Relevant feature selection and ensemble classifier design using bi-objective genetic algorithm
Knowledge and Information Systems(2019)
摘要
In the era of digital boom, single classifier cannot perform well in various datasets. Ensemble classifier aims to bridge this performance gap by combining multiple classifiers of diverse characteristics to get better generalization. But classifier selection highly depends on the dataset, and its efficiency degrades tremendously due to the presence of irrelevant features. Feature selection aids the performance of classifier by removing those irrelevant features. Initially, we have proposed a bi-objective genetic algorithm-based feature selection method (FSBOGA), where nonlinear, uniform, hybrid cellular automata are used to generate an initial population. Objective functions are defined using lower bound approximation of rough set theory and Kullback–Leibler divergence method of information theory to select unambiguous and informative features. The replacement strategy for creation of next-generation population is based on the Pareto optimal solution with respect to both the objective functions. Next, a novel bi-objective genetic algorithm-based ensemble classification method (CCBOGA) is devised to ensemble the individual classifiers designed using obtained reduced datasets. It is observed that the constructed ensemble classifier performs better than the individual classifiers. The performances of proposed FSBOGA and CCBOGA are investigated on some popular datasets and compared with the state - of - the - art algorithms to demonstrate their effectiveness.
更多查看译文
关键词
Feature selection,Cellular automata,Lower bound approximation,Kullback–Leibler divergence,Bi-objective genetic algorithm,Ensemble classifier
AI 理解论文
溯源树
样例
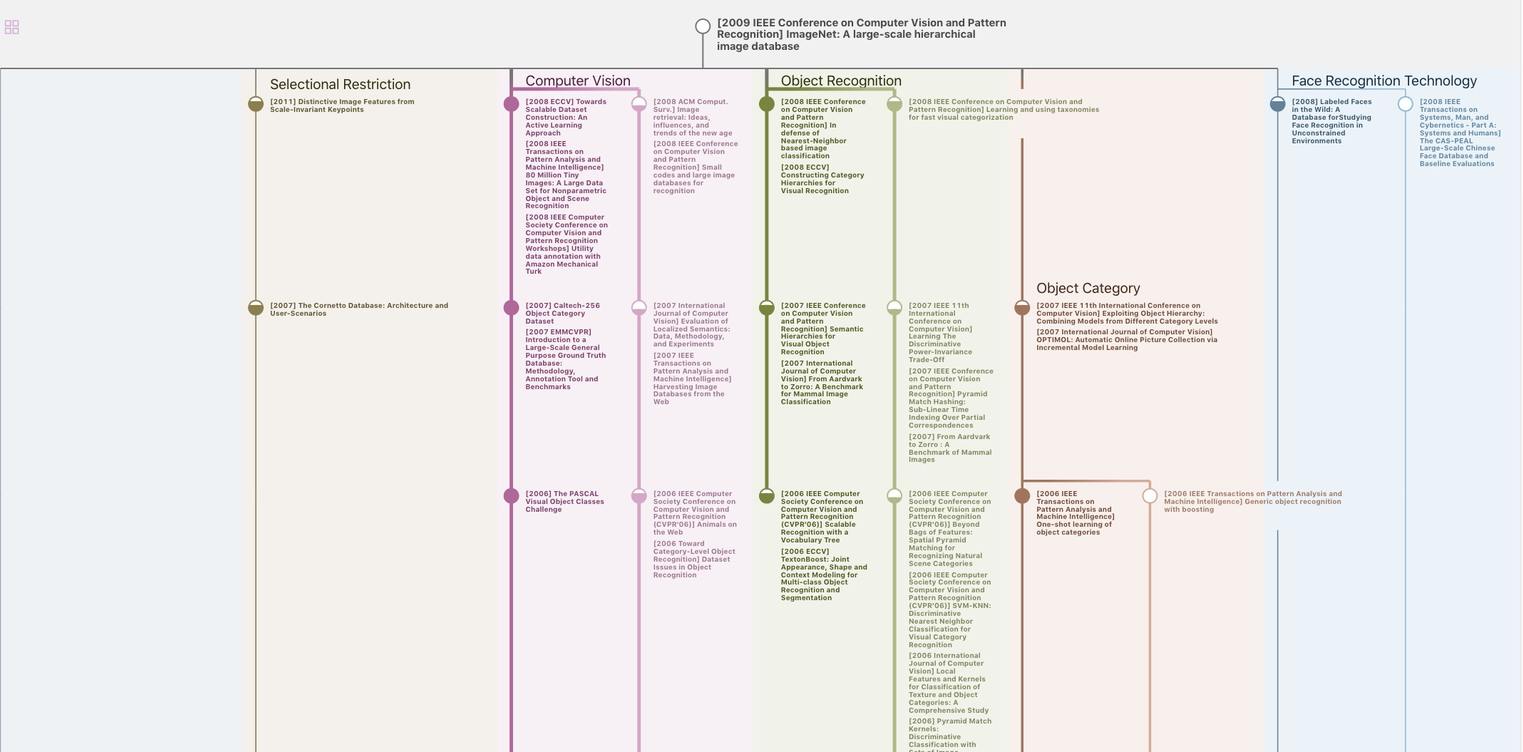
生成溯源树,研究论文发展脉络
Chat Paper
正在生成论文摘要