Transferable control for quantum parameter estimation through reinforcement learning
arxiv(2019)
摘要
Measurement and estimation of parameters are essential for science and engineering, where one of the main quests is to find systematic and robust schemes that can achieve high precision. While conventional schemes for quantum parameter estimation focus on the optimization of the probe states and measurements, it has been recently realized that control during the evolution can significantly improve the precision. The identification of optimal controls, however, is often computationally demanding, as typically the optimal controls depend on the value of the parameter which then needs to be re-calculated after the update of the estimation in each iteration. Here we show that reinforcement learning provides an efficient way to identify the controls that can be employed to improve the precision. We also demonstrate that reinforcement learning is highly transferable, namely the neural network trained under one particular value of the parameter can work for different values within a broad range. These desired features make reinforcement learning more efficient than conventional optimal quantum control methods.
更多查看译文
AI 理解论文
溯源树
样例
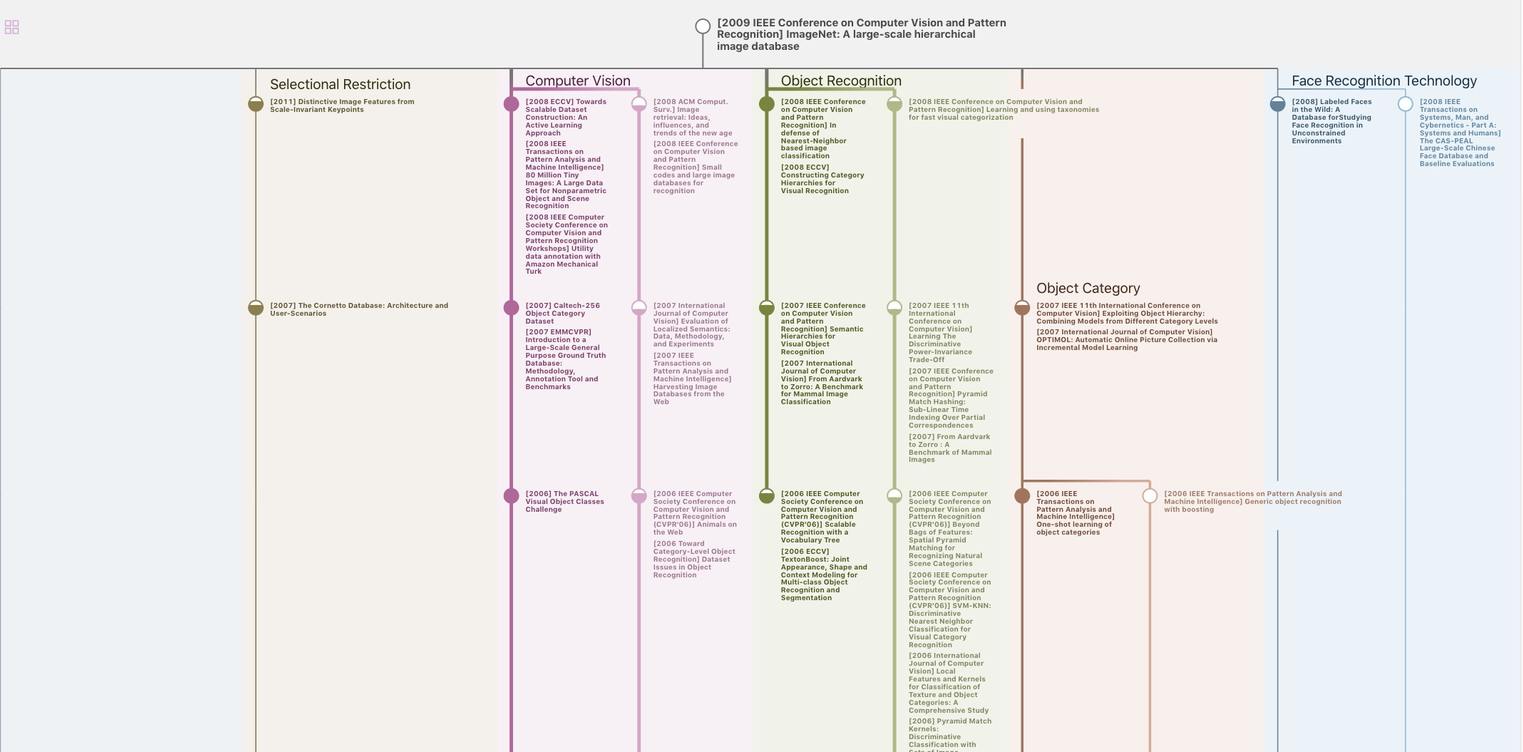
生成溯源树,研究论文发展脉络
Chat Paper
正在生成论文摘要