Modeling Complementary Products and Customer Preferences with Context Knowledge for Online Recommendation
arXiv: Information Retrieval(2019)
摘要
Modeling item complementariness and user preferences from purchase data is essential for learning good representations of products and customers, which empowers the modern personalized recommender system for Walmartu0027s e-commerce platform. The intrinsic complementary relationship among products captures the buy-also-buy patterns and provides great sources for recommendations. Product complementary patterns, though often reflected by population purchase behaviors, are not separable from customer-specific bias in purchase data. We propose a unified model with Bayesian network structure that takes account of both factors. In the meantime, we merge the contextual knowledge of both products and customers into their representations. We also use the dual product embeddings to capture the intrinsic properties of complementariness, such as asymmetry. The separating hyperplane theory sheds light on the geometric interpretation of using the additional embedding. We conduct extensive evaluations on our model before final production, and propose a novel ranking criterion based on product and customer embeddings. Our method compares favorably to existing approaches in various offline and online testings, and case studies demonstrate the advantage and usefulness of the dual product embeddings as well as the user embeddings.
更多查看译文
AI 理解论文
溯源树
样例
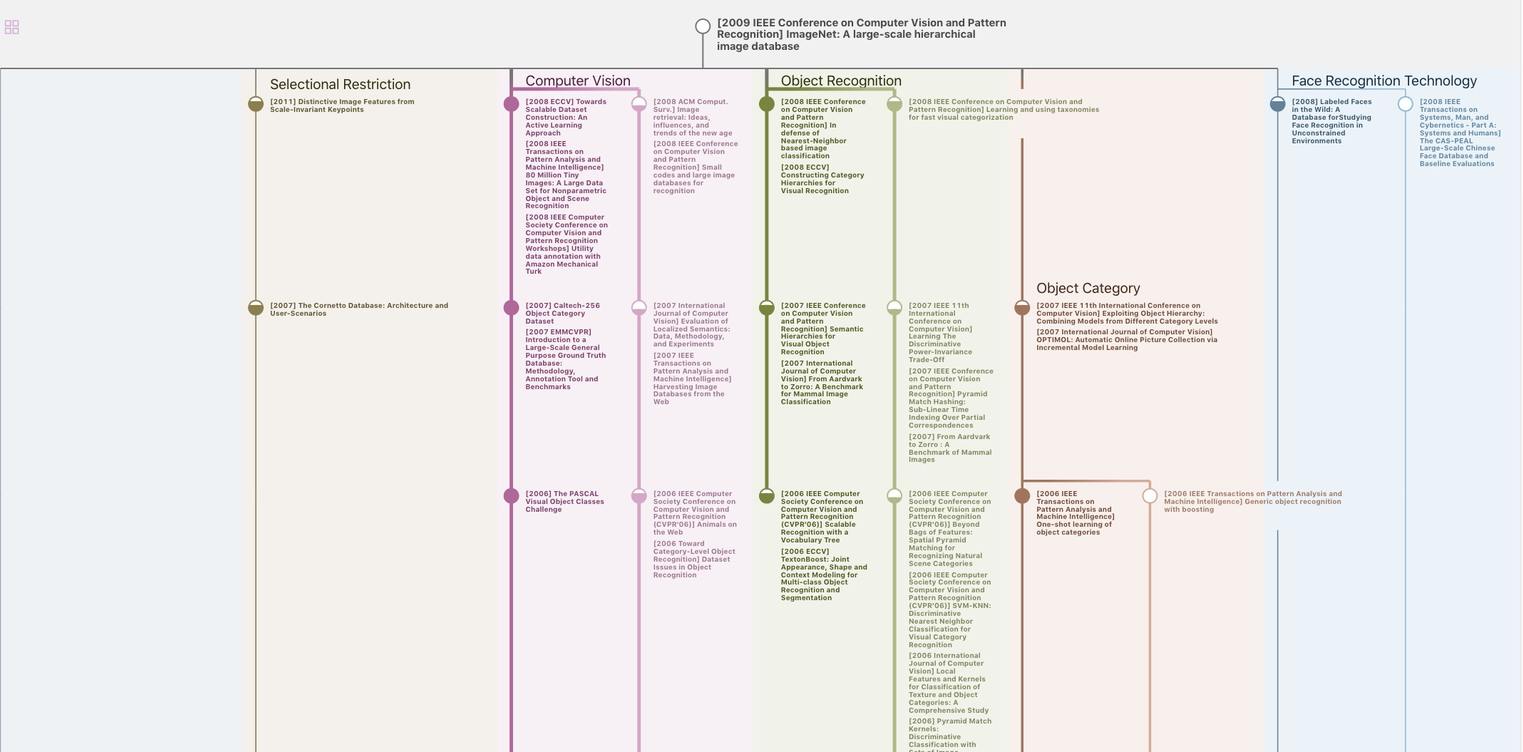
生成溯源树,研究论文发展脉络
Chat Paper
正在生成论文摘要