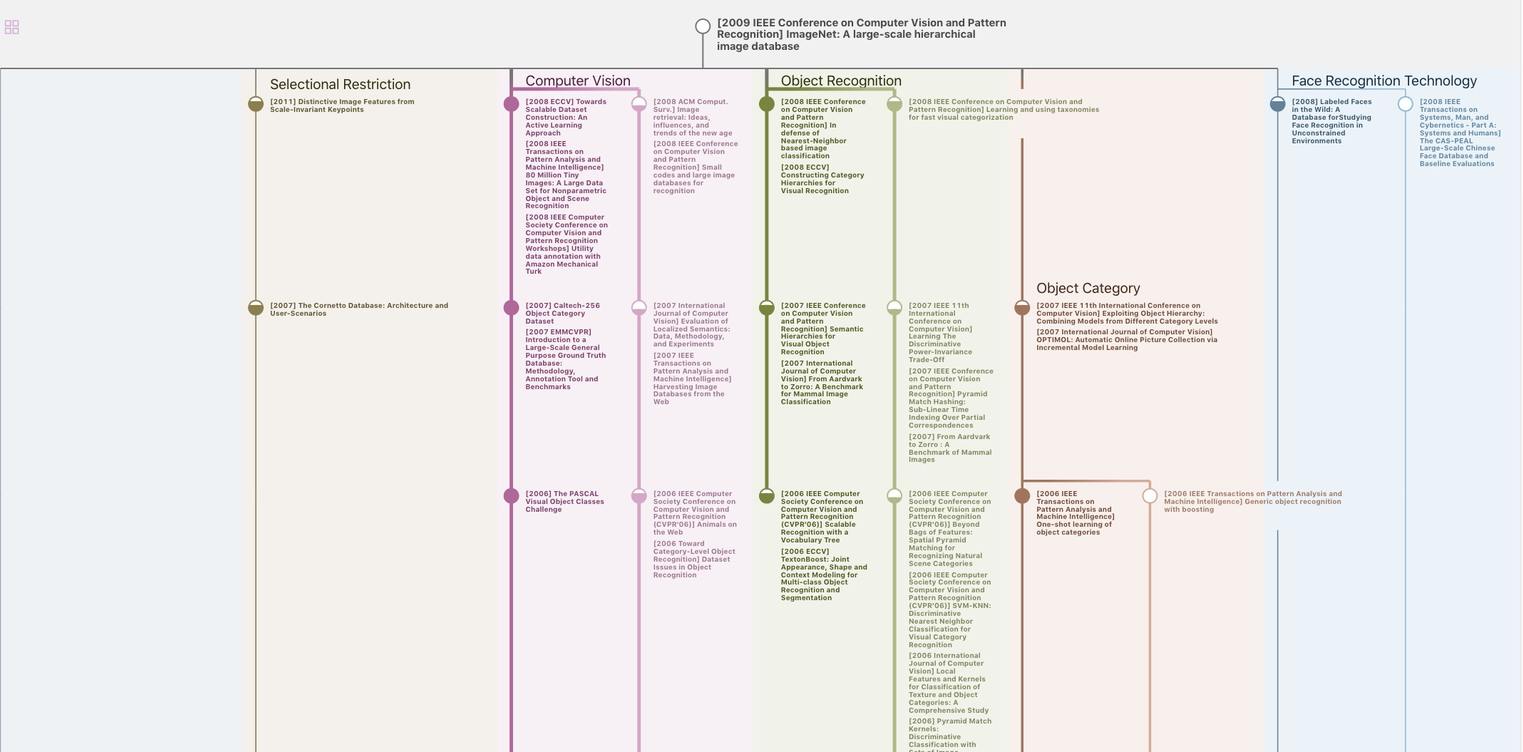
生成溯源树,研究论文发展脉络
Chat Paper
正在生成论文摘要