Fast Approximate Geodesics For Deep Generative Models
ARTIFICIAL NEURAL NETWORKS AND MACHINE LEARNING - ICANN 2019: DEEP LEARNING, PT II(2019)
摘要
The length of the geodesic between two data points along a Riemannian manifold, induced by a deep generative model, yields a principled measure of similarity. Current approaches are limited to low-dimensional latent spaces, due to the computational complexity of solving a non-convex optimisation problem. We propose finding shortest paths in a finite graph of samples from the aggregate approximate posterior, that can be solved exactly, at greatly reduced runtime, and without a notable loss in quality. Our approach, therefore, is hence applicable to high-dimensional problems, e.g., in the visual domain. We validate our approach empirically on a series of experiments using variational autoen-coders applied to image data, including the Chair, FashionMNIST, and human movement data sets.
更多查看译文
关键词
Deep generative model, Geodesic, A* search
AI 理解论文
溯源树
样例
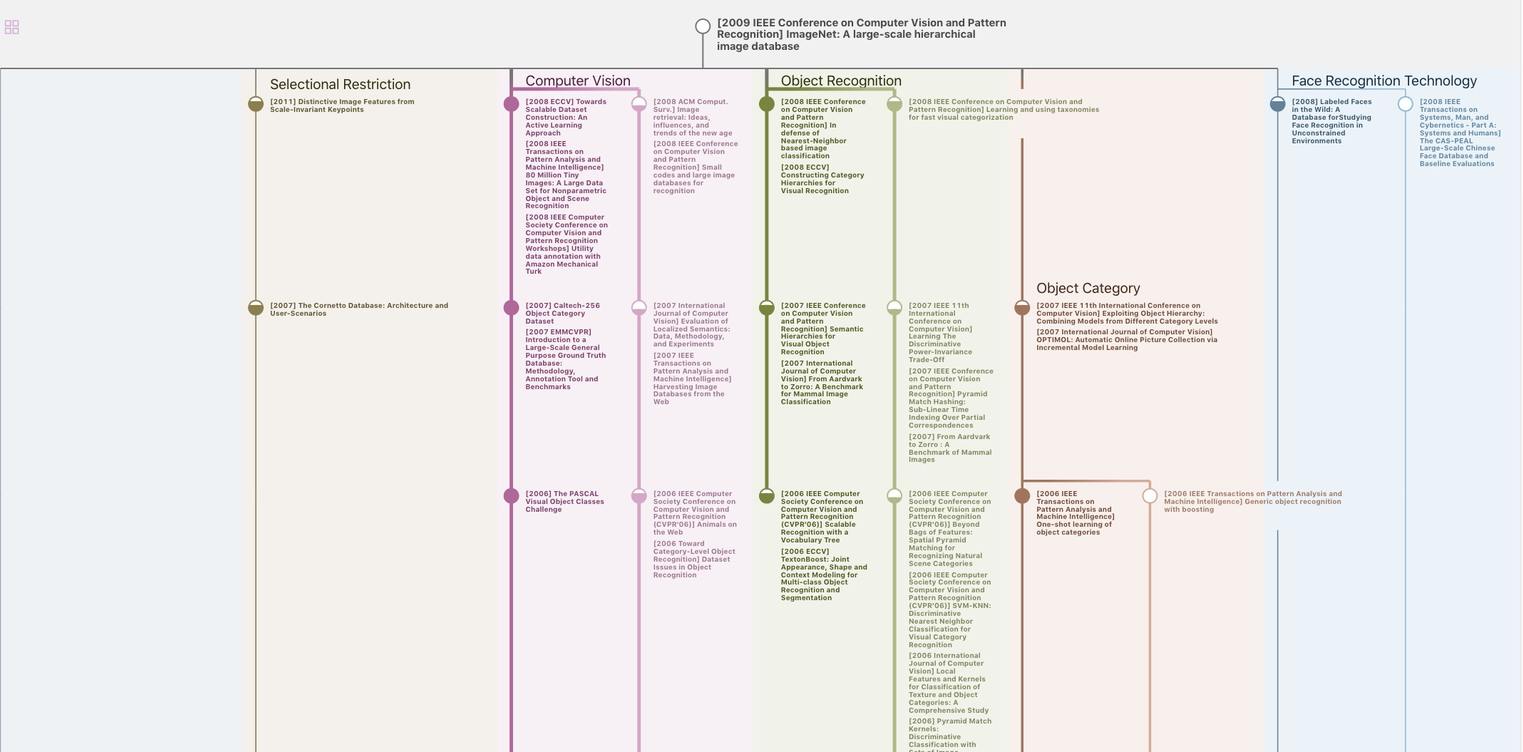
生成溯源树,研究论文发展脉络
Chat Paper
正在生成论文摘要