Blind Identification Of Stochastic Block Models From Dynamical Observations
SIAM JOURNAL ON MATHEMATICS OF DATA SCIENCE(2020)
摘要
We consider a blind identification problem in which we aim to recover a statistical model of a network without knowledge of the network's edges but based solely on nodal observations of a certain process. More concretely, we focus on observations that consist of single snapshots taken from multiple trajectories of a diffusive process that evolves over the unknown network. We model the network as generated from an independent draw from a latent stochastic block model (SBM), and our goal is to infer both the partition of the nodes into blocks and the parameters of this SBM. We discuss some nonidentifiability issues related to this problem and present simple spectral algorithms that provably solve the partition recovery and parameter estimation problems with high accuracy. Our analysis relies on recent results in random matrix theory and covariance estimation and on associated concentration inequalities. We illustrate our results with several numerical experiments.
更多查看译文
关键词
system identification, network inference, topology inference, consensus dynamics, stochastic block model, blind identification
AI 理解论文
溯源树
样例
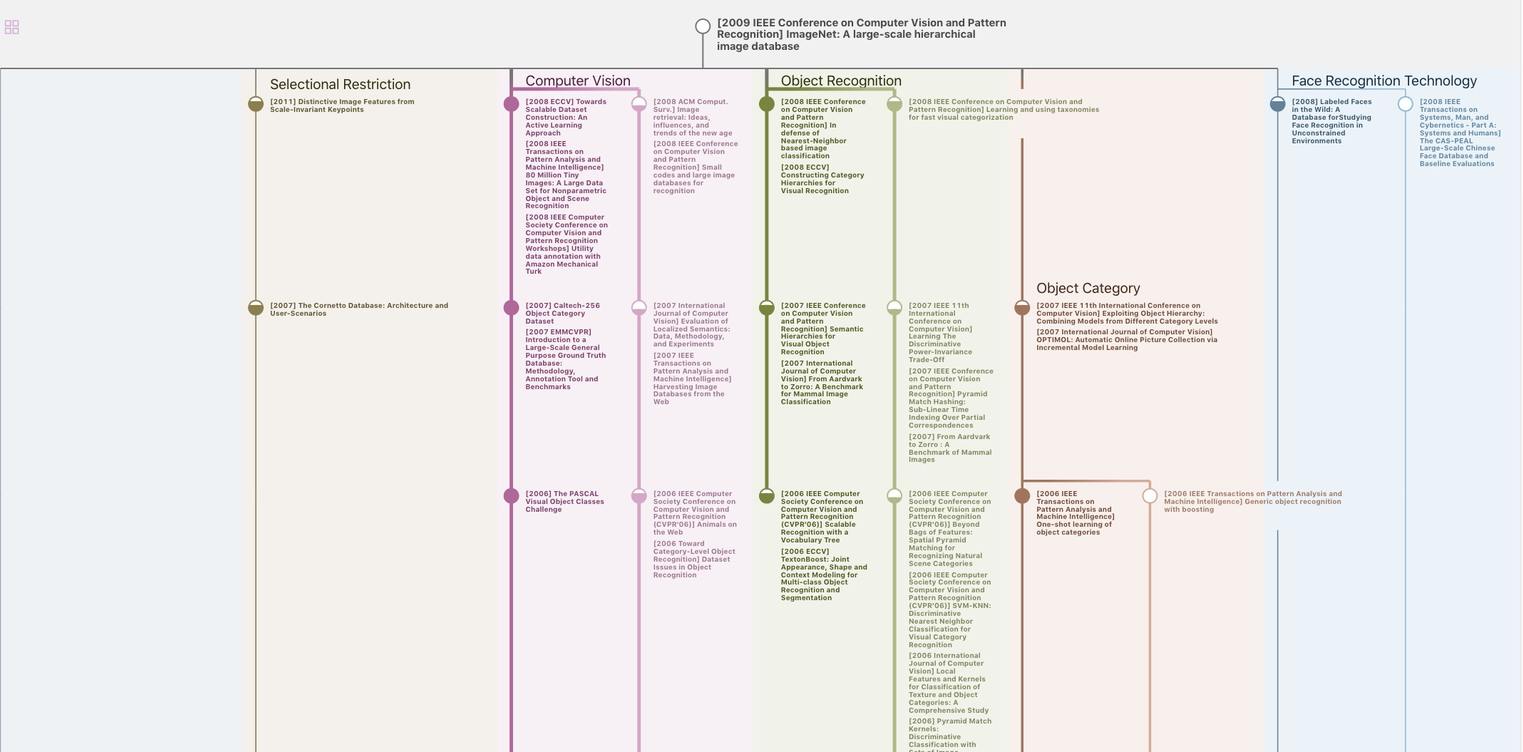
生成溯源树,研究论文发展脉络
Chat Paper
正在生成论文摘要