LithoGAN: End-to-End Lithography Modeling with Generative Adversarial Networks
Proceedings of the 56th Annual Design Automation Conference 2019(2019)
摘要
Lithography simulation is one of the most fundamental steps in process modeling and physical verification. Conventional simulation methods suffer from a tremendous computational cost for achieving high accuracy. Recently, machine learning was introduced to trade off between accuracy and runtime through speeding up the resist modeling stage of the simulation flow. In this work, we propose LithoGAN, an end-to-end lithography modeling framework based on a generative adversarial network (GAN), to map the input mask patterns directly to the output resist patterns. Our experimental results show that LithoGAN can predict resist patterns with high accuracy while achieving orders of magnitude speedup compared to conventional lithography simulation and previous machine learning based approach.
更多查看译文
关键词
LithoGAN,generative adversarial network,fundamental steps,physical verification,conventional simulation methods,simulation flow,input mask patterns,conventional lithography simulation,computational cost,machine learning,process modeling,end-to-end lithography modeling framework,resist pattern prediction
AI 理解论文
溯源树
样例
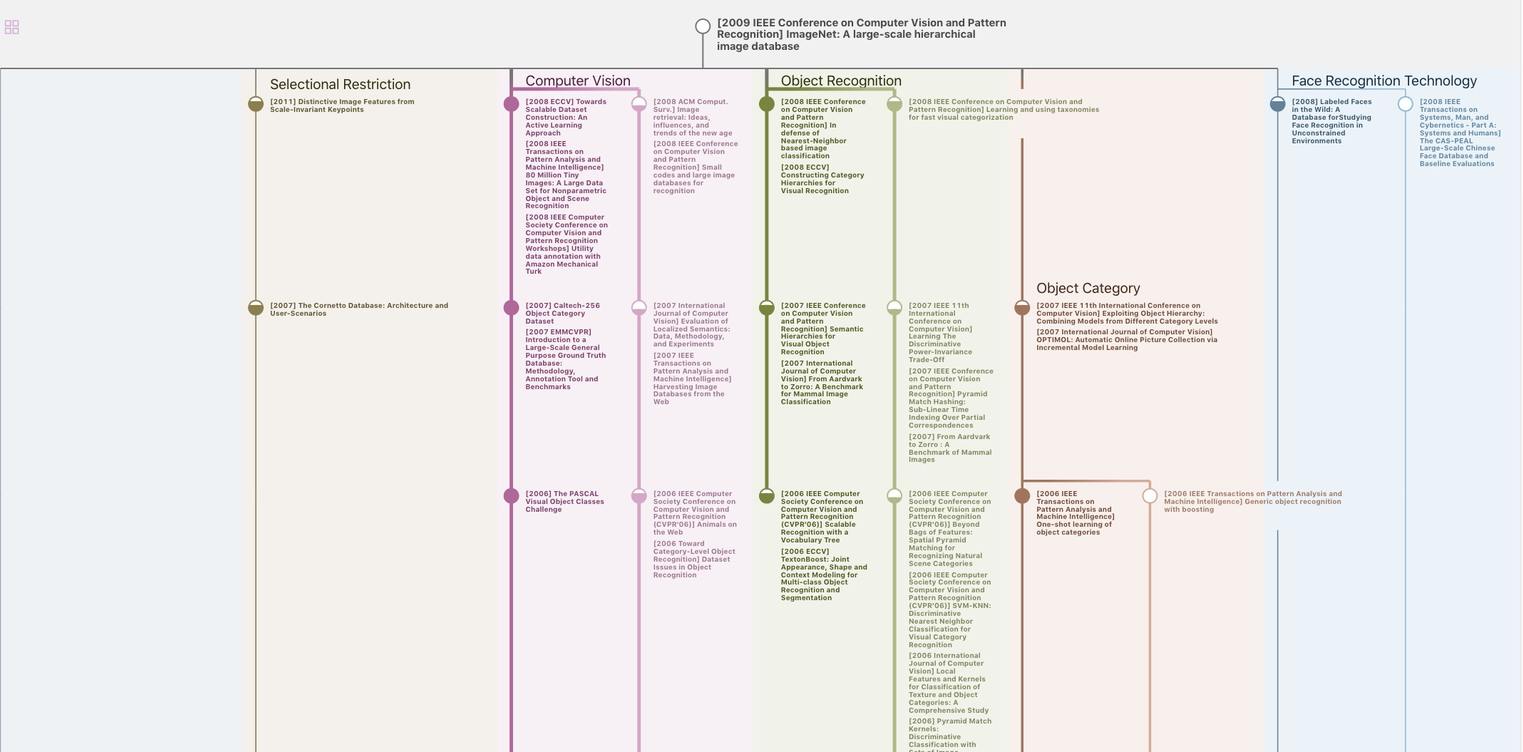
生成溯源树,研究论文发展脉络
Chat Paper
正在生成论文摘要