Image Denoising via Multi-Scale Gated Fusion Network
IEEE ACCESS(2019)
摘要
Deep convolutional neural networks have made significant progress in image denoising. However, in most cases, denoising methods using a single-stream structure with a single kernel size do not perform so well in integrating complementary contextual information; owing to the lack of this type of information, they may fail to reconstruct fine textures and patterns. To address this problem, we propose a multi-scale gated fusion network (MGFN) for image denoising, which learns direct end-to-end mappings from corrupted images to clean images. Our proposed network consists of several multi-scale mutuallygated (MM) blocks. In each MM block, we incorporate dilated convolution into a merge-and-run (MR) module to exploit multi-scale features in an effective way and further recognize useful features by filtration via a gating mechanism. Moreover, we propose a simple but effective loss function named dropout-loss to train the network. The extensive experiments on benchmark datasets show that our proposed method can well recover textures, yielding favorable performance against other state-of-the-art methods.
更多查看译文
关键词
Image denoising,convolutional neural networks,merge-and-run,multi-scale
AI 理解论文
溯源树
样例
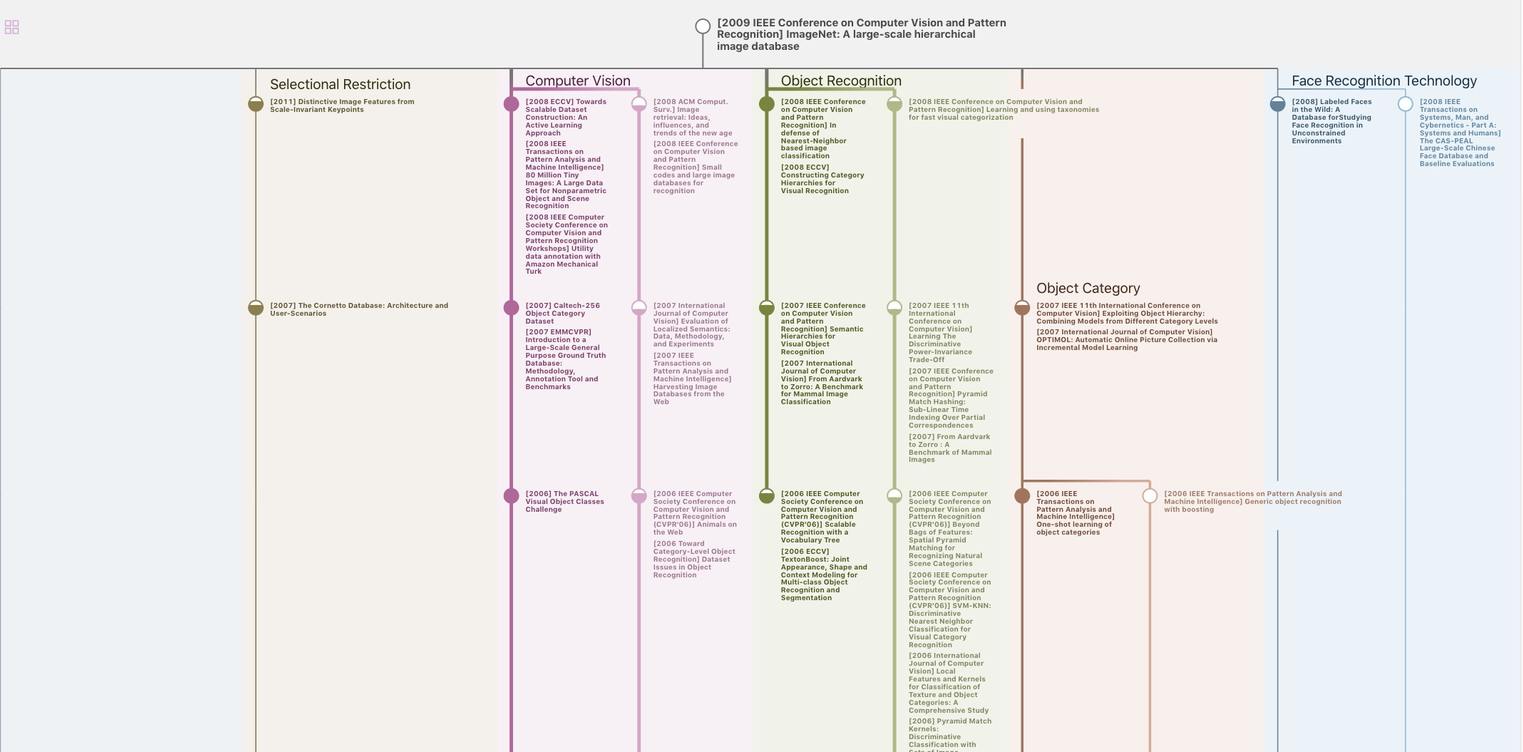
生成溯源树,研究论文发展脉络
Chat Paper
正在生成论文摘要