Non-Uniform Initialization Of Inputs Groupings In Contextual Neural Networks
INTELLIGENT INFORMATION AND DATABASE SYSTEMS, ACIIDS 2019, PT II(2019)
摘要
Contextual neural networks which are using neurons with conditional aggregation functions were found to be efficient and useful generalizations of classical multilayer perceptrons. They allow to generate neural classification models with good generalization and low activity of connections between neurons in hidden layers. The key factor to build such solutions is achieving self-consistency between continuous values of weights of neurons' connections and their mutually related non-continuous aggregation priorities. This allows to optimize neuron inputs aggregation priorities by simultaneous gradient-based optimization of connections' weights with generalized BP algorithm. But such method additionally needs initial setting of connections groupings (scan-paths) to define priorities of signals during first x epochs of training. In earlier studies all connections were initially assigned to a single group to give neurons access to all input signals at the beginning of training. We found out that such uniform solution not always is the best one. Thus within this text we compare efficiency of training of contextual neural networks with uniform and non-uniform, random initialization of connections groupings. On this basis we also discuss the properties of analyzed training algorithm which are related to characteristics of used scan-paths initialization methods.
更多查看译文
关键词
Classification, Self-consistency, Scan-paths initialization, Aggregation functions
AI 理解论文
溯源树
样例
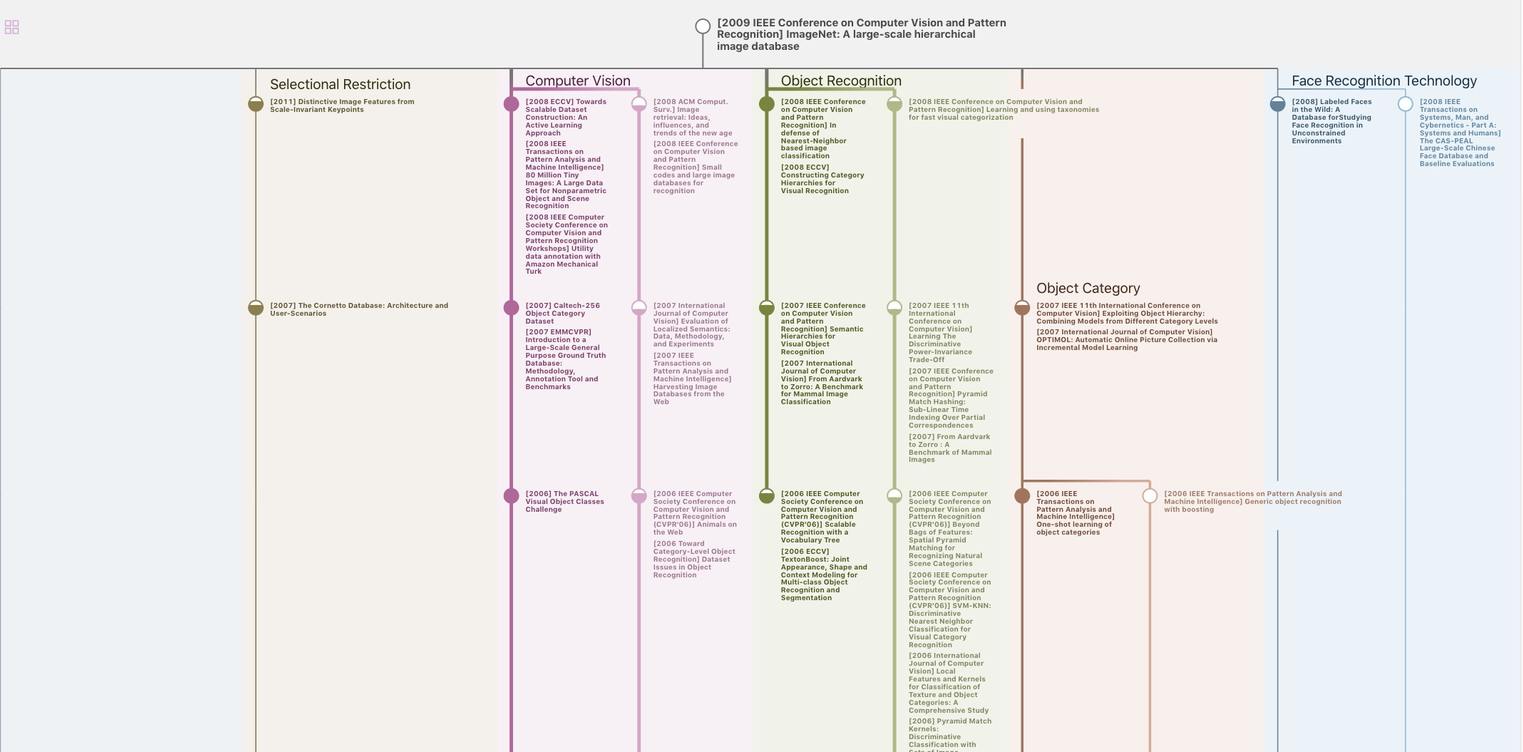
生成溯源树,研究论文发展脉络
Chat Paper
正在生成论文摘要