Detection Of Marginal Heteroscedasticity For Partial Linear Single-Index Models
COMMUNICATIONS IN STATISTICS-SIMULATION AND COMPUTATION(2021)
摘要
We consider the detection of marginal heteroscedasticity for partial linear single-index models. We regress absolute values of residuals on each covariate by marginal univariate nonparametric smoothing with a sequence of bandwidths, then a ordinary least squares estimator between the quadratic nonparametric estimate and bandwidths are obtained. We rank least squares estimates and obtain the top ranked covariates according to a ridge-type absolute coefficient ratio. Then, a refinement step is based to the smoothly clipped absolute deviation penalization method for marginal heteroscedastic detection. Simulation studies and a real dataset are conducted to demonstrate the performance of the proposed method.
更多查看译文
关键词
Marginal heteroscedasticity, Kernel smoothing, Single-index, Ridge-type absolute coefficient ratio, SCAD
AI 理解论文
溯源树
样例
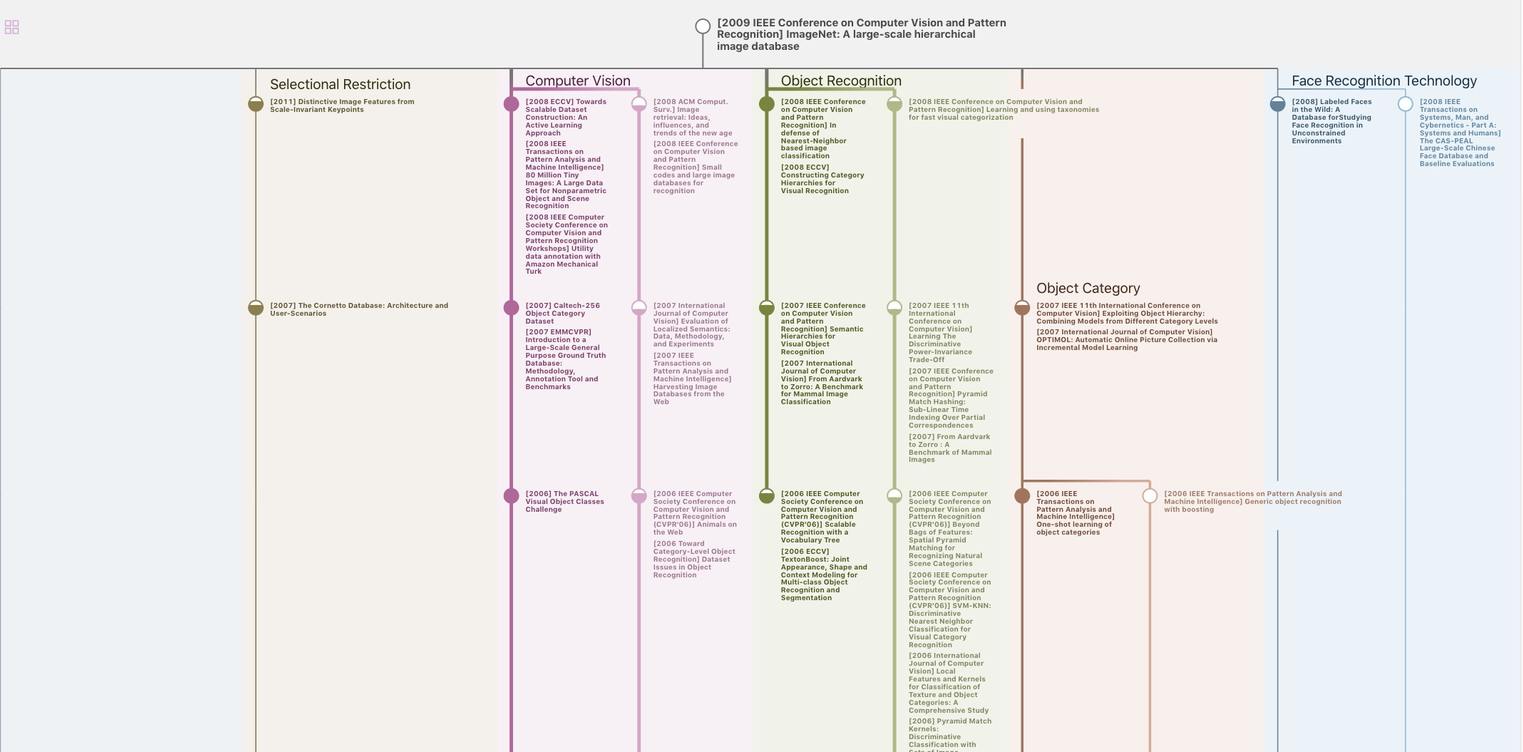
生成溯源树,研究论文发展脉络
Chat Paper
正在生成论文摘要