Computational Methods for Optimal Deployment of IoT Low Power Wide Area Networks
IEEE Internet of Things Journal(2019)
摘要
In this paper, we tackle the design issue of optimal deployment of low power wide area network(LPWAN) IoT gateways(GWs). We classify GW deployment problem into two different categories, i.e., network spatial topology (NST) aware and NST-agnostic. In NST-aware GW deployment, precise location of IoT end devices (EDs) is known and thus the design questions are: (i) where to place gateways, i.e. to maximize received signal strength; and (ii) given received signal strength which GW should the ED be associated with to balance the network load. For, NST-agnostic GW deployment, same questions are answered in the absence of precise knowledge for the locations of EDs. For the NST-aware deployment we borrow tools from machine-learning such as K-means clustering for determination of optimized GW location. Subsequently, the link assignment problem is presented as an Integer Linear Programming (ILP) optimization. We prove that the NST-agnostic GW deployment principle of placement of GWs at highest altitudes, if applied automatically, may lead to poor network performance increasing the network operational costs. Consequently, we introduce the concept of network-agnostic GW placement algorithm whereby the location of GWs can be estimated without prior knowledge of specific locations of EDs and we use it as a guiding principle to design spatial algorithm for finding GW locations. We show that spatial algorithm can, in principle, provide effective gateway placement suggestions compared to a network-aware method such as K-means clustering. We show that using a computational method for GW placement like K-means or spatial algorithm, has a potential of creating competitive network performance using just the same number of GWs, thus cutting down the financial costs of the network and increasing its sustainability.
更多查看译文
AI 理解论文
溯源树
样例
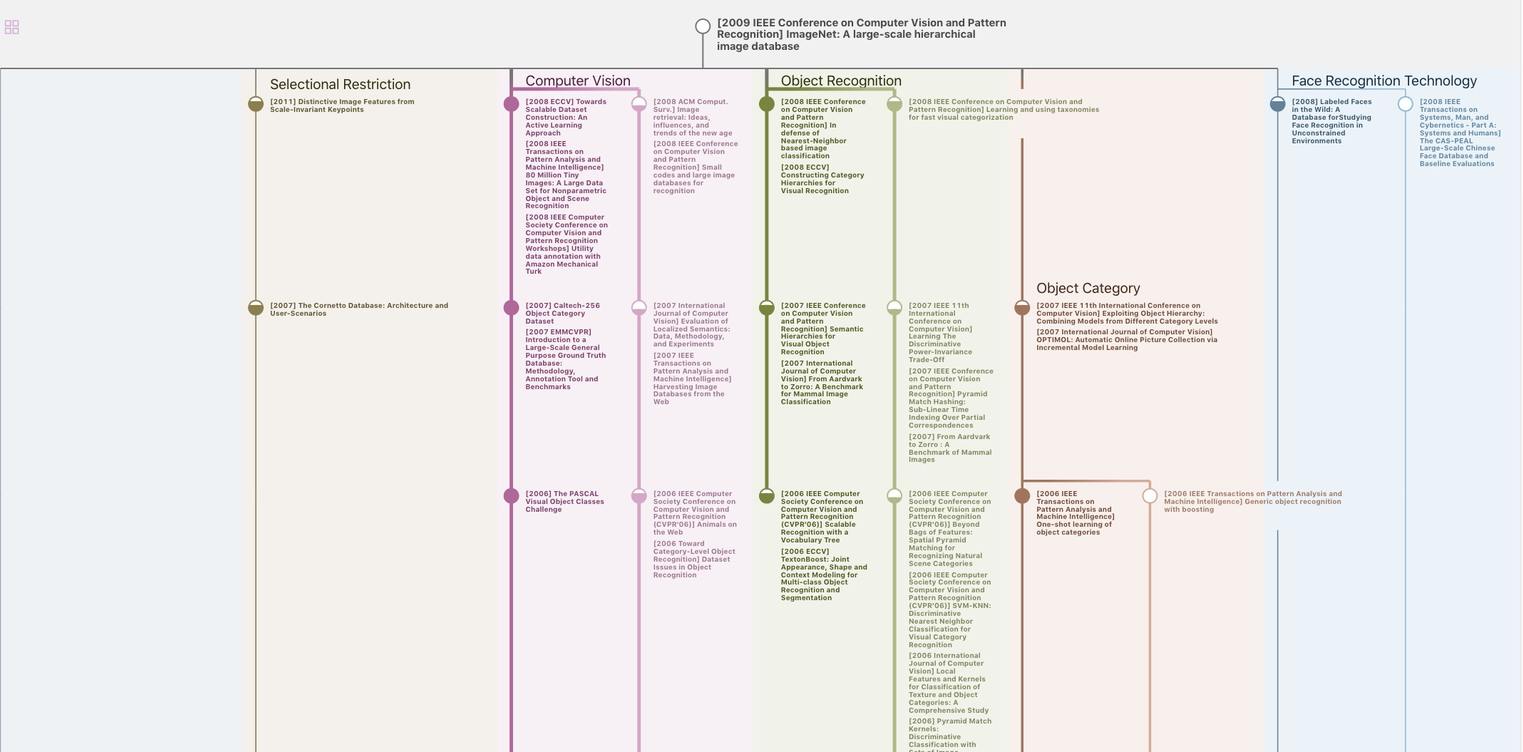
生成溯源树,研究论文发展脉络
Chat Paper
正在生成论文摘要