Sequential Gaussian Processes for Online Learning of Nonstationary Functions
CoRR(2023)
摘要
Many machine learning problems can be framed in the context of estimating functions, and often these are time -dependent functions that are estimated in real-time as observations arrive. Gaussian processes (GPs) are an attractive choice for modeling real-valued nonlinear functions due to their flexibility and uncertainty quantification. However, the typical GP regression model suffers from several drawbacks: 1) Conventional GP inference scales O(N3) with respect to the number of observations; 2) Updating a GP model sequentially is not trivial; and 3) Covariance kernels typically enforce stationarity constraints on the function, while GPs with non-stationary covariance kernels are often intractable to use in practice. To overcome these issues, we propose a sequential Monte Carlo algorithm to fit infinite mixtures of GPs that capture non-stationary behavior while allowing for online, distributed inference. Our approach empirically improves performance over state-of-the-art methods for online GP estima-tion in the presence of non-stationarity in time-series data. To demonstrate the utility of our proposed online Gaussian process mixture-of-experts approach in applied settings, we show that we can sucessfully implement an optimization algorithm using online Gaussian process bandits.
更多查看译文
关键词
Gaussian processes, Kernel, Data models, Computational modeling, Adaptation models, Mathematical models, Monte Carlo methods, sequential Monte Carlo, online learning
AI 理解论文
溯源树
样例
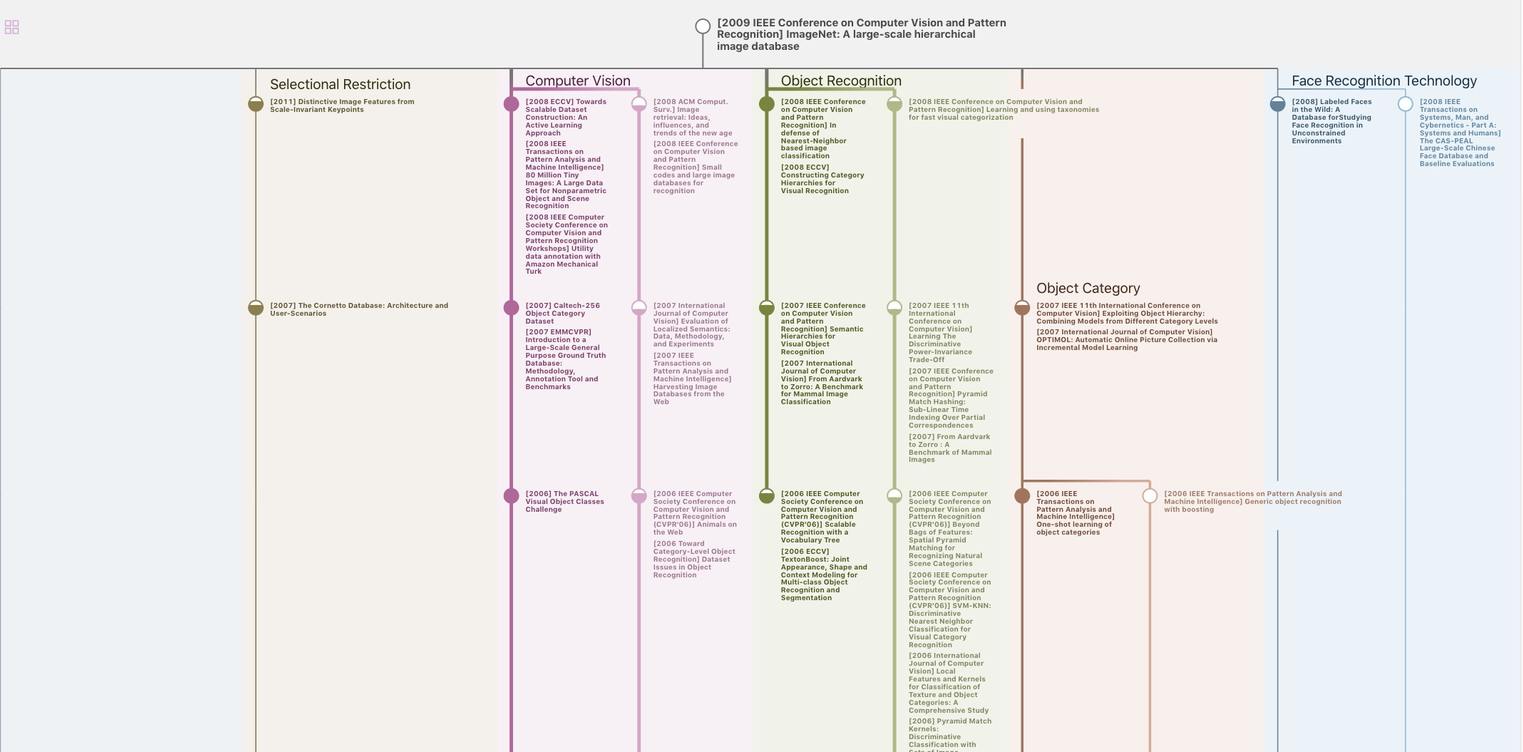
生成溯源树,研究论文发展脉络
Chat Paper
正在生成论文摘要