Robust Wireless Fingerprinting via Complex-Valued Neural Networks
2019 IEEE GLOBAL COMMUNICATIONS CONFERENCE (GLOBECOM)(2019)
摘要
A "wireless fingerprint" which exploits hardware imperfections unique to each device is a potentially powerful tool for wireless security. Such a fingerprint should be able to distinguish between devices sending the same message, and should be robust against standard spoofing techniques. Since the information in wireless signals resides in complex baseband, in this paper, we explore the use of neural networks with complex- valued weights to learn fingerprints using supervised learning. We demonstrate that, while there are potential benefits to using sections of the signal beyond just the preamble to learn fingerprints, the network cheats when it can, using information such as transmitter ID (which can be easily spoofed) to artificially inflate performance. We also show that noise augmentation by inserting additional white Gaussian noise can lead to significant performance gains, which indicates that this counter-intuitive strategy helps in learning more robust fingerprints. We provide results for two different wireless protocols, WiFi and ADS-B, demonstrating the effectiveness of the proposed method.
更多查看译文
关键词
wireless fingerprinting,complex-valued neural networks
AI 理解论文
溯源树
样例
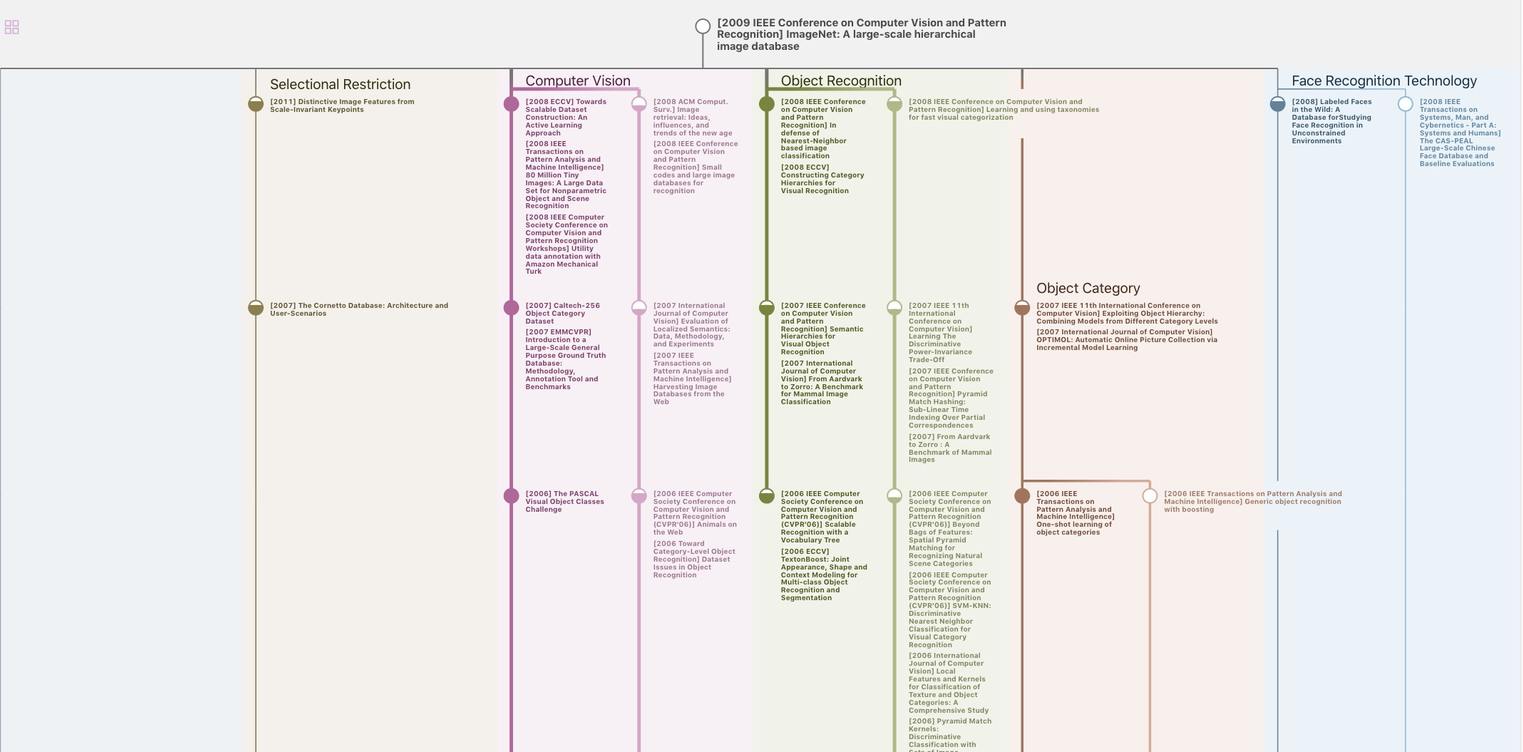
生成溯源树,研究论文发展脉络
Chat Paper
正在生成论文摘要