A Laplacian approach to $$\ell _1$$ ℓ 1 -norm minimization
arXiv: Data Structures and Algorithms(2021)
摘要
We propose a novel differentiable reformulation of the linearly-constrained
$$\ell _1$$
minimization problem, also known as the basis pursuit problem. The reformulation is inspired by the Laplacian paradigm of network theory and leads to a new family of gradient-based methods for the solution of
$$\ell _1$$
minimization problems. We analyze the iteration complexity of a natural solution approach to the reformulation, based on a multiplicative weights update scheme, as well as the iteration complexity of an accelerated gradient scheme. The results can be seen as bounds on the complexity of iteratively reweighted least squares (IRLS) type methods of basis pursuit.
更多查看译文
关键词
regression,Basis pursuit,Iteratively reweighted least squares,Multiplicative weights,Laplacian paradigm,Convex optimization
AI 理解论文
溯源树
样例
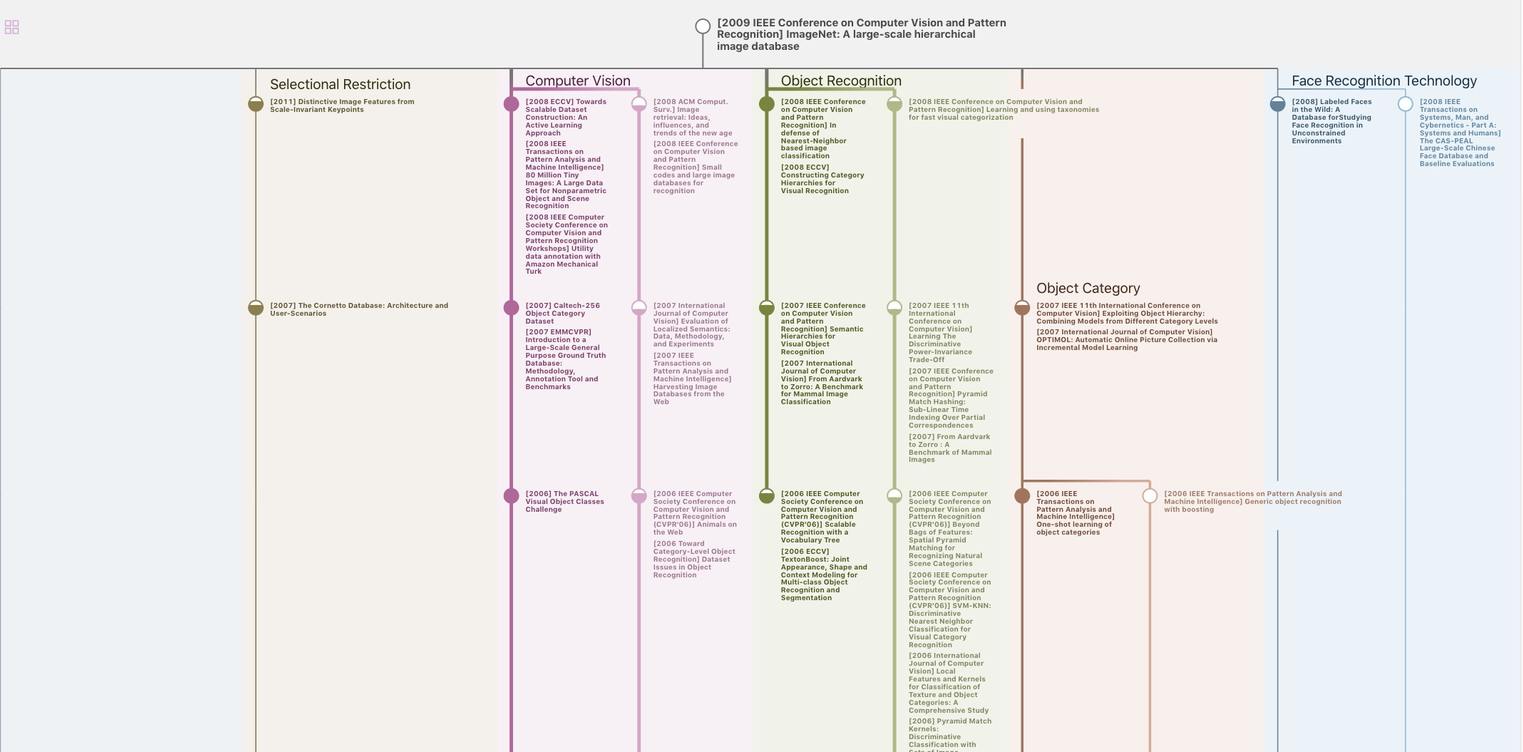
生成溯源树,研究论文发展脉络
Chat Paper
正在生成论文摘要