Learning Laplacian Matrix From Bandlimited Graph Signals
2019 IEEE INTERNATIONAL CONFERENCE ON ACOUSTICS, SPEECH AND SIGNAL PROCESSING (ICASSP)(2019)
摘要
In this paper, we present a method for learning an underlying graph topology using observed graph signals as training data. The novelty of our method lies on the combination of two assumptions that are imposed as constraints to the graph learning process: i) the standard assumption used in the literature that signals are smooth with respect to graph structure (i.e. small signal variation at adjacent nodes), with ii) the additional assumption that signals are bandlimited, which implies sparsity in the signals' representation in the spectral domain. The latter assumption affects the inference of the whole eigenvalue decomposition of the Laplacian matrix and leads to a challenging new optimization problem. The conducted experimental evaluation shows that the proposed algorithm to solve this problem outperforms a reference state-of-the-art method that is based only on the smoothness assumption, when compared in the graph learning task on synthetic and real graph signals.
更多查看译文
关键词
Graph learning, graph signal processing, signal representation, bandlimited signals, sparse coding
AI 理解论文
溯源树
样例
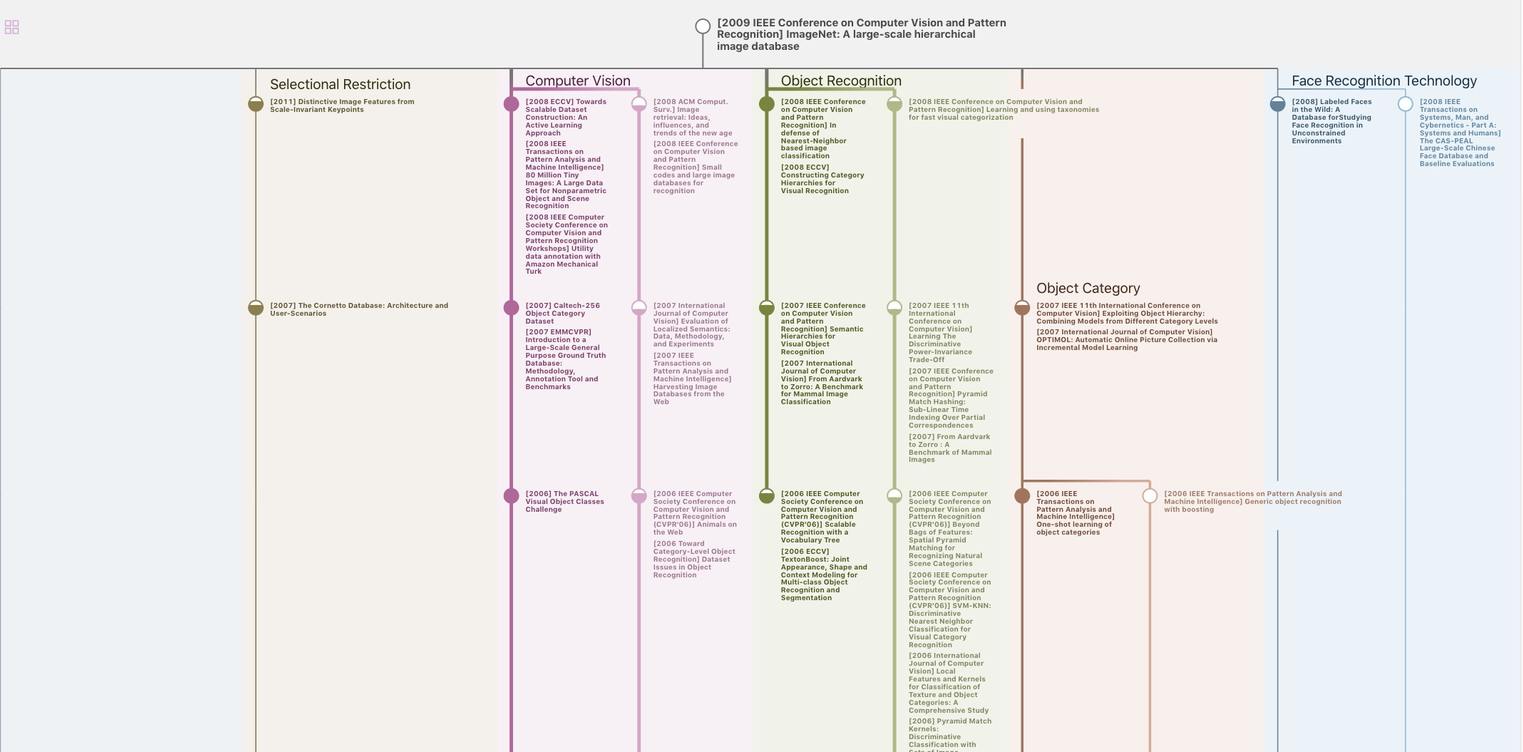
生成溯源树,研究论文发展脉络
Chat Paper
正在生成论文摘要